Robust Meta Network Embedding Against Adversarial Attacks
20TH IEEE INTERNATIONAL CONFERENCE ON DATA MINING (ICDM 2020)(2020)
摘要
Recent studies have shown that graph mining models are vulnerable to adversarial attacks. This paper proposes a robust meta network embedding framework, RoMNE, which improves the robustness of multiple network embedding on adversarial noisy networks while preserving the utility on original clean ones. First, we propose a generic meta learning based multiple network embedding model that can quickly adapt it to new embedding tasks on a variety of network data with only a small number of parameter and training updates. Second, Gumbel estimator and Gaussian smoothing techniques are introduced to implement differentiable approximation for optimizing non-differential objective of effective adversarial attacks. Last but not least, the adversarial attack and defense models are integrated into a dynamic adversarial training model. The competition of two models helps the latter be robust to adversarial attacks.
更多查看译文
关键词
Meta learning,multiple network embedding,adversarial attacks,dynamic adversarial training
AI 理解论文
溯源树
样例
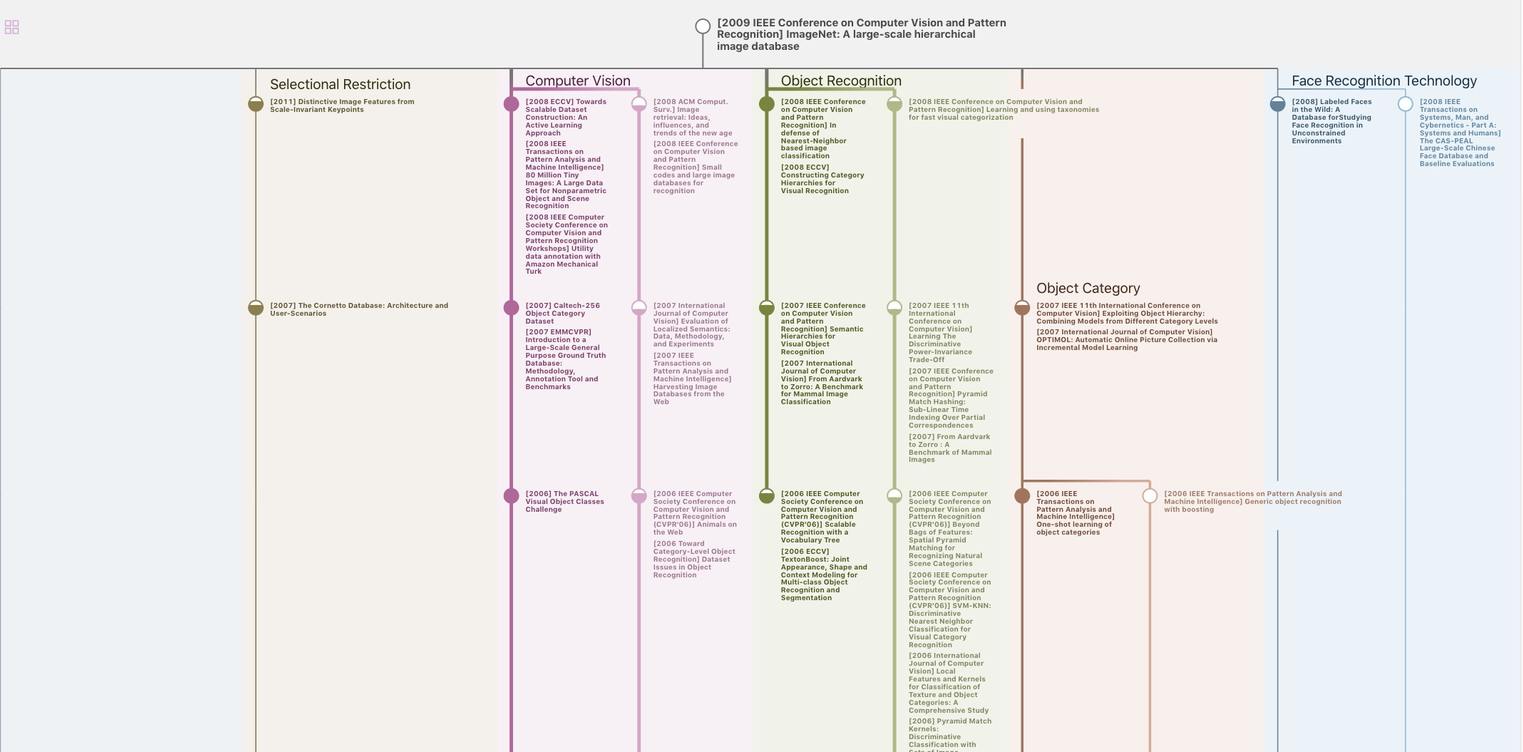
生成溯源树,研究论文发展脉络
Chat Paper
正在生成论文摘要