Safe Policy Learning with Constrained Return Variance.
ADVANCES IN ARTIFICIAL INTELLIGENCE(2019)
摘要
It is desirable for a safety-critical application that the agent performs in a reliable and repeatable manner which conventional setting in reinforcement learning (RL) often fails to provide. In this work, we derive a novel algorithm to learn a safe hierarchical policy by constraining the direct estimate of the variance in the return in the Option- Critic framework [1]. We first present the novel theorem of safe control in the policy gradient methods and then extend the derivation to the Option-Critic framework.
更多查看译文
关键词
Safety,Policy gradient,Option-Critic
AI 理解论文
溯源树
样例
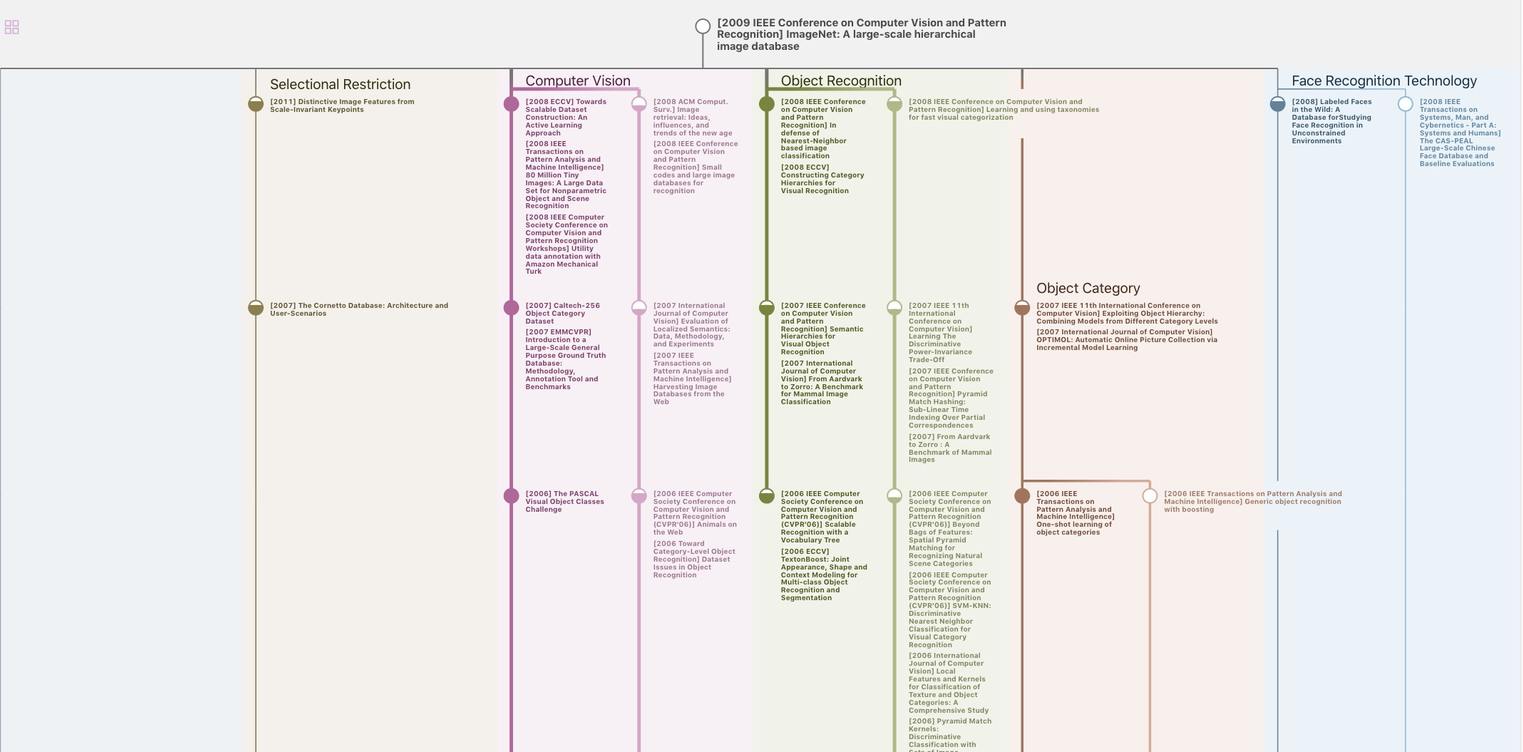
生成溯源树,研究论文发展脉络
Chat Paper
正在生成论文摘要