Learning Vision-Based Physics Intuition Models for Non-Disruptive Object Extraction.
IROS(2020)
摘要
Robots operating in human environments must be careful, when executing their manipulation skills, not to disturb nearby objects. This requires robots to reason about the effect of their manipulation choices by accounting for the support relationships among objects in the scene. Humans do this in part by visually assessing their surroundings and using physics intuition for how likely it is that a particular object can be safely manipulated (i.e., cause no disruption in the rest of the scene). Existing work has shown that deep convolutional neural networks can learn intuitive physics over images generated in simulation and determine the stability of a scene in the real world. In this paper, we extend these physics intuition models to the task of assessing safe object extraction by conditioning the visual images on specific objects in the scene. Our results, in both simulation and real-world settings, show that with our proposed method, physics intuition models can be used to inform a robot of which objects can be safely extracted and from which direction to extract them.
更多查看译文
关键词
physics intuition,cause no disruption,deep convolutional neural networks,intuitive physics,safe object extraction,visual images,vision-based physics intuition models,nondisruptive object extraction,human environments,manipulation skills,manipulation choices
AI 理解论文
溯源树
样例
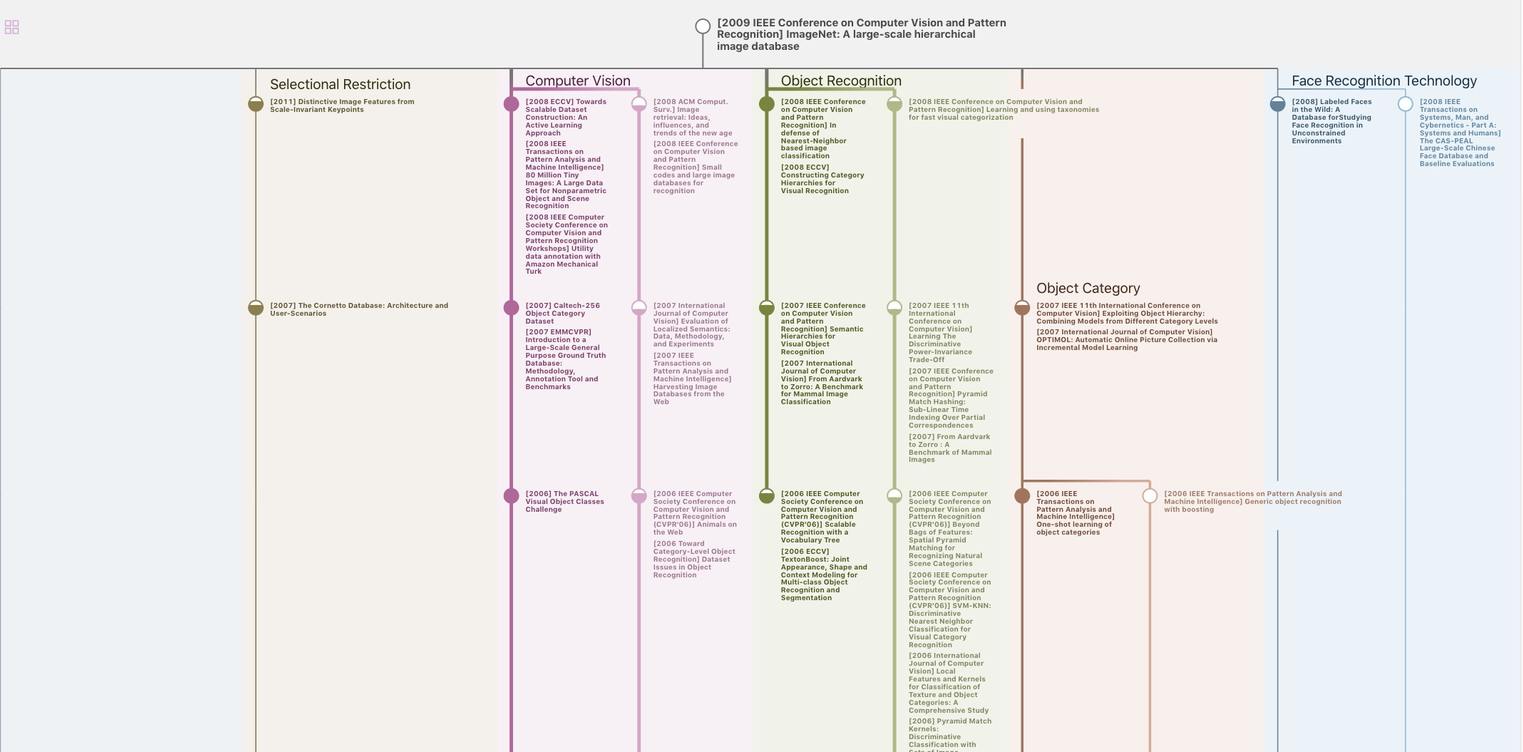
生成溯源树,研究论文发展脉络
Chat Paper
正在生成论文摘要