Weighted Error Entropy-Based Information Theoretic Learning for Robust Subspace Representation
IEEE Transactions on Neural Networks and Learning Systems(2022)
摘要
In most of the existing representation learning frameworks, the noise contaminating the data points is often assumed to be independent and identically distributed (
i.i.d.
), where the Gaussian distribution is often imposed. This assumption, though greatly simplifies the resulting representation problems, may not hold in many practical scenarios. For example, the noise in face representation is usually attributable to local variation, random occlusion, and unconstrained illumination, which is essentially structural, and hence, does not satisfy the
i.i.d.
property or the Gaussianity. In this article, we devise a generic noise model, referred to as independent and piecewise identically distributed (
i.p.i.d.
) model for robust presentation learning, where the statistical behavior of the underlying noise is characterized using a union of distributions. We demonstrate that our proposed
i.p.i.d.
model can better describe the complex noise encountered in practical scenarios and accommodate the traditional
i.i.d.
one as a special case. Assisted by the proposed noise model, we then develop a new information-theoretic learning framework for robust subspace representation through a novel minimum weighted error entropy criterion. Thanks to the superior modeling capability of the
i.p.i.d.
model, our proposed learning method achieves superior robustness against various types of noise. When applying our scheme to the subspace clustering and image recognition problems, we observe significant performance gains over the existing approaches.
更多查看译文
关键词
Independent and piecewise identically distributed,information-theoretic learning (ITL),subspace representation (SR),weighted Parzen window (WPW)
AI 理解论文
溯源树
样例
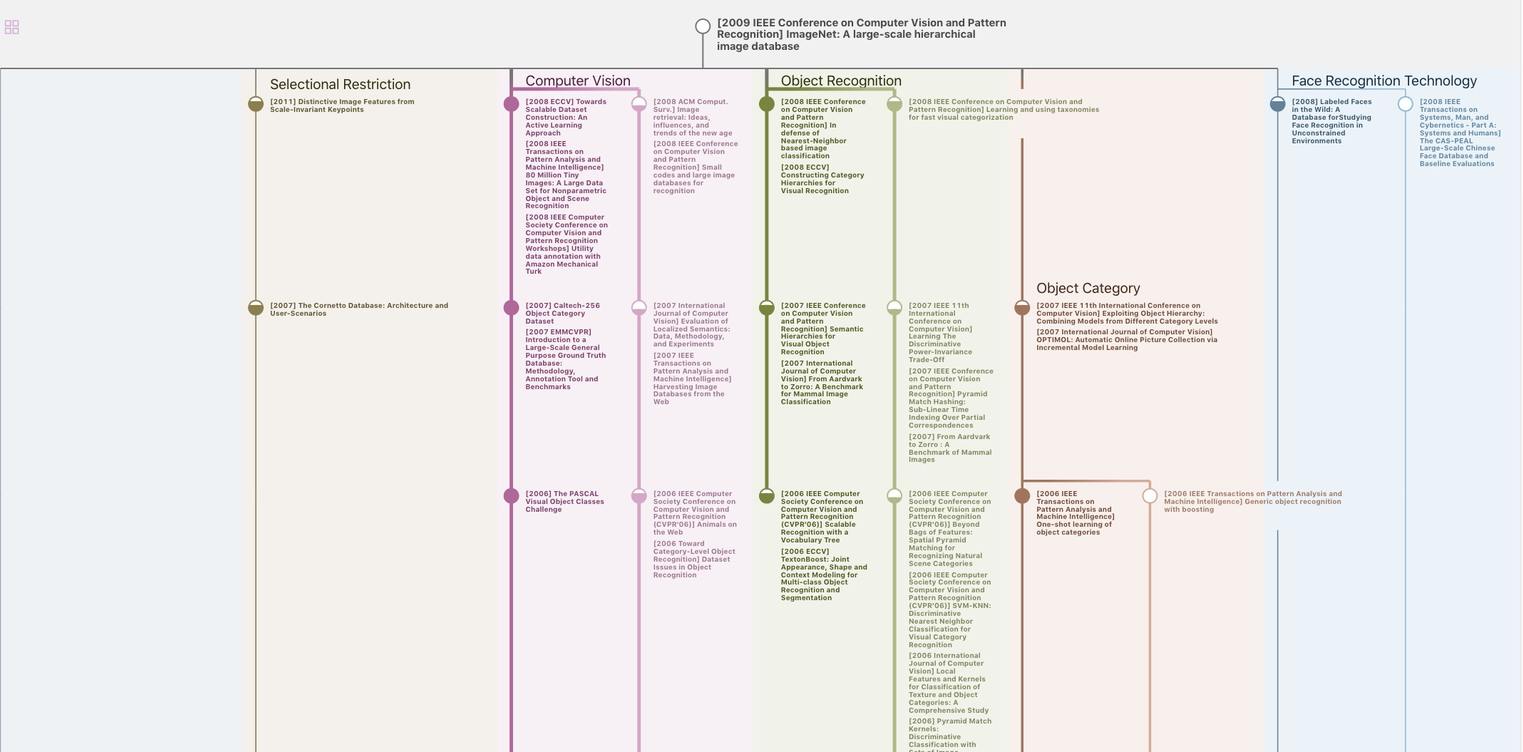
生成溯源树,研究论文发展脉络
Chat Paper
正在生成论文摘要