Massively Distributed Clustering via Dirichlet Process Mixture
MACHINE LEARNING AND KNOWLEDGE DISCOVERY IN DATABASES: APPLIED DATA SCIENCE AND DEMO TRACK, ECML PKDD 2020, PT V(2021)
摘要
Dirichlet Process Mixture (DPM) is a model used for multivariate clustering with the advantage of discovering the number of clusters automatically and offering favorable characteristics, but with prohibitive response times, which makes centralized DPM approaches inefficient. We propose a demonstration of two parallel clustering solutions : i) DC-DPM that gracefully scales to millions of data points while remaining DPM compliant, which is the challenge of distributing this process, ii) HD4C that addresses the curse of dimensionality by performing a distributed DPM clustering of high dimensional data such as time series or hyperspectral data.
更多查看译文
关键词
Gaussian random process, Dirichlet process mixture model, Clustering, Parallelism, Reproducing kernel hilbert space
AI 理解论文
溯源树
样例
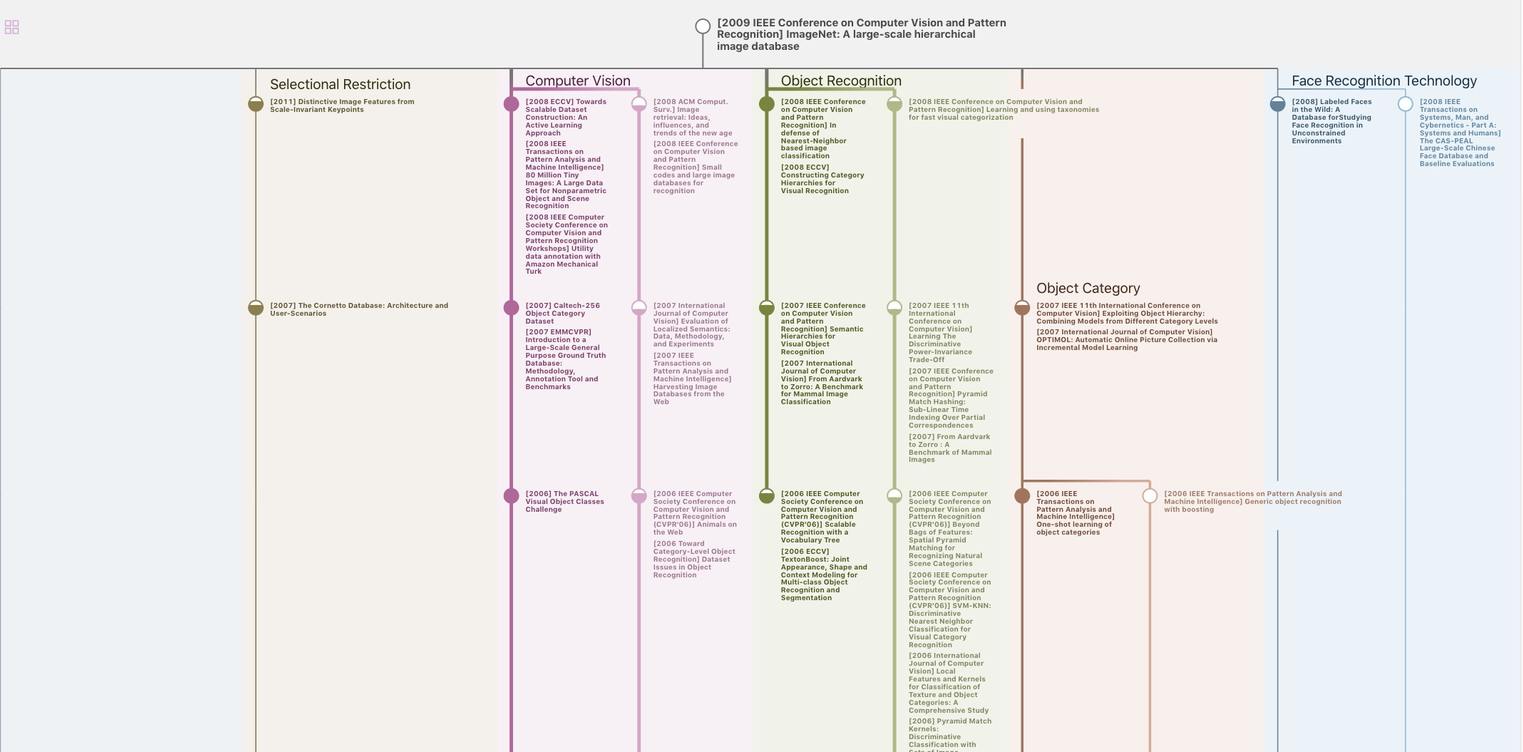
生成溯源树,研究论文发展脉络
Chat Paper
正在生成论文摘要