Cerebrovascular segmentation from TOF-MRA based on multiple-U-net with focal loss function
Computer Methods and Programs in Biomedicine(2021)
Abstract
Background and Objective: Accurate cerebrovascular segmentation plays an important role in the diagnosis of cerebrovascular diseases. Considering the complexity and uncertainty of doctors? manual segmentation of cerebral vessels, this paper proposed an automatic segmentation algorithm based on Multiple-U-net (M-U-net) to segment cerebral vessel structures from the Time-of-flight Magnetic Resonance Angiography (TOF-MRA) data. Methods: First, the TOF-MRA data was normalized by volume and then divided into three groups through slices of axial, coronal and sagittal directions respectively. Three single U-nets were trained by divided dataset. To solve the problem of uneven distribution of positive and negative samples, the focal loss function was adopted in training. After obtaining the prediction results of three single U-nets, the voting feature fusion and the post-processing process based on connected domain analysis would be performed. 95 volumes of TOF-MRA provided by the MIDAS platform were applied to the experiment, among which 20 volumes were treated as the training dataset, 5 volumes were used as the validation dataset and the remaining 70 volumes were divided into 10 groups to test the trained model respectively. Results: Experiments showed that the proposed M-U-net based algorithm achieved 88.60% and 87.93% Dice Similarity Coefficient (DSC) on the verification dataset and testing dataset, which performed better than any single U-net. Conclusions: Compared with other existing algorithms, our algorithm reached the state of the art level. The feature fusion of three single U-nets could effectively complement the segmentation results. ? 2021 Elsevier B.V. All rights reserved.
MoreTranslated text
Key words
Cerebrovascular segmentation,U-net,Focal loss function,Feature fusion
AI Read Science
Must-Reading Tree
Example
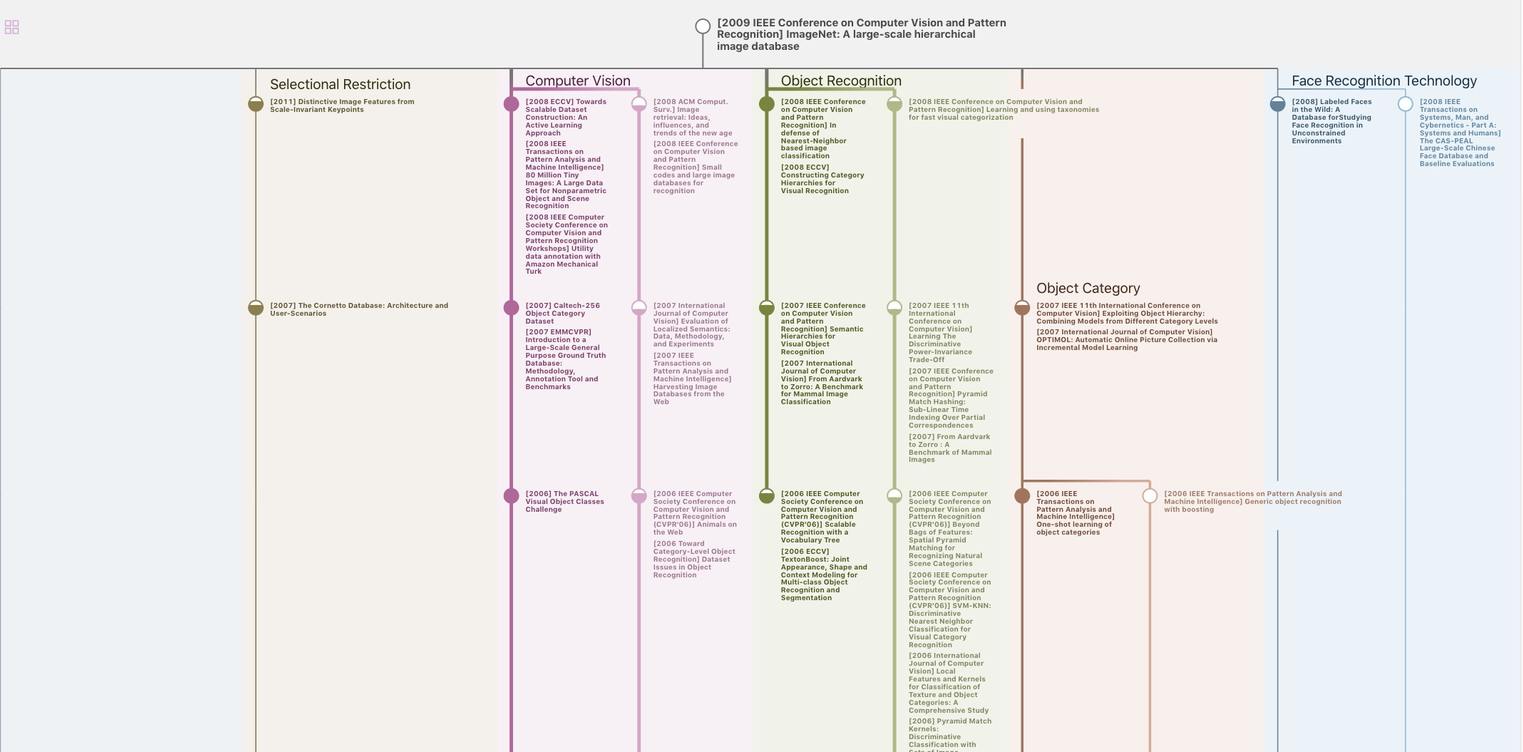
Generate MRT to find the research sequence of this paper
Chat Paper
Summary is being generated by the instructions you defined