Dem Extraction From Airborne Lidar Point Cloud In Thick-Forested Areas Via Convolutional Neural Network
IGARSS 2020 - 2020 IEEE INTERNATIONAL GEOSCIENCE AND REMOTE SENSING SYMPOSIUM(2020)
Abstract
Digital Elevation Model (DEM),representing the height of the earth terrain, is one of the crucial geographic information products. One of the main data source of DEM is the airborne LiDAR point cloud with its non-ground-reflections filtered out. Point cloud filtering in thick-forested areas is difficult without enough ground control points when using conventional methods. In this paper, a supervised method is proposed to handle the problem of automatic DEM extraction with little ground control points. The design of the method is inspired by the successful application of the convolutional neural networks (CNN) in the image super resolution (SR) process. First, with the given LiDAR point cloud, the digital surface model (DSM) is resampled with regular grid. Then, by learning the spatial autocorrelation between the DSM and its corresponding DEM, a robust CNN model is established. Finally, the DEM in thick-forested areas can be generated from the DSM with the trained model. Experimental results at two different mountain sites in China validate the effectiveness of the proposed method of high-precision DEM generation.
MoreTranslated text
Key words
Digital Elevation Model (DEM), Digital Surface Model (DSM), convolutional neural network (CNN), LiDAR point cloud, DEM extraction
AI Read Science
Must-Reading Tree
Example
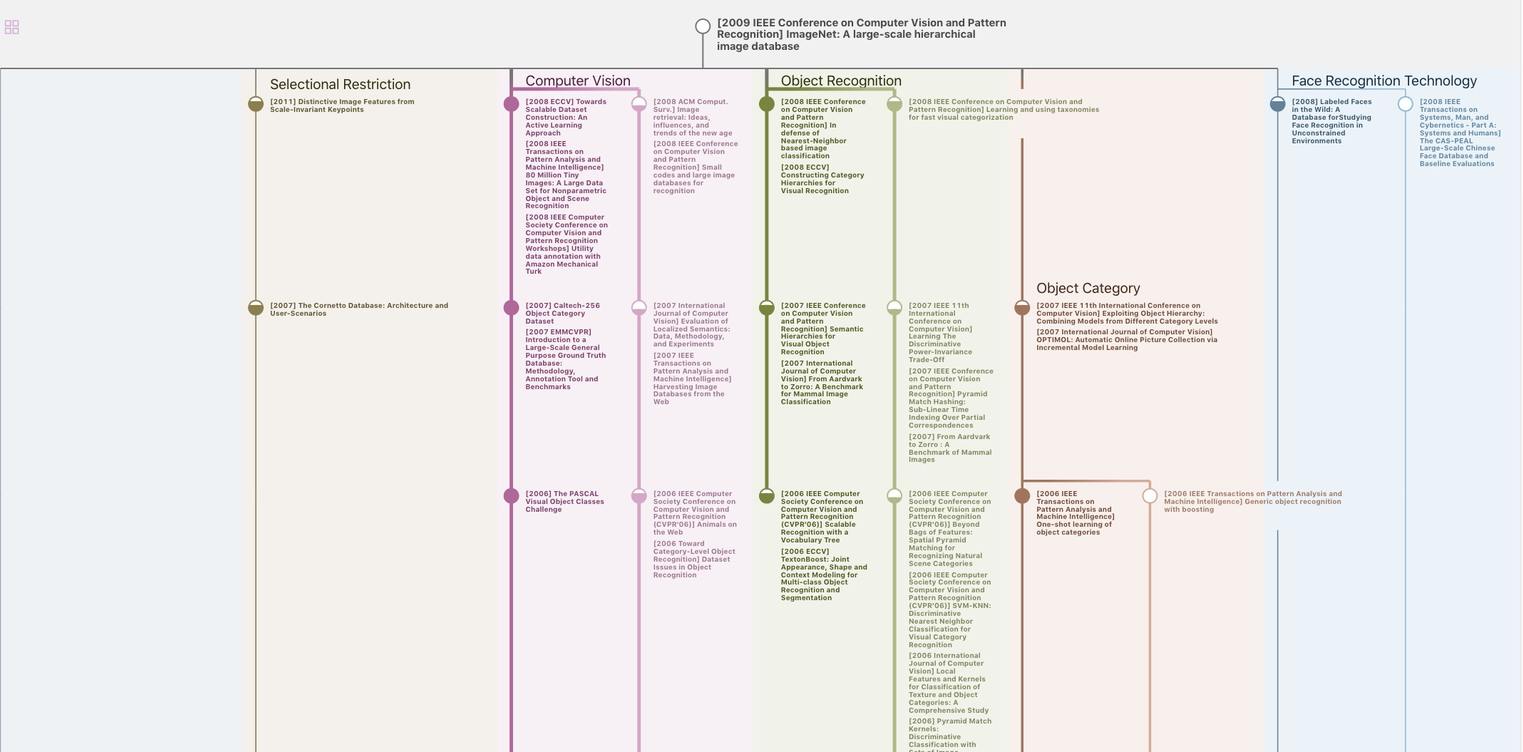
Generate MRT to find the research sequence of this paper
Chat Paper
Summary is being generated by the instructions you defined