Development And Validation Of A Machine Learning Prognostic Model For Hepatocellular Carcinoma Recurrence After Surgical Resection
FRONTIERS IN ONCOLOGY(2021)
摘要
Surgical resection remains primary curative treatment for patients with hepatocellular carcinoma (HCC) while over 50% of patients experience recurrence, which calls for individualized recurrence prediction and early surveillance. This study aimed to develop a machine learning prognostic model to identify high-risk patients after surgical resection and to review importance of variables in different time intervals. The patients in this study were from two centers including Eastern Hepatobiliary Surgery Hospital (EHSH) and Mengchao Hepatobiliary Hospital (MHH). The best-performed model was determined, validated, and applied to each time interval (0-1 year, 1-2 years, 2-3 years, and 3-5 years). Importance scores were used to illustrate feature importance in different time intervals. In addition, a risk heat map was constructed which visually depicted the risk of recurrence in different years. A total of 7,919 patients from two centers were included, of which 3,359 and 230 patients experienced recurrence, metastasis or died during the follow-up time in the EHSH and MHH datasets, respectively. The XGBoost model achieved the best discrimination with a c-index of 0.713 in internal validation cohort. Kaplan-Meier curves succeed to stratify external validation cohort into different risk groups (p < 0.05 in all comparisons). Tumor characteristics contribute more to HCC relapse in 0 to 1 year while HBV infection and smoking affect patients' outcome largely in 3 to 5 years. Based on machine learning prediction model, the peak of recurrence can be predicted for individual HCC patients. Therefore, clinicians can apply it to personalize the management of postoperative survival.
更多查看译文
关键词
hepatocellular carcinoma, recurrence, machine learning, modeling, prognosis
AI 理解论文
溯源树
样例
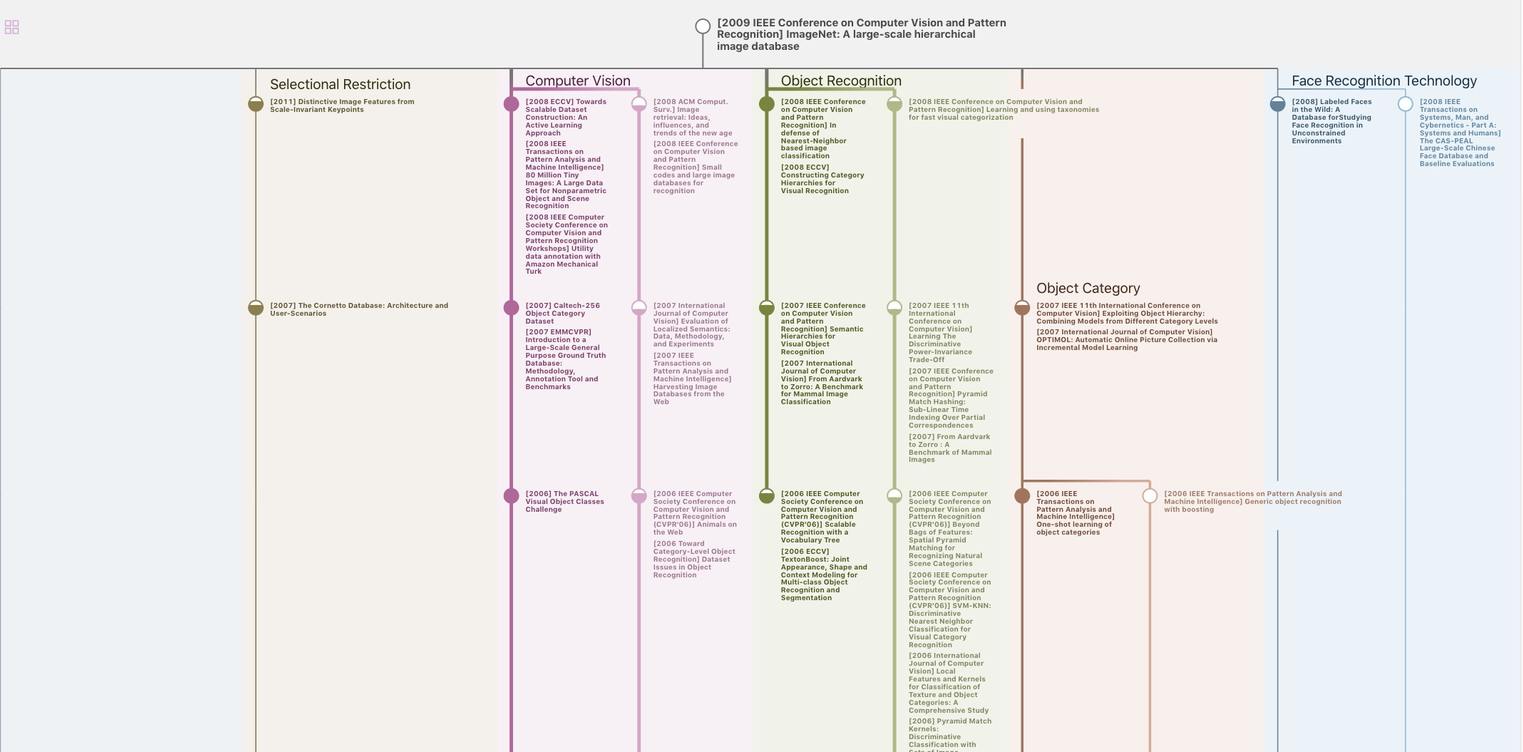
生成溯源树,研究论文发展脉络
Chat Paper
正在生成论文摘要