Rethinking Dice Loss for Medical Image Segmentation
2020 IEEE International Conference on Data Mining (ICDM)(2020)
摘要
Deep learning has proved to be a powerful tool for medical image analysis in recent years. Data imbalance is a common problem in medical images. Dice Loss is widely used in medical image segmentation tasks to address the data imbalance problem. However, it only addresses the imbalance problem between foreground and background yet overlooks another imbalance between easy and hard examples that also severely affects the training process of a learning model. Empirically speaking, an easy example generally contributes less to the overall loss than a hard example. However, in practice, compared with hard examples, a large number of easy examples will be generated from a medical image and will dominate the training model, resulting in sub-optimal training or worse. To tackle this problem, we propose a novel Focal Dice Loss to alleviate the imbalance between hard examples and easy examples. Focal Dice Loss is able to reduce the contribution from easy examples and make the model focus on hard examples through our proposed novel balanced sampling strategy during the training process. Furthermore, to evaluate the effectiveness of our proposed loss functions, we conduct extensive experiments on two real-world medical image datasets with 2D and 3D convolutional neural networks. The experimental results show that our proposed Focal Dice Loss brings a significant improvement in segmentation performance compared to Dice Loss. Moreover, we find that our proposed Focal Dice Loss can effectively alleviate the over-fitting problem.
更多查看译文
关键词
medical image segmentation, assisted medical diagnosis, data imbalance, Focal Dice Loss, over-fitting
AI 理解论文
溯源树
样例
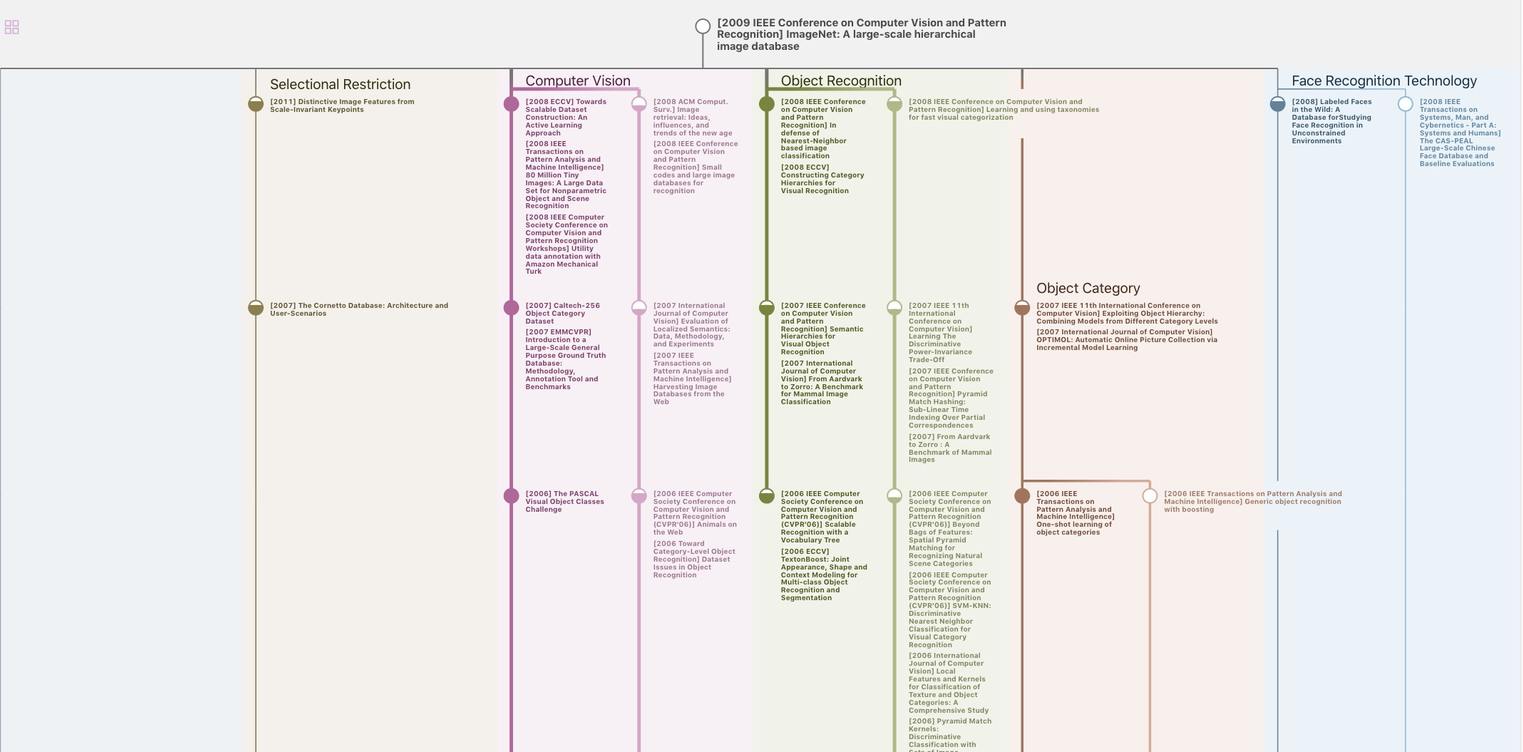
生成溯源树,研究论文发展脉络
Chat Paper
正在生成论文摘要