TDD Massive MISO Piloting Strategy With User Information: A Reinforcement Learning Approach
IEEE Wireless Communications Letters(2021)
摘要
In TDD massive MISO systems, user equipments (UEs) send channel measurement pilots to the BS for beamforming. Frequently sending these pilots, although improving beamforming, could consume significant communication resources. In this letter, we investigate how frequent these pilots should be sent for each UE so as to increase overall throughput performance for TDD massive MISO downlink. This real-time resource allocation problem is challenging due to non-trivial performance metric and boundary conditions. Assuming that instantaneous speed and location information of UEs can be obtained by the BS, we propose a reinforcement learning framework in which the BS acts as a learning agent to decide pilot intervals. Simulation results show that, for this multi-terminal setting where UEs compete for resources, using this centralized reinforcement learning framework, performance can be improved by choosing pilot intervals and transmission rates based on the UE information.
更多查看译文
关键词
Massive MISO,outdated CSI,pilot interval optimization,reinforcement learning,self-adaptive network
AI 理解论文
溯源树
样例
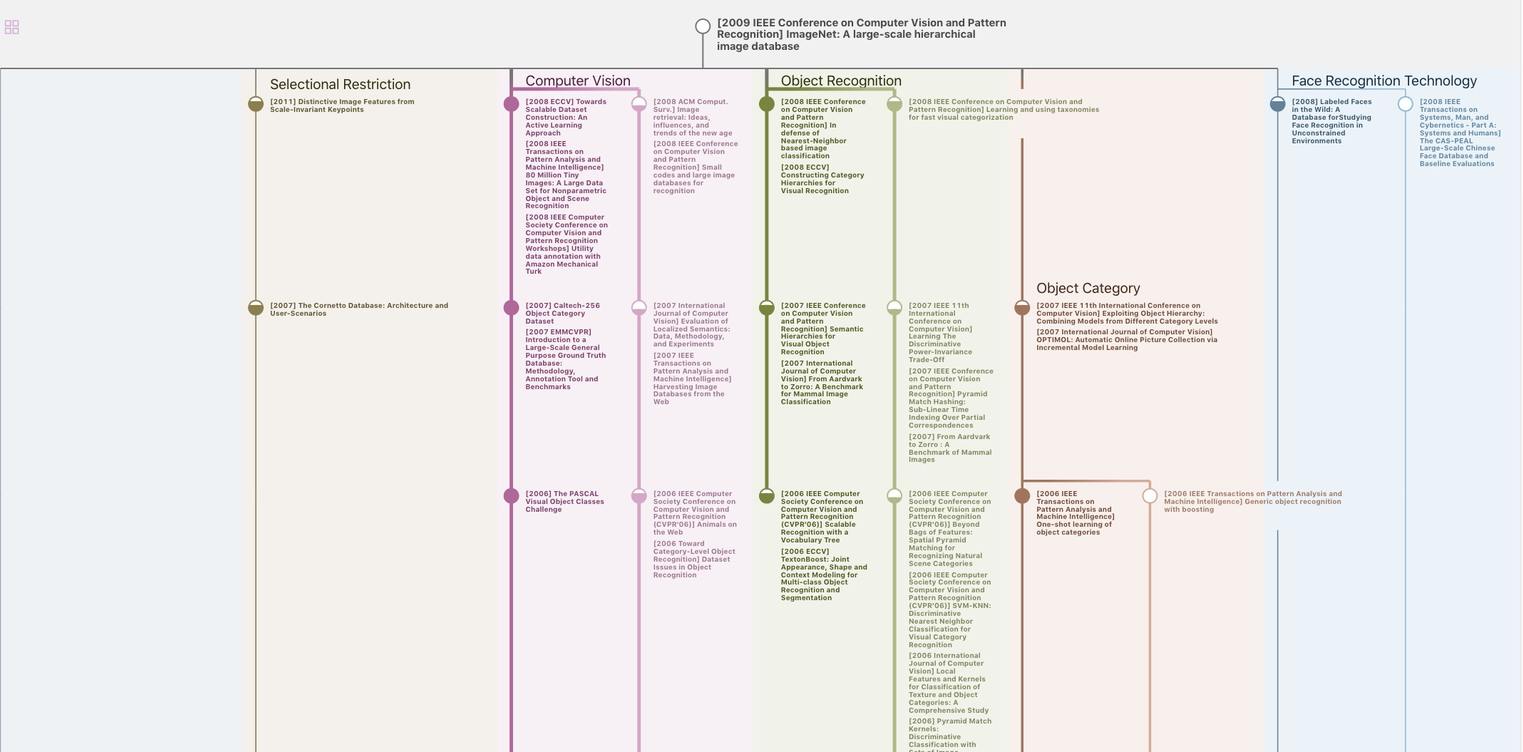
生成溯源树,研究论文发展脉络
Chat Paper
正在生成论文摘要