Crop Height Estimation Of Corn From Multi-Year Radarsat-2 Polarimetric Observables Using Machine Learning
REMOTE SENSING(2021)
摘要
This study presents a demonstration of the applicability of machine learning techniques for the retrieval of crop height in corn fields using space-borne PolSAR (Polarimetric Synthetic Aperture Radar) data. Multi-year RADARSAT-2 C-band data acquired over agricultural areas in Canada, covering the whole corn growing period, are exploited. Two popular machine learning regression methods, i.e., Random Forest Regression (RFR) and Support Vector Regression (SVR) are adopted and evaluated. A set of 27 representative polarimetric parameters are extracted from the PolSAR data and used as input features in the regression models for height estimation. Furthermore, based on the unique capability of the RFR method to determine variable importance contributing to the regression, a smaller number of polarimetric features (6 out of 27 in our study) are selected in the final regression models. Results of our study demonstrate that PolSAR observables can produce corn height estimates with root mean square error (RMSE) around 40-50 cm throughout the growth cycle. The RFR approach shows better overall accuracy in corn height estimation than the SVR method in all tests. The six selected polarimetric features by variable importance ranking can generate better results. This study provides a new perspective on the use of PolSAR data in retrieving agricultural crop height from space.
更多查看译文
关键词
crop height, RADARSAT-2, corn, Synthetic Aperture Radar (SAR), PolSAR, machine learning, RFR, SVR, agriculture
AI 理解论文
溯源树
样例
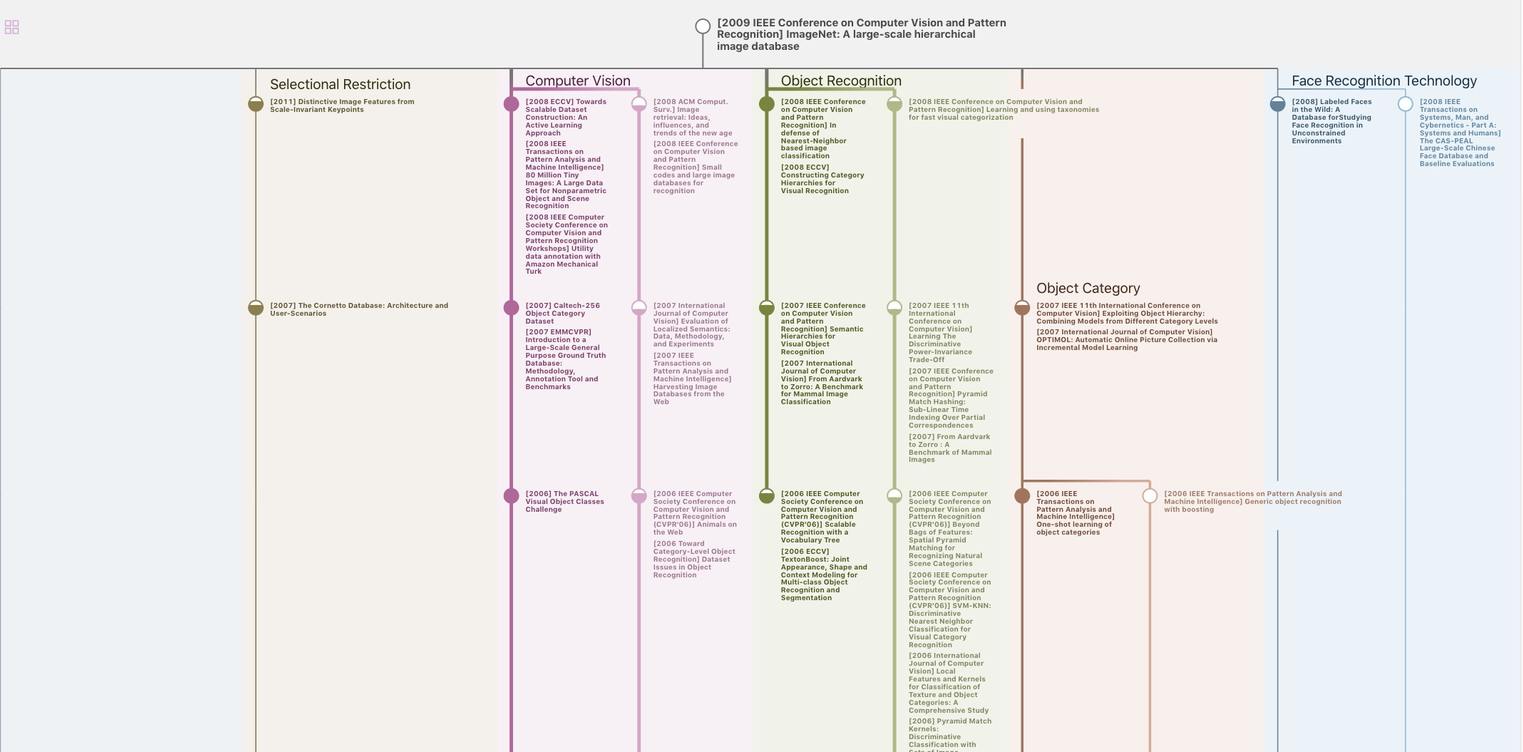
生成溯源树,研究论文发展脉络
Chat Paper
正在生成论文摘要