Inverse design of nanoporous crystalline reticular materials with deep generative models
NATURE MACHINE INTELLIGENCE(2021)
摘要
Reticular frameworks are crystalline porous materials that form via the self-assembly of molecular building blocks in different topologies, with many having desirable properties for gas storage, separation, catalysis, biomedical applications and so on. The notable variety of building blocks makes reticular chemistry both promising and challenging for prospective materials design. Here we propose an automated nanoporous materials discovery platform powered by a supramolecular variational autoencoder for the generative design of reticular materials. We demonstrate the automated design process with a class of metal–organic framework (MOF) structures and the goal of separating carbon dioxide from natural gas or flue gas. Our model shows high fidelity in capturing MOF structural features. We show that the autoencoder has a promising optimization capability when jointly trained with multiple top adsorbent candidates identified for superior gas separation. MOFs discovered here are strongly competitive against some of the best-performing MOFs/zeolites ever reported.
更多查看译文
关键词
Cheminformatics,Metal–organic frameworks,Method development,Renewable energy,Sustainability,Engineering,general
AI 理解论文
溯源树
样例
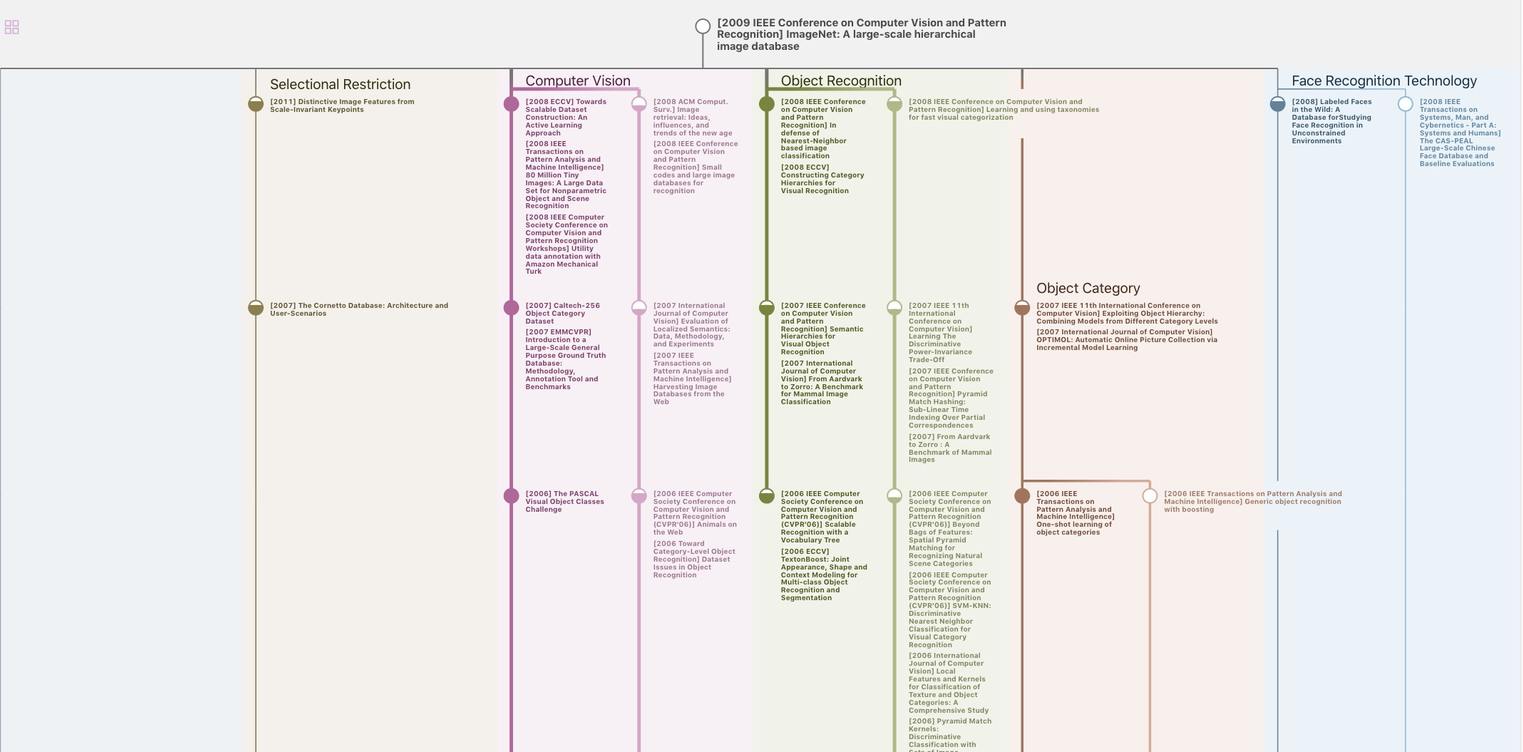
生成溯源树,研究论文发展脉络
Chat Paper
正在生成论文摘要