Improving Interpretability for Cyber Vulnerability Assessment Using Focus and Context Visualizations
2020 IEEE Symposium on Visualization for Cyber Security (VizSec)(2020)
摘要
Risk scoring provides a simple and quantifiable metric for decision support in cyber security operations, including prioritizing how to address discovered software vulnerabilities. However, scoring systems are often opaque to operators, which makes scores difficult to interpret in the context of their own networks, each other, or in a broader threat landscape. This interpretability challenge is exacerbated by recent applications of artificial intelligence (AI) and machine learning (ML) for vulnerability assessment, where opaque machine reasoning can hinder domain experts' trust in the decision-support toolkit or the actionability of its outputs. In this paper, we address this challenge through a combination of visualizations and analytics that complement existing techniques for vulnerability assessment. We present a study toward designing more interpretable visual encodings for decision support for vulnerability assessment. In particular, we consider the problem of making datasets of known vulnerabilities more interpretable at multiple scales, inspired by focus and context principles from the information visualization design community. The first scale considers individually scored vulnerabilities by using an explainable AI (XAI) toolkit for an ML risk-scoring model and by developing new visualizations of CVSS score features. The second scale uses an embedding for vulnerability descriptions to cluster potentially similar vulnerabilities. We outline use cases for these tools and discuss opportunities for applying XAI concepts to cyber risk and vulnerability management.
更多查看译文
关键词
Human-centered computing—Visualization—Visualization systems and tools—Visualization toolkits,Human-centered computing—Visualization—Visualization application domains—Information Visualization,Security and privacy—Systems security—Vulnerability Management
AI 理解论文
溯源树
样例
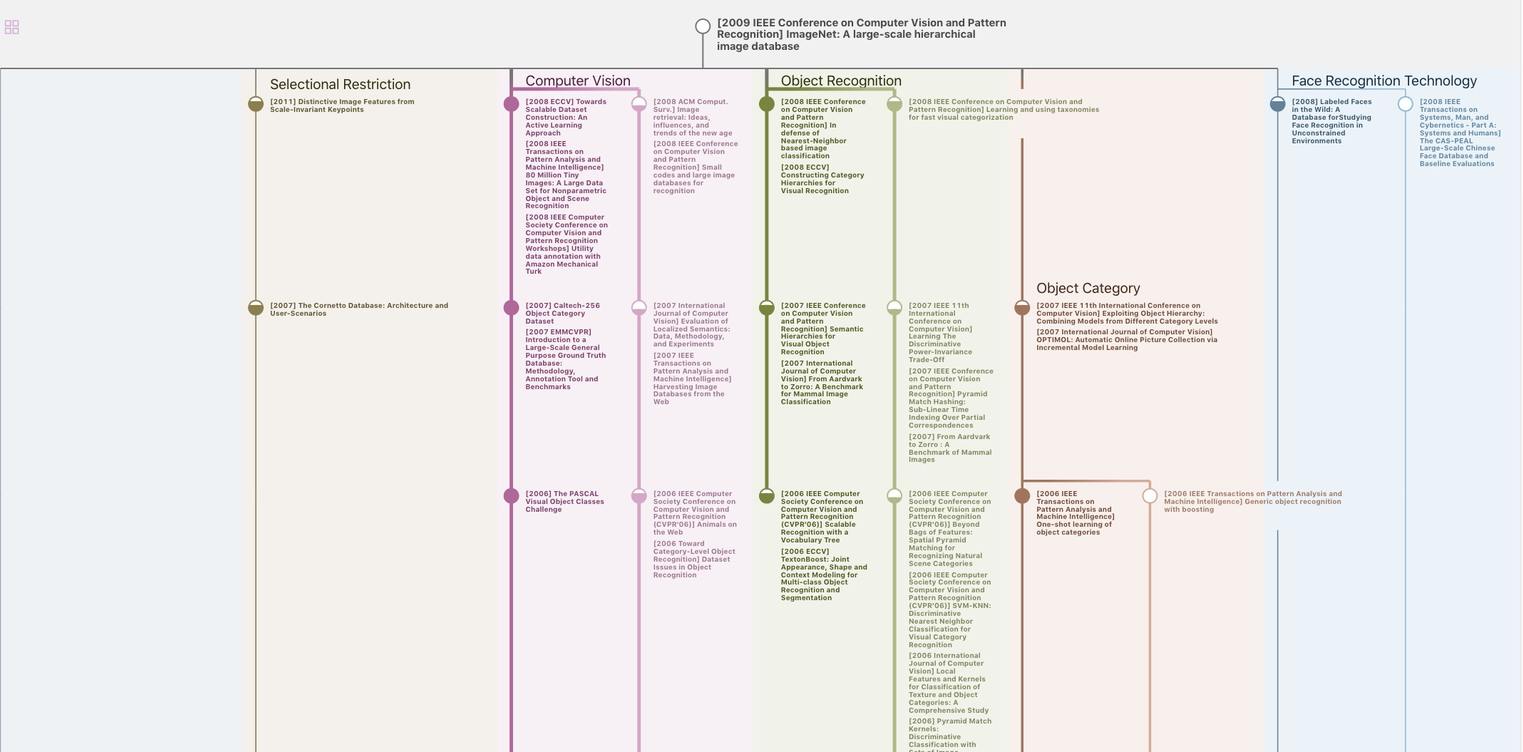
生成溯源树,研究论文发展脉络
Chat Paper
正在生成论文摘要