Adaptive Spatial Scale Person Reidentification
JOURNAL OF ELECTRONIC IMAGING(2021)
摘要
Person reidentification (ReID) requires the discriminative features of an entire pedestrian image to handle the problems of inaccurate person bounding box detection, background confusion, and occlusion. Many recent person ReID methods have attempted to learn the feature information of an entire pedestrian image through parts feature representations, but it is often too time consuming. Person ReID relies on discriminative pedestrian features, and different spatial scales can distinguish features by differing degrees. We propose an innovative and effective adaptive spatial scale person ReID network model based on the residual neural network (ResNet) of an instance batch normalization. Through experimental visualizations, pedestrian features extracted by ResNet from four layers are analyzed, and two layers with discriminative features are selected. Using an adaptive dimension adjustment module, different spatial scale features are aggregated and merged by the aggregation layer. To effectively learn spatial channel correlations and prevent overfitting, a multilayer distribution normalization processing module is designed to implement end-to-end training and evaluate the person ReID networks. Compared with other methods, this network model showed excellent performance on four public person ReID datasets and is superior to most current methods. (c) 2021 SPIE and IS&T
更多查看译文
关键词
person reidentification, adaptive spatial scale, distribution normalization
AI 理解论文
溯源树
样例
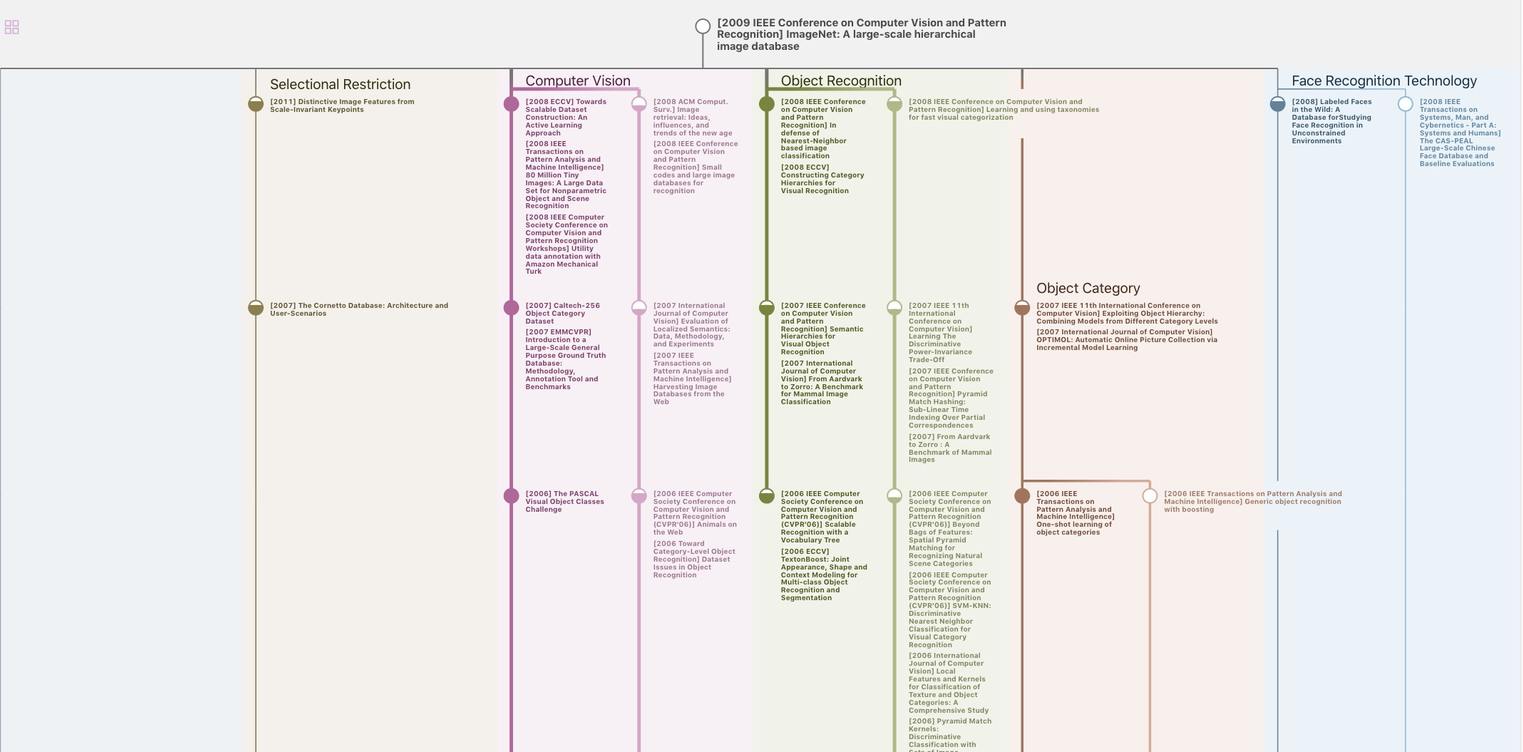
生成溯源树,研究论文发展脉络
Chat Paper
正在生成论文摘要