Reducing numerical work in non-linear parameter identification
arxiv(2022)
摘要
The real-life merit functions have an unimaginable complexity of an M-dimensional topography, where M is the number of the parameters. It is shown that there is an underlying noise-free merit function, called follower merit function which can be constructed from simulated, noise-free data using the solution of a Least Squares minimization. The difference of these is controlled by the norm of the error vector. The local minima arisen from the noise can be skipped during such minimization that apply adjustable, large step sizes, depending on the norm of the error vector. The suggested hierarchical minimisation - based on the implicit function of a projection map - makes the minimisation in two steps. In the first step some parameters are eliminated with conditional minimisation, resulting in a kind of deepest section of the merit function, called clever section with respect to the remainder parameters. Then the clever section is minimized. The method can be used to handle a quasi-degenerate global minimum, in sensitivity analyses, reliability testing.
更多查看译文
关键词
parameter,numerical work,identification,non-linear
AI 理解论文
溯源树
样例
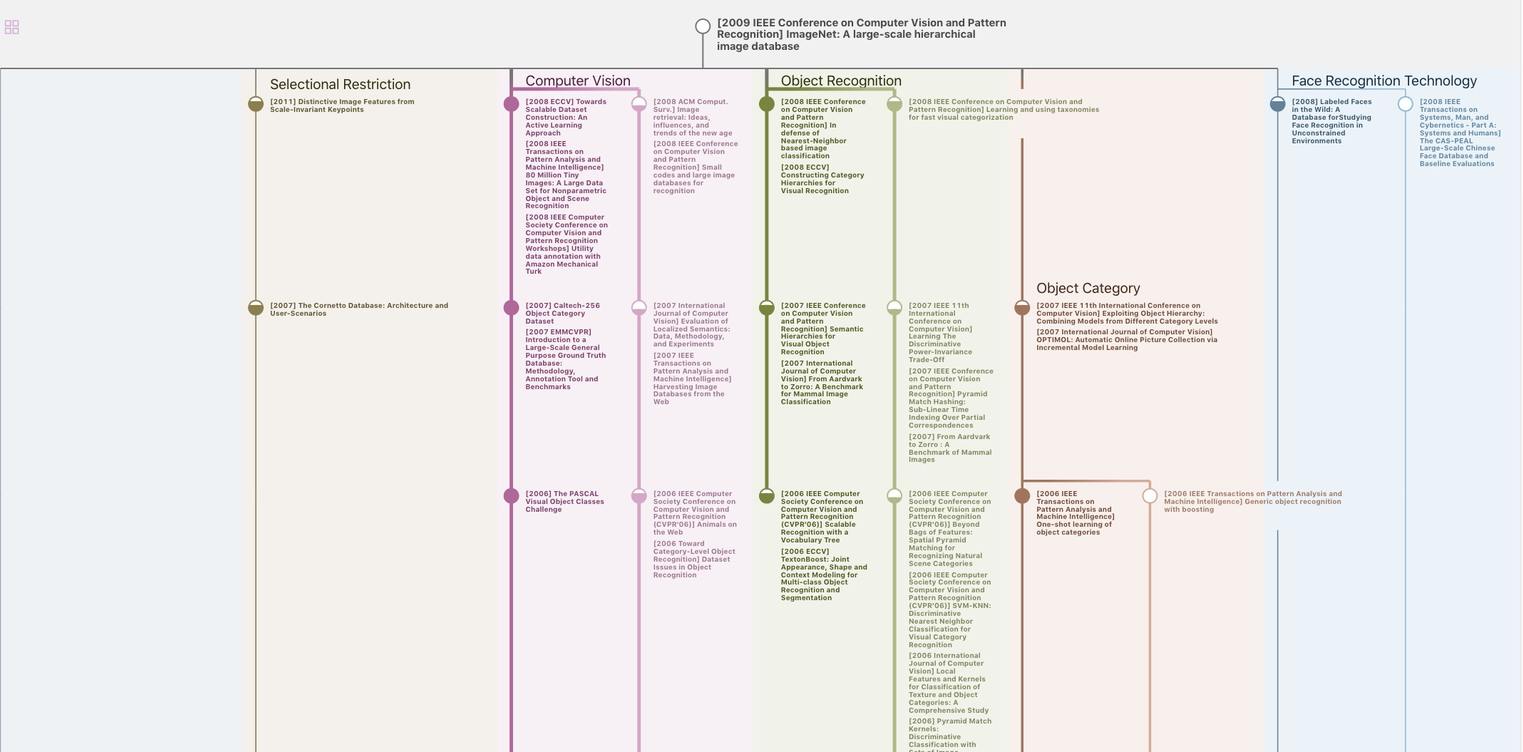
生成溯源树,研究论文发展脉络
Chat Paper
正在生成论文摘要