ACTOR-CRITIC METHOD FOR HIGH DIMENSIONAL STATIC HAMILTON-JACOBI-BELLMAN PARTIAL DIFFERENTIAL EQUATIONS BASED ON NEURAL NETWORKS
SIAM JOURNAL ON SCIENTIFIC COMPUTING(2021)
摘要
We propose a novel numerical method for high dimensional Hamilton-JacobiBellman (HJB) type elliptic partial differential equations (PDEs). The HJB PDEs, reformulated as optimal control problems, are tackled by the actor-critic framework inspired by reinforcement learning, based on neural network parametrization of the value and control functions. Within the actor-critic framework, we employ a policy gradient approach to improve the control, while for the value function, we derive a variance reduced least-squares temporal difference method using stochastic calculus. To numerically discretize the stochastic control problem, we employ an adaptive step size scheme to improve the accuracy near the domain boundary. Numerical examples up to 20 spatial dimensions including the linear quadratic regulators, the stochastic Van der Pol oscillators, the diffusive Eikonal equations, and fully nonlinear elliptic PDEs derived from a regulator problem are presented to validate the effectiveness of our proposed method.
更多查看译文
关键词
Hamilton-Jacobi-Bellman equations, high dimensional partial differential equa-tions, stochastic control, actor-critic methods
AI 理解论文
溯源树
样例
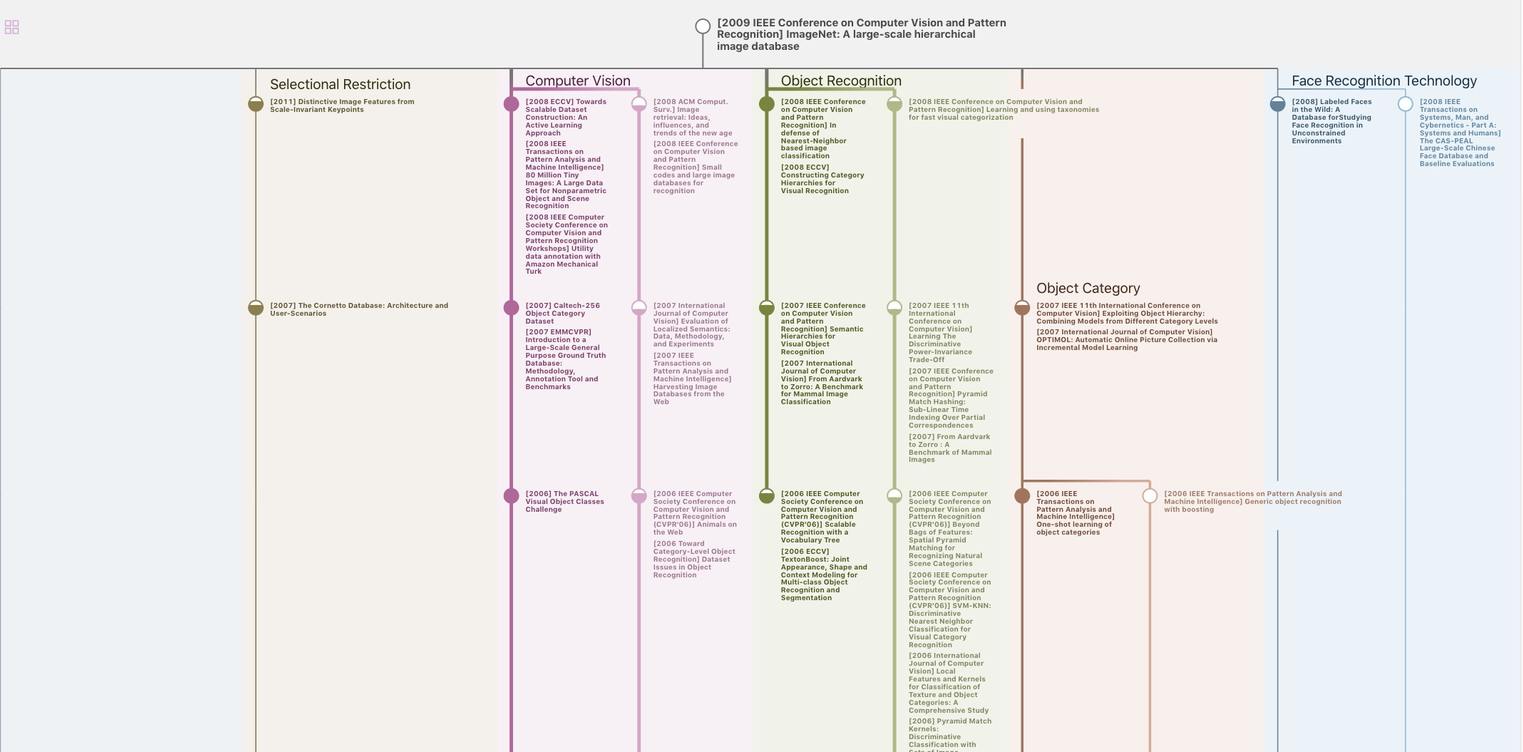
生成溯源树,研究论文发展脉络
Chat Paper
正在生成论文摘要