Bayesian prediction of potential depressions in the Erlian Basin based on integrated geophysical parameters
APPLIED GEOPHYSICS(2021)
Abstract
In this study, we analyzed the geological, gravity, magnetic, and electrical characteristics of depressions in the Erlian Basin. Based on the results of these analyses, we could identify four combined feature parameters showing strong correlations and sensibilities to the reservoir oil-bearing conditions: the average residual gravity anomaly, the average magnetic anomaly, the average depth of the conductive key layer, and the average elevation of the depressions. The feature parameters of the 65 depressions distributed in the whole basin were statistically analyzed: each of them showed a Gaussian distribution and had the basis of Bayesian theory. Our Bayesian predictions allowed the definition of a formula to calculate the posterior probability of oil occurrence in the depressions based on the combined characteristic parameters. The feasibility of this prediction method was verified by considering the results obtained for the 22 drilled depressions. Subsequently, we were able to determine the oil-bearing threshold of hydrocarbon potential for the depressions in the Erlian Basin, which can be used as a standard for quantitative optimizations. Finally, the proposed prediction method was used to calculate the probability of hydrocarbons in the other 43 depressions. Based on this probability and on the oil-bearing threshold, the five depressions with the highest potential were selected as targets for future seismic explorations and drilling. We conclude that the proposed method, which makes full use of massive gravity, magnetic, electric, and geological data, is fast, effective, and allows quantitative optimizations; hence, it will be of great value for the comprehensive geophysical evaluation of oil and gas in basins with depression group characteristics.
MoreTranslated text
Key words
Potential depressions,Bayesian prediction,feature parameters,a priori information,posterior probability
AI Read Science
Must-Reading Tree
Example
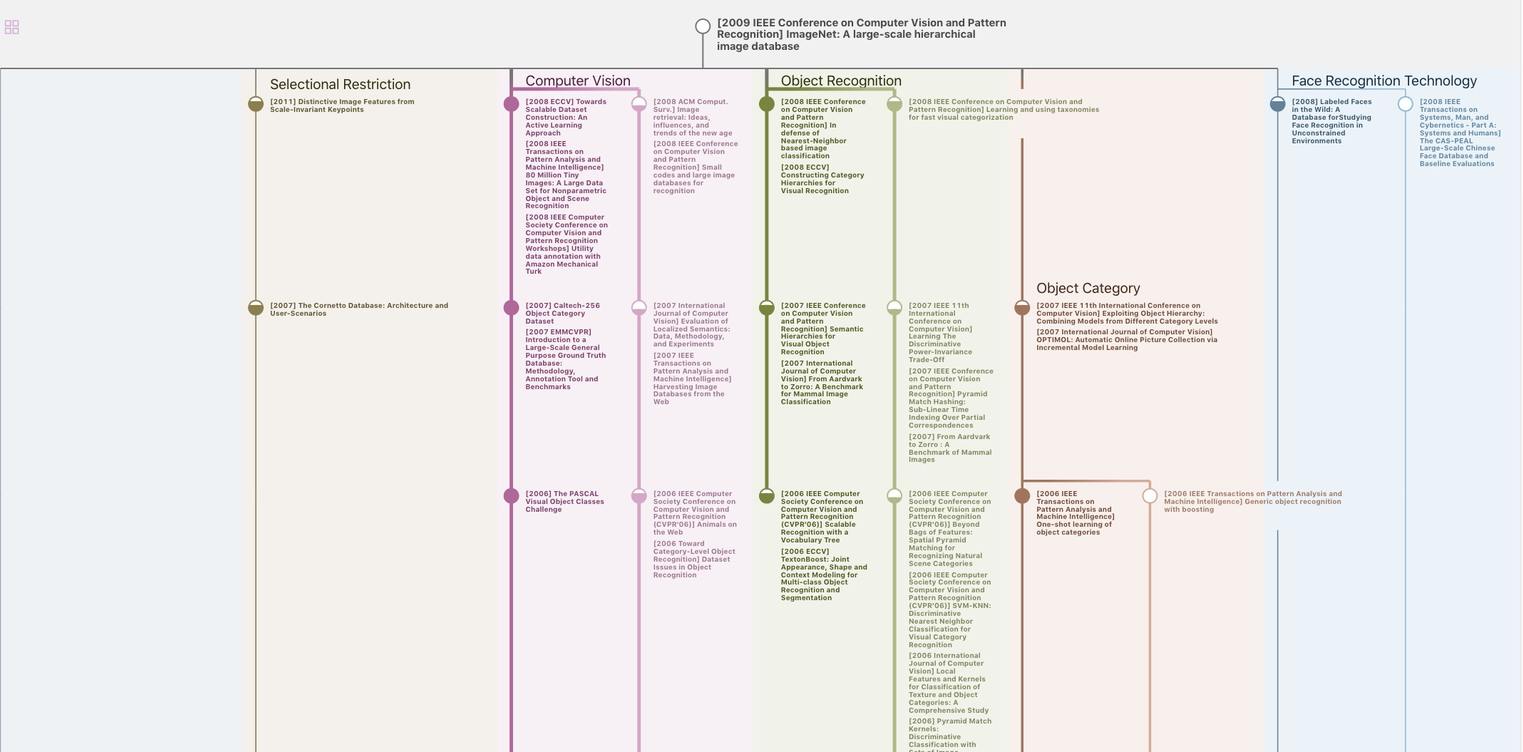
Generate MRT to find the research sequence of this paper
Chat Paper
Summary is being generated by the instructions you defined