Edge Sparse Basis Network: A Deep Learning Framework for EEG Source Localization
2021 INTERNATIONAL JOINT CONFERENCE ON NEURAL NETWORKS (IJCNN)(2021)
摘要
EEG source localization is an important technical issue in EEG analysis. Despite many numerical methods existed for EEG source localization, they all rely on strong priors and the deep sources are intractable. Here we propose a deep learning framework using spatial basis function decomposition for EEG source localization. This framework combines the edge sparsity prior and Gaussian source basis, called Edge Sparse Basis Network (ESBN). The performance of ESBN is validated by both synthetic data and real EEG data during motor tasks. The results suggest that the supervised ESBN outperforms the traditional numerical methods in synthetic data and the unsupervised fine-tuning provides more focal and accurate localizations in real data. Our proposed deep learning framework can be extended to account for other source priors, and the real-time property of ESBN can facilitate the applications of EEG in brain-computer interfaces and clinics.
更多查看译文
关键词
EEG, Inverse problem, source localization, deep learning, edge sparsity
AI 理解论文
溯源树
样例
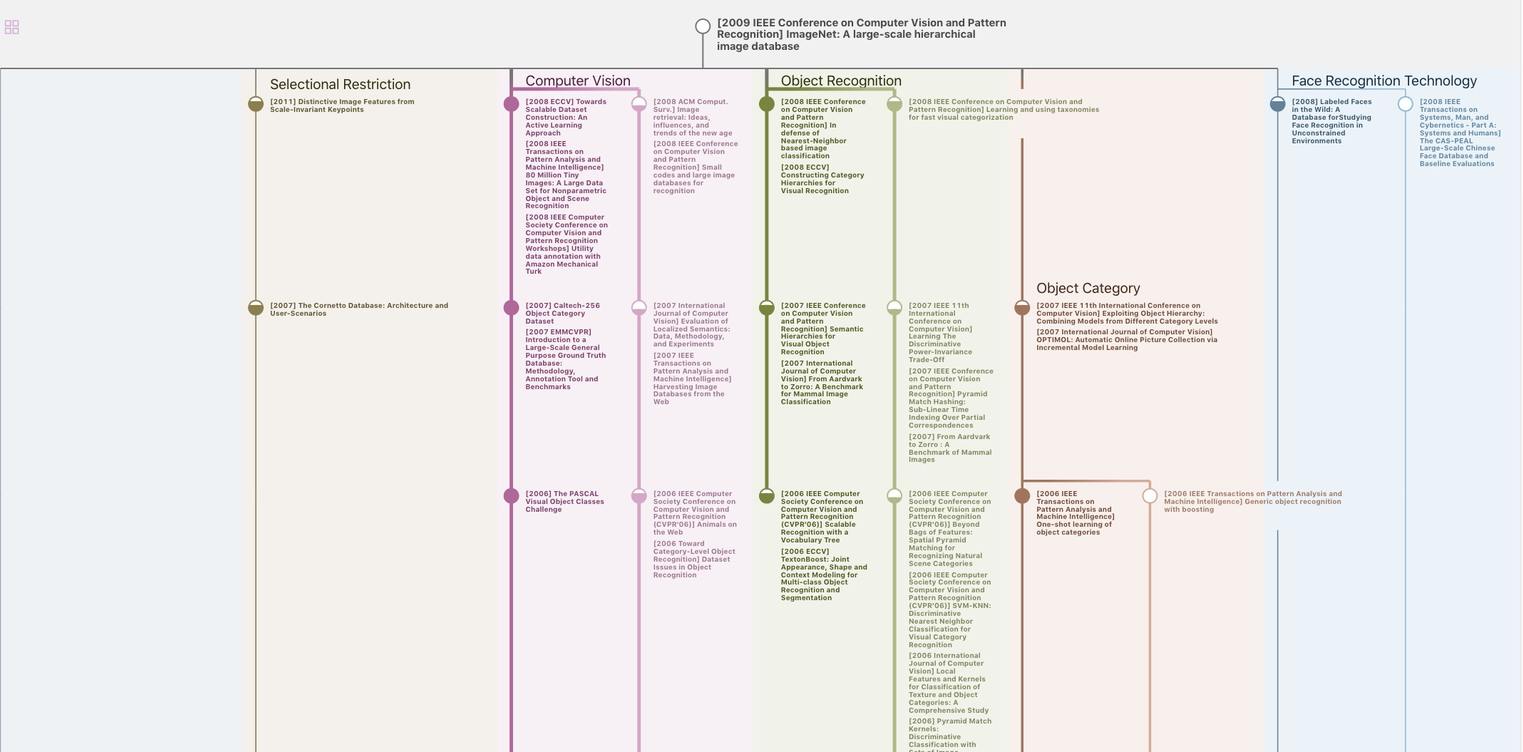
生成溯源树,研究论文发展脉络
Chat Paper
正在生成论文摘要