Rhythm Classification Of 12-Lead Ecgs Using Deep Neural Networks And Class-Activation Maps For Improved Explainability
2020 COMPUTING IN CARDIOLOGY(2020)
摘要
As part of the PhysioNet/Computing in Cardiology Challenge 2020, we developed a model for multilabel classification of 12-lead electrocardiogram (ECG) data according to specified cardiac abnormalities. Our team, LaussenLabs, developed a novel classifier pipeline with 6 core features (1) the addition of r-peak, p-wave, and t-wave features that were input into the model along with the 12-lead data, (2) data augmentation, (3) competition metric hacking, (4) modified WaveNet architecture, (5) Sigmoid threshold tuning, and (6) model stacking. Our approach received a score of 0.63 using 6-fold cross-validation on the full training data. Unfortunately, our model was unable to run on the test dataset due to time constraints, therefore, our model's final test score is undetermined.
更多查看译文
关键词
rhythm classification,12-lead ECGs,deep neural networks,class-activation maps,improved explainability,Cardiology Challenge 2020,multilabel classification,12-lead electrocardiogram data,ECG,specified cardiac abnormalities,classifier pipeline,6 core features,t-wave features,12-lead data,training data
AI 理解论文
溯源树
样例
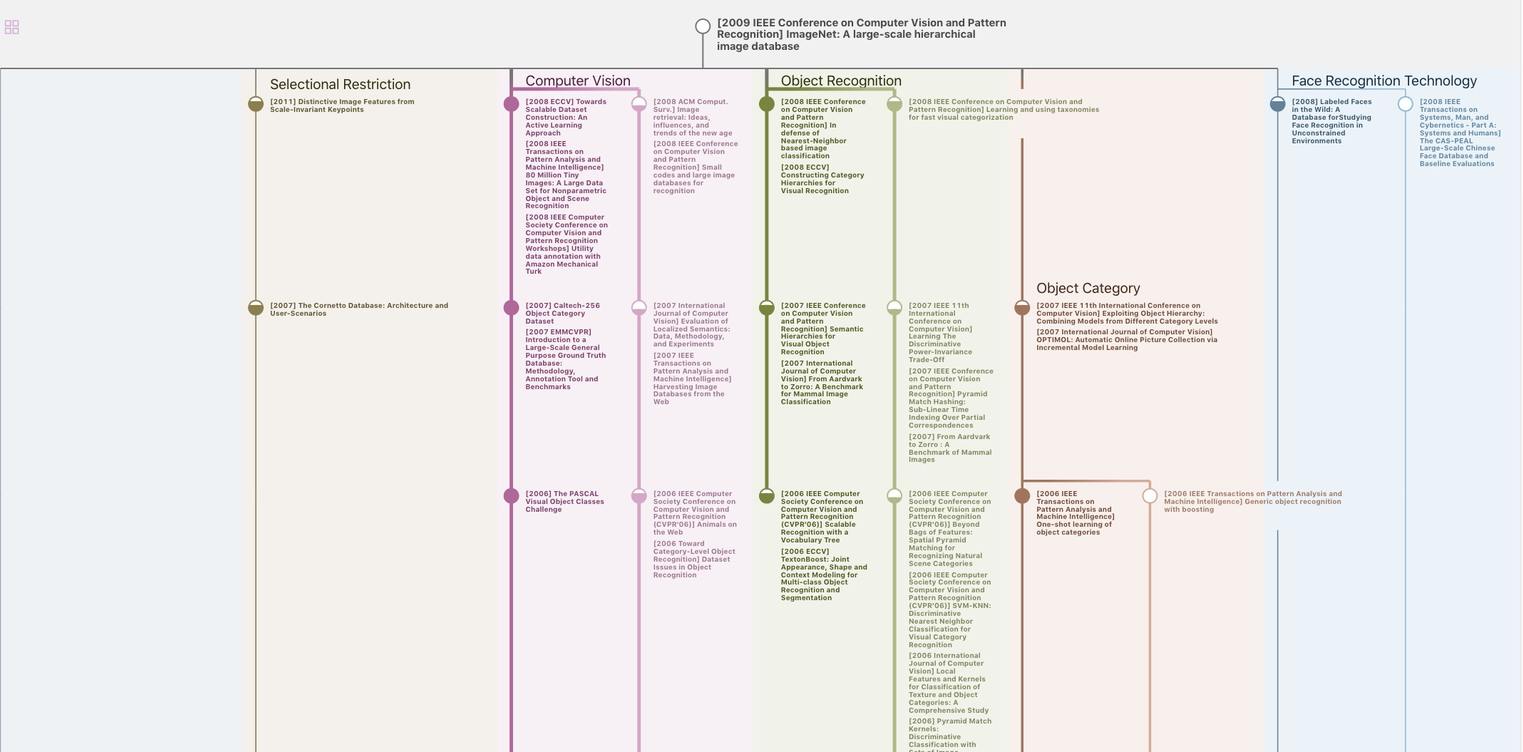
生成溯源树,研究论文发展脉络
Chat Paper
正在生成论文摘要