Conditional variance estimator for sufficient dimension reduction
BERNOULLI(2022)
摘要
Conditional Variance Estimation (CVE) is a novel sufficient dimension reduction (SDR) method for additive error regressions with continuous predictors and link function. It operates under the assumption that the predictors can be replaced by a lower dimensional projection without loss of information. Conditional Variance Estimation is fully data driven, does not require the restrictive linearity and constant variance conditions, and is not based on inverse regression as the majority of moment and likelihood based sufficient dimension reduction methods. CVE is shown to be consistent and its objective function to be uniformly convergent. CVE outperforms the mean average variance estimation, (MAVE), its main competitor, in several simulation settings, remains on par under others, while it always outperforms inverse regression based linear SDR methods, such as Sliced Inverse Regression.
更多查看译文
关键词
Regression, nonparametric, mean subspace, minimum average variance estimation, dimension, reduction
AI 理解论文
溯源树
样例
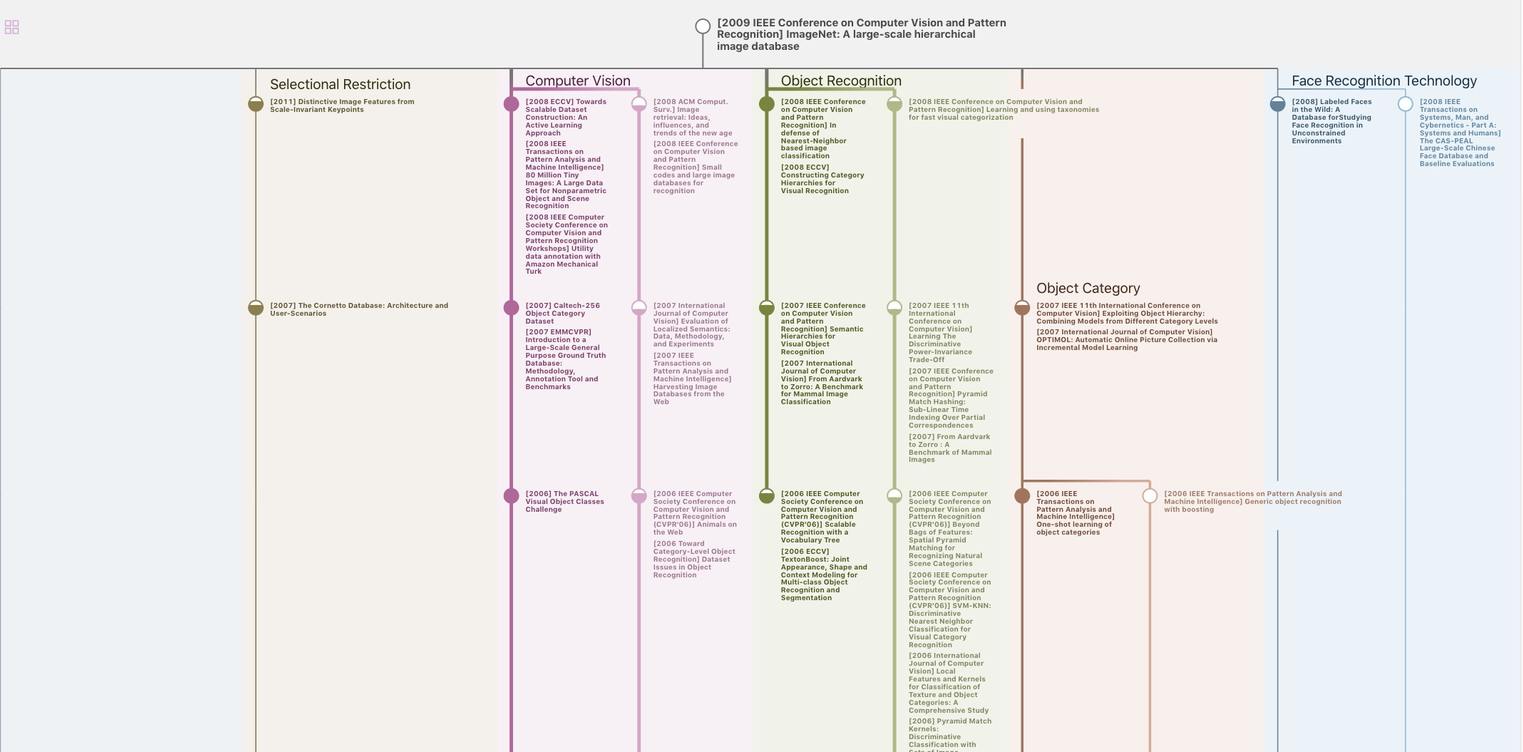
生成溯源树,研究论文发展脉络
Chat Paper
正在生成论文摘要