SBI: A Simulation-Based Test of Identifiability for Bayesian Causal Inference
arxiv(2022)
摘要
A growing family of approaches to causal inference rely on Bayesian formulations of assumptions that go beyond causal graph structure. For example, Bayesian approaches have been developed for analyzing instrumental variable designs, regression discontinuity designs, and within-subjects designs. This paper introduces simulation-based identifiability (SBI), a procedure for testing the identifiability of queries in Bayesian causal inference approaches that are implemented as probabilistic programs. SBI complements analytical approaches to identifiability, leveraging a particle-based optimization scheme on simulated data to determine identifiability for analytically intractable models. We analyze SBI's soundness for a broad class of differentiable, finite-dimensional probabilistic programs with bounded effects. Finally, we provide an implementation of SBI using stochastic gradient descent, and show empirically that it agrees with known identification results on a suite of graph-based and quasi-experimental design benchmarks, including those using Gaussian processes.
更多查看译文
关键词
bayesian causal
AI 理解论文
溯源树
样例
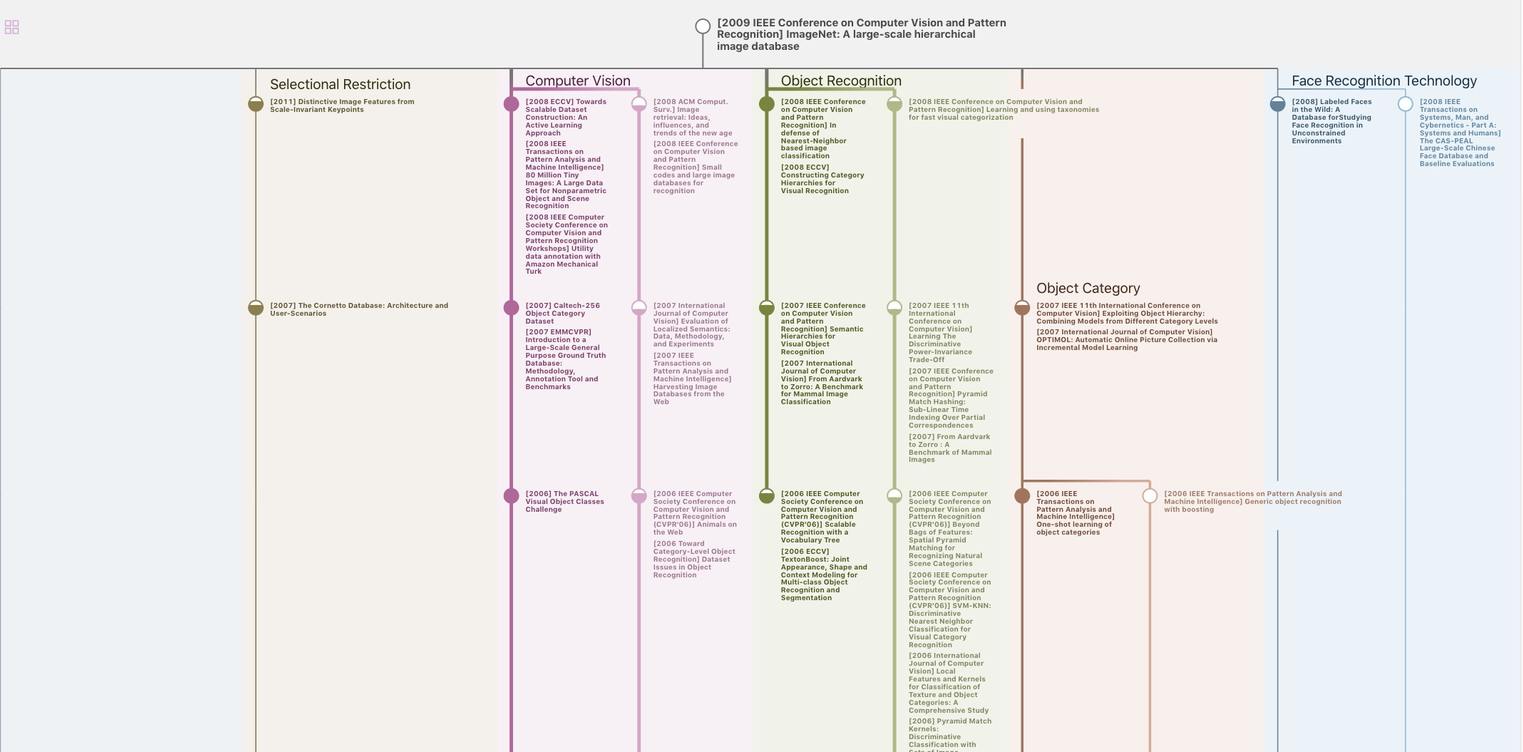
生成溯源树,研究论文发展脉络
Chat Paper
正在生成论文摘要