Satellite Data Fusion Of Multiple Observed Xco2 Using Compressive Sensing And Deep Learning
IGARSS 2020 - 2020 IEEE INTERNATIONAL GEOSCIENCE AND REMOTE SENSING SYMPOSIUM(2020)
摘要
Providing climate data records to infer seasonal and interannual variations from multiple heterogeneous sources is a challenging data fusion. We combine the Compressive Sensing (CS) and Deep Learning (DL) into a single framework for fusing data from multiple sensors to improve spatial and temporal resolution for long term analysis. CS is used as an initial guess to combine data from multiple sources. DL models, using Long Short-Term Memory Neural Network (LSTM/RNN), Convolutional Neural Network (CNN), refine and further improve the data fusion from CS algorithm's output. The proposed framework has been tested the using daily global observations from two satellites the NASA Orbiting Carbon Observatory-2 (OCO-2) and the JAXA Greenhouse gases from Orbiting Satellites (GOSAT). Our framework achieves lower errors and high correlation compared with the original data. The quality of fused data is evaluated by comparing again AmeriFlux ground station's datasets. Long term trends using fused data over the United States indicate an increase of 8 parts per million (ppm) annually in XCO2 over four years with Root Mean Square Errors of 0.39 ppm and correlation of 0.98 compared with original data. Interannual variability of the seasonal cycle shows an increase in years 2015 - 2017, but a sharp decrease in 2018.
更多查看译文
关键词
Compressive Sensing, Deep Learning, LSTM, Convolutional Neural Network, Data fusion, XCO2, OCO-2, GOSAT
AI 理解论文
溯源树
样例
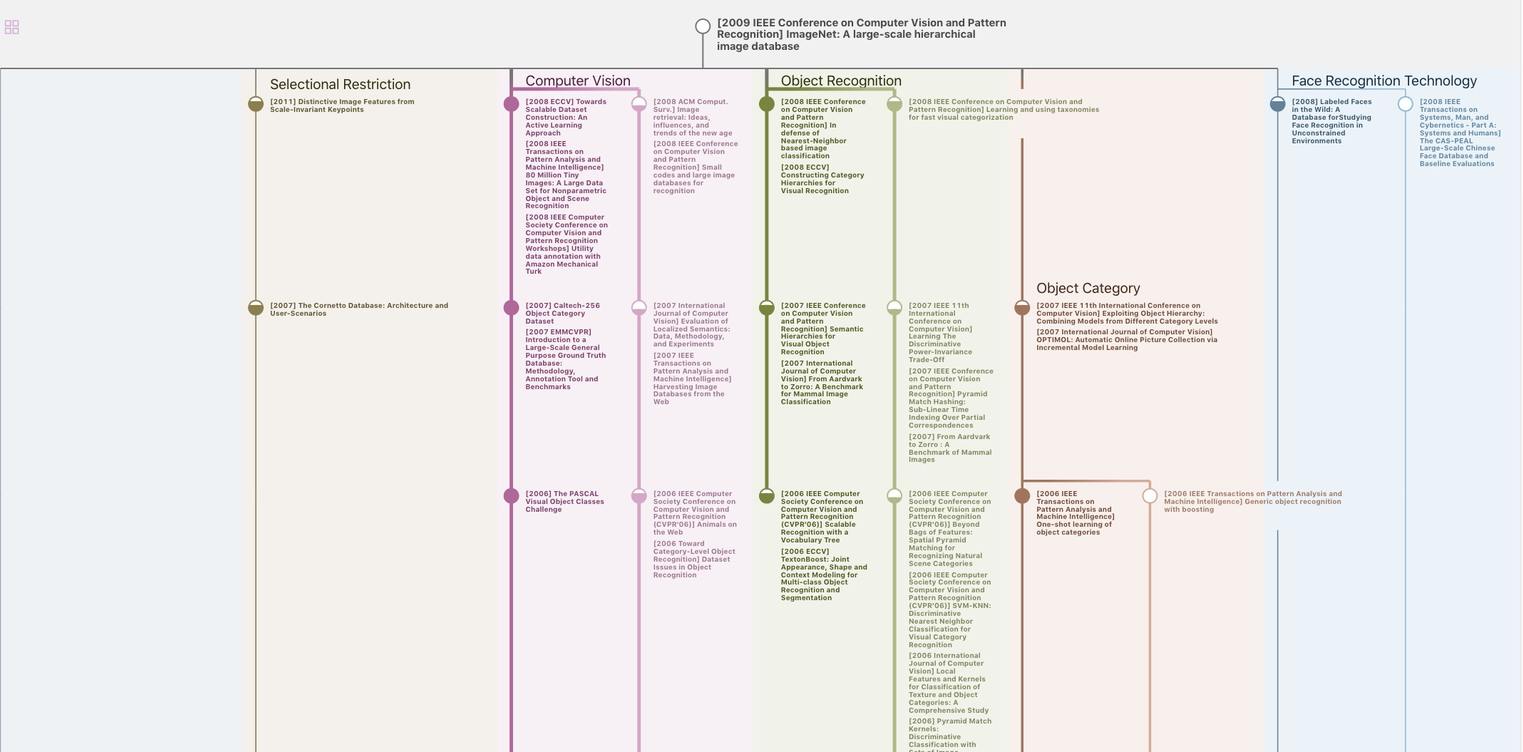
生成溯源树,研究论文发展脉络
Chat Paper
正在生成论文摘要