Robust Naive Learning in Social Networks
arxiv(2021)
摘要
We study a model of opinion exchange in social networks where a state of the world is realized and every agent receives a zero-mean noisy signal of the realized state. It is known from Golub and Jackson that under the DeGroot dynamics agents reach a consensus which is close to the state of the world when the network is large. The DeGroot dynamics, however, is highly non-robust and the presence of a single `bot' that does not adhere to the updating rule, can sway the public consensus to any other value. We introduce a variant of the DeGroot dynamics which we call \emph{ $\varepsilon$-DeGroot}. The $\varepsilon$-DeGroot dynamics approximates the standard DeGroot dynamics and like the DeGroot dynamics it is Markovian and stationary. We show that in contrast to the standard DeGroot dynamics, the $\varepsilon$-DeGroot dynamics is highly robust both to the presence of bots and to certain types of misspecifications.
更多查看译文
关键词
robust naive learning,social networks
AI 理解论文
溯源树
样例
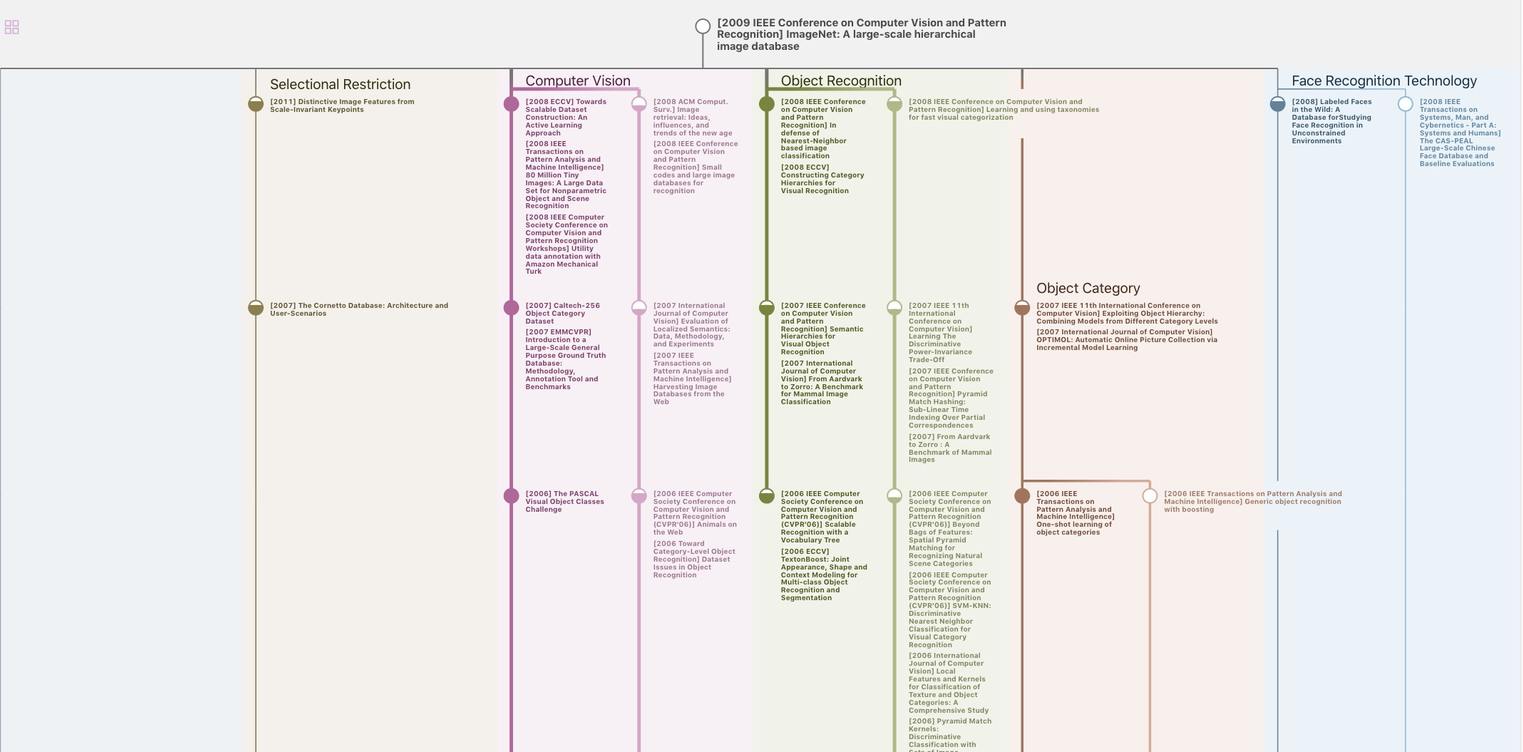
生成溯源树,研究论文发展脉络
Chat Paper
正在生成论文摘要