A Fast Low Rank Approximation And Sparsity Representation Approach To Hyperspectral Anomaly Detection
IGARSS 2020 - 2020 IEEE INTERNATIONAL GEOSCIENCE AND REMOTE SENSING SYMPOSIUM(2020)
摘要
Anomaly detection is an important application in hyperspectral data exploitation. This paper develops a novel fast low rank approximation and sparsity representation approach to anomaly detection. It first uses a standard random projection approach to construct low rank matrix, and then use it to calculate sparsity representation, S. Secondly, continuous to decompose S to get new low rank and sparsity representation until S converges into predefined threshold. Sum all S in the iteration process. Thirdly, using the summation of S to construct original matrix's low rank. Finally, comparing with state-of-art methods like Go Decomposition (GoDec), our method is seven times faster than GoDec's. The experimental on real hyperspectral images results indicate that the detection power of Reed-Xiaoli detector (RXD) is approximately increased 11% based on our sparsity representation comparing with GoDec method but the false alarm is lower than GoDec's.
更多查看译文
关键词
Anomaly detection, Low Rank, sparse representation, Reed-Xiaoli detector (RXD), GoDec
AI 理解论文
溯源树
样例
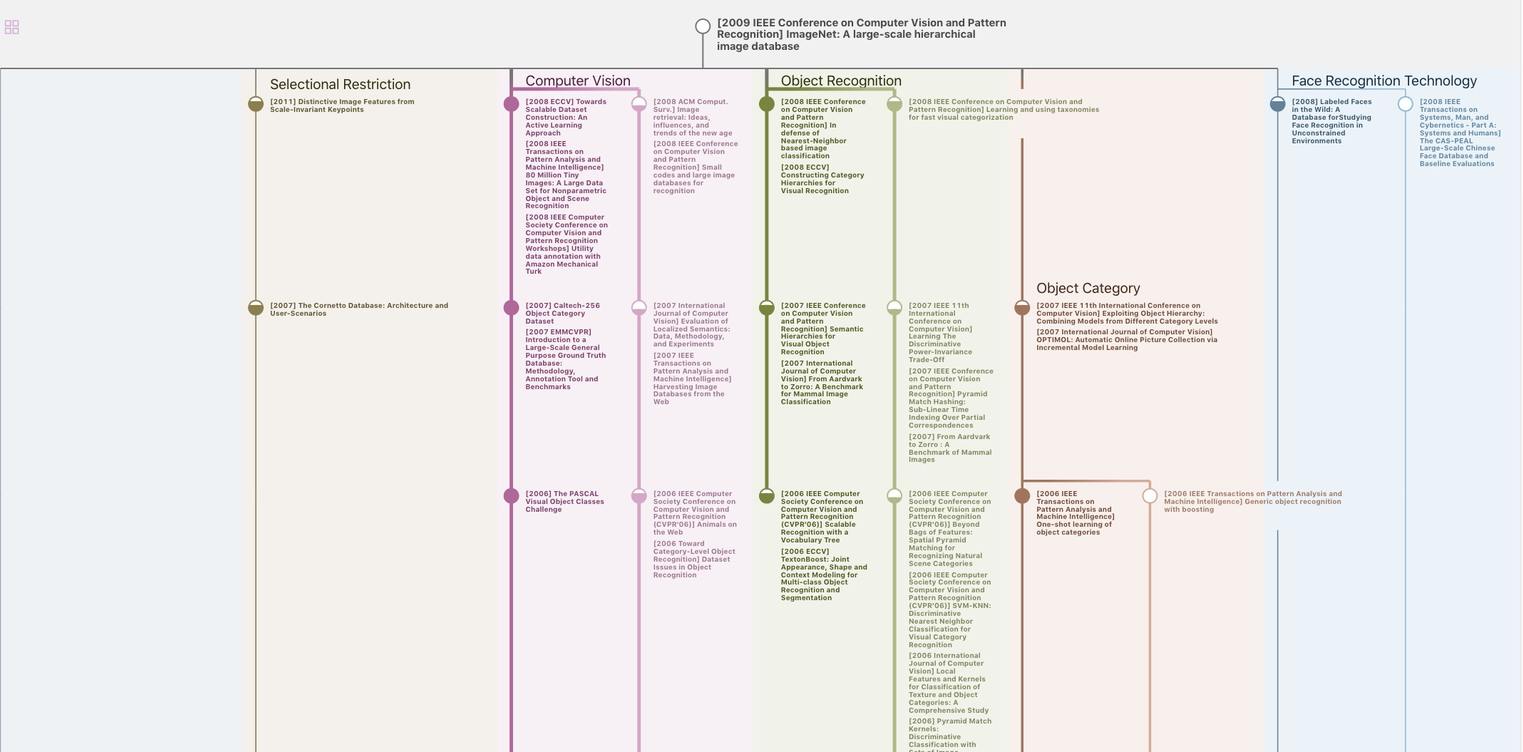
生成溯源树,研究论文发展脉络
Chat Paper
正在生成论文摘要