Deep Reinforcement Learning for Dynamic Spectrum Sharing of LTE and NR
IEEE INTERNATIONAL CONFERENCE ON COMMUNICATIONS (ICC 2021)(2021)
摘要
In this paper, a proactive dynamic spectrum sharing scheme between 4G and 5G systems is proposed. In particular, a controller decides on the resource split between NR and LTE every subframe while accounting for future network states such as high interference subframes and multimedia broadcast single frequency network (MBSFN) subframes. To solve this problem, a deep reinforcement learning (RL) algorithm based on Monte Carlo Tree Search (MCTS) is proposed. The introduced deep RL architecture is trained offline whereby the controller predicts a sequence of future states of the wireless access network by simulating hypothetical bandwidth splits over time starting from the current network state. The action sequence resulting in the best reward is then assigned. This is realized by predicting the quantities most directly relevant to planning, i.e., the reward, the action probabilities, and the value for each network state. Simulation results show that the proposed scheme is able to take actions while accounting for future states instead of being greedy in each subframe. The results also show that the proposed framework improves system-level performance.
更多查看译文
关键词
dynamic spectrum sharing,lte
AI 理解论文
溯源树
样例
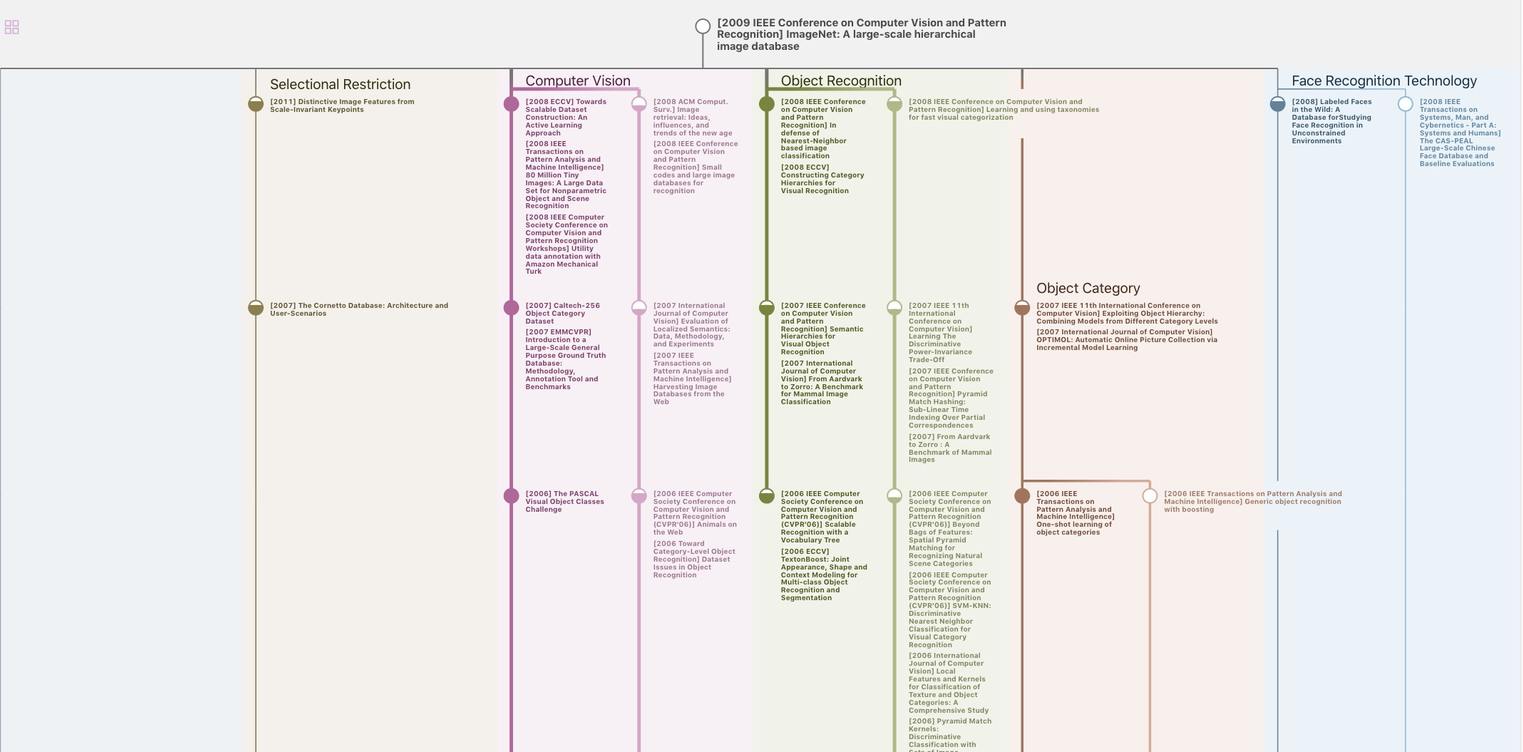
生成溯源树,研究论文发展脉络
Chat Paper
正在生成论文摘要