Detection of defects in fabrics using information set features in comparison with deep learning approaches
JOURNAL OF THE TEXTILE INSTITUTE(2022)
摘要
In this paper, a novel method based on information set theory is proposed to detect defects in fabric texture. Information set extends the fuzzy set by finding the uncertainty associated with the attribute values. By applying the information set theory on the defective fabric images, five new features are obtained. These features are Effective Information, Energy, Sigmoid, Shannon Transform and Composite Transform. These features are the result of modeling the possibilistic distribution due to variation in pixel intensities of the fabric image. These features are tested on the collected samples of fabrics from a silk cloth mill of Berhampur having four types of defects, viz., miss pick, multiple picks, oil stain and protruding yarn and the fifth type is normal. Nearest neighbor algorithm is used to classify the defects in fabrics. A comparison of the reported results with the existing features like topothesy features and deep neural network features shows that the proposed information features are better in terms of accuracy and speed.
更多查看译文
关键词
Texture defect detection, information set theory, deep learning
AI 理解论文
溯源树
样例
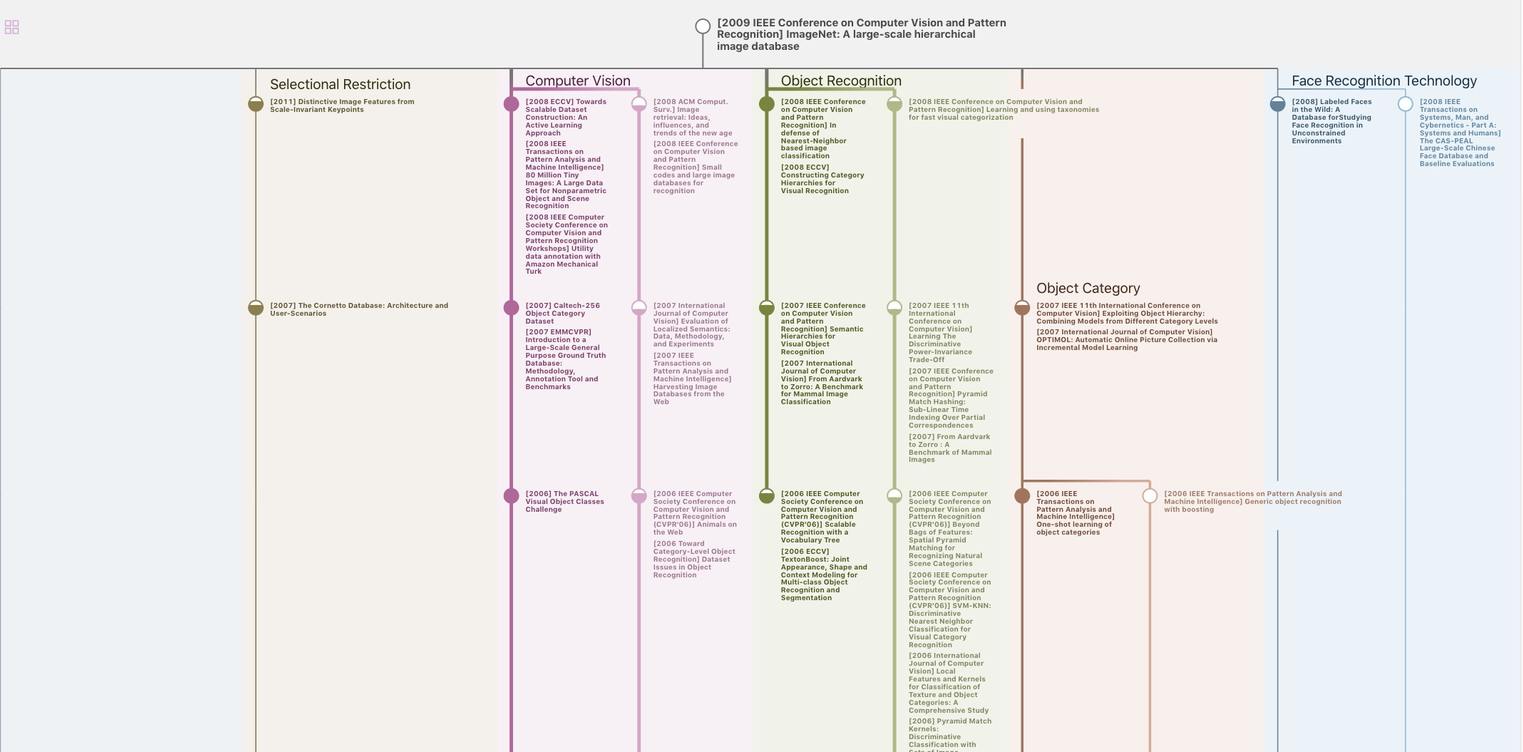
生成溯源树,研究论文发展脉络
Chat Paper
正在生成论文摘要