A Flexible Attentive Temporal Graph Networks for Anomaly Detection in Dynamic Networks
TrustCom(2020)
Abstract
Anomaly Detection in Dynamic Networks plays a critical role in various real-world applications such as cybersecurity, e-commerce, and social media. Recent approaches based on graph neural networks have achieved fruitful results in static networks, and most of the researches focus on learning node embeddings to represent the large-scale complex networks, thereby facilitating downstream anomaly detection task. However, these methods require the whole network to extract intrinsic properties, so they are sensitive to the frequent changes of nodes and incapable of capturing the dynamism as real world networks evolve over time. In this paper, we propose a novel framework DynAD for anomalous edge detection on time-evolving networks, which performs adaptive parameter learning in an end-to-end manner. In particular, DynAD first extracts fixed-size node embedding from each snapshot with temporal graph convolution and pooling operations. Then, it captures the temporal information of the graph sequence using Gated recurrent units (GRU) for anomaly detection, where an attention mechanism is employed to highlight the differences between the dynamic patterns. Experimental results on three real-world datasets illustrate that DynAD significantly outperforms the state-of-the-art baseline methods in anomaly detection.
MoreTranslated text
Key words
Anomaly Detection, Dynamic Networks, Graph Embedding, Graph Convolutional Network
AI Read Science
Must-Reading Tree
Example
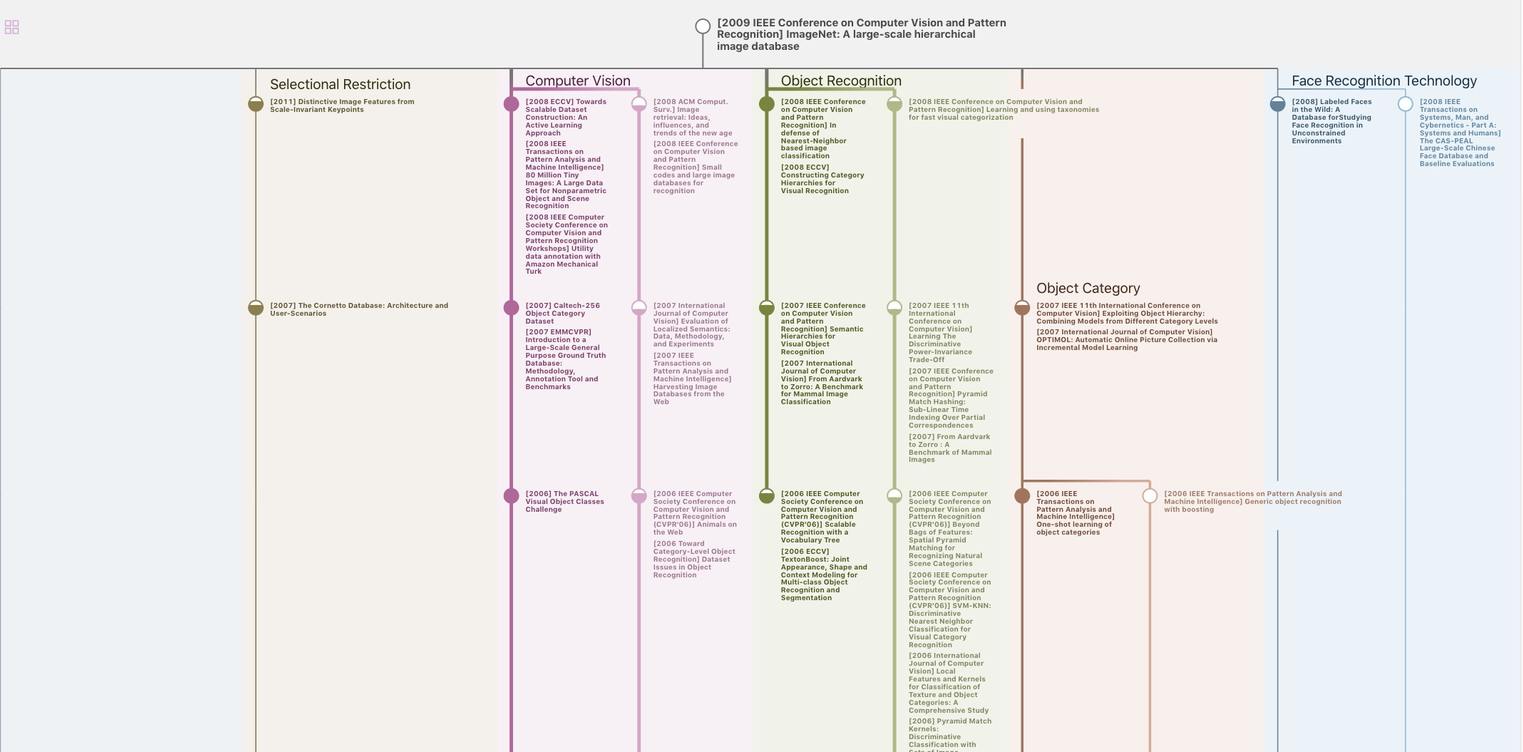
Generate MRT to find the research sequence of this paper
Chat Paper
Summary is being generated by the instructions you defined