Distributed And Multi-Task Learning At The Edge For Energy Efficient Radio Access Networks
IEEE ACCESS(2021)
摘要
The big data availability of Radio Access Network (RAN) statistics suggests using it for improving the network management through machine learning based Self Organized Network (SON) functionalities. However, this may increase the already high energy consumption of mobile networks. Multi-access Edge Computing can mitigate this problem; however, the machine learning solutions have to be properly designed for efficiently working in a distributed fashion. In this work, we propose distributed architectures for two RAN SON functionalities based on multi-task and gossip learning. We evaluate their accuracy and consumed energy in realistic scenarios. Results show that the proposed distributed implementations have the same performance but save energy with respect to their correspondent centralized versions and benchmark solutions. We conclude the paper discussing open research issues for this interesting emerging field.
更多查看译文
关键词
Training, Computational modeling, 5G mobile communication, Radio access networks, Peer-to-peer computing, Energy consumption, Edge computing, Distributed learning, edge intelligence, energy efficiency, Green AI, mobile networks
AI 理解论文
溯源树
样例
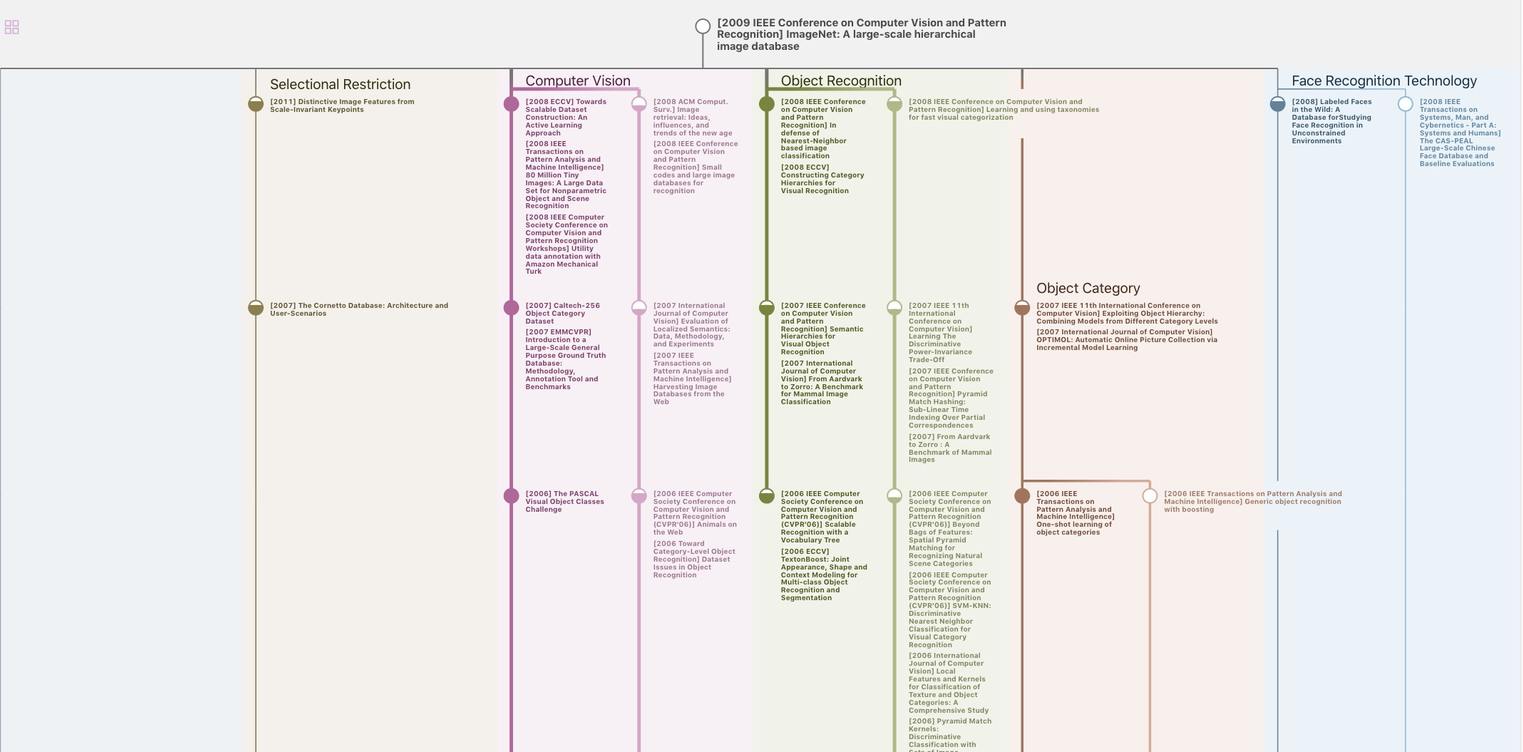
生成溯源树,研究论文发展脉络
Chat Paper
正在生成论文摘要