Oversampling Methods Combined Clustering And Data Cleaning For Imbalanced Network Data
INTELLIGENT AUTOMATION AND SOFT COMPUTING(2020)
摘要
In network anomaly detection, network traffic data are often imbalanced, that is, certain classes of network traffic data have a large sample data volume while other classes have few, resulting in reduced overall network traffic anomaly detection on a minority class of samples. For imbalanced data, researchers have proposed the use of oversampling techniques to balance data sets; in particular, an oversampling method called the SMOTE provides a simple and effective solution for balancing data sets. However, current oversampling methods suffer from the generation of noisy samples and poor information quality. Hence, this study proposes an oversampling method for imbalanced network traffic data that combines the SMOTE algorithm and FINCH clustering algorithm to filter out minority sample clusters, proposes a scheme to allocate the number of synthetic samples per cluster according to the clustering sparsity and sample weight, and finally uses multi-layer sensors for noisy sample cleaning during sampling. We compare the proposed method with other oversampling methods, verifying that a data set processed using this method works better in network traffic anomaly detection.
更多查看译文
关键词
Imbalanced learning, oversampling, SMOTE, network anomaly detection
AI 理解论文
溯源树
样例
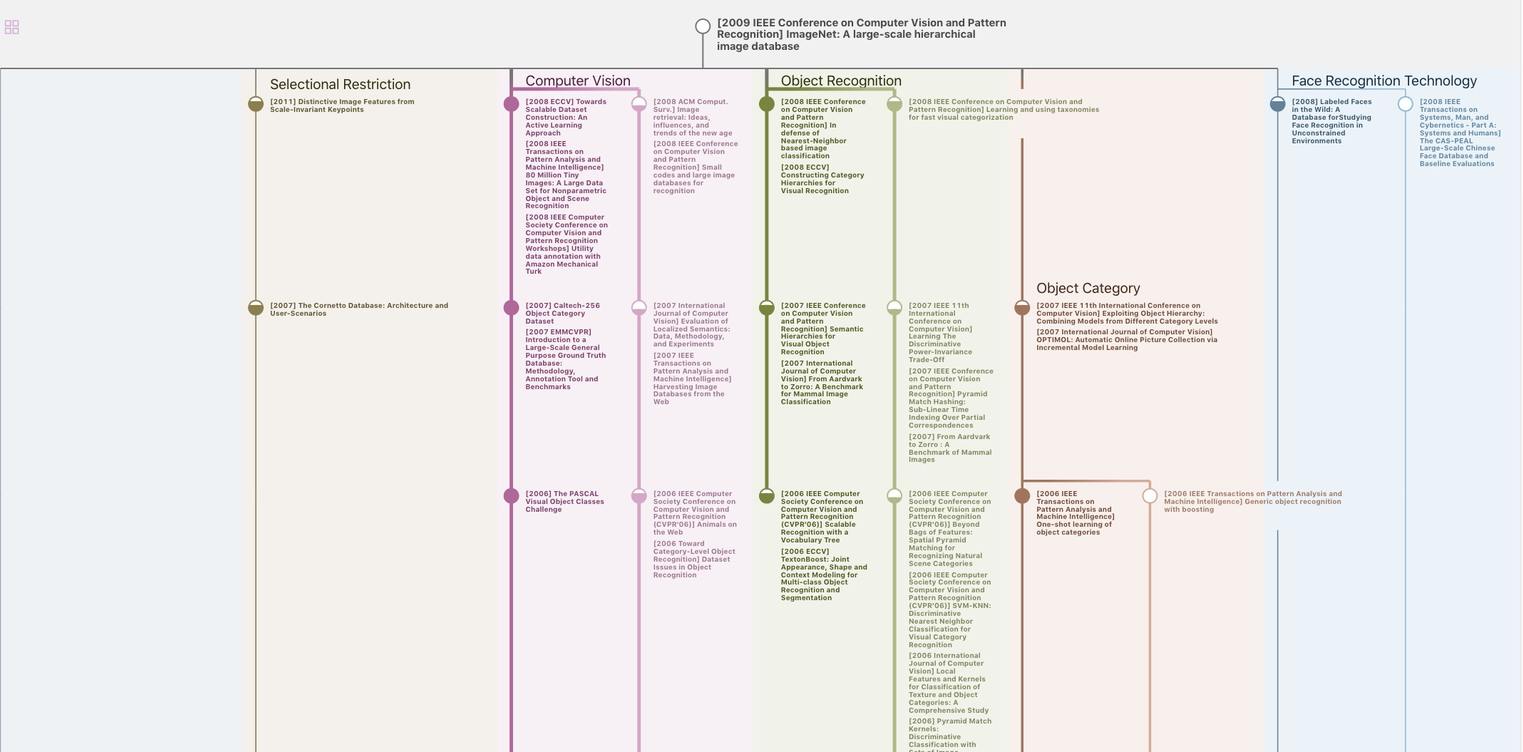
生成溯源树,研究论文发展脉络
Chat Paper
正在生成论文摘要