Tight risk bound for high dimensional time series completion
ELECTRONIC JOURNAL OF STATISTICS(2022)
摘要
Initially designed for independent datas, low-rank matrix completion was successfully applied in many domains to the reconstruction of partially observed high-dimensional time series. However, there is a lack of theory to support the application of these methods to dependent datas. In this paper, we propose a general model for multivariate, partially observed time series. We show that the least-square method with a rank penalty leads to reconstruction error of the same order as for independent datas. Moreover, when the time series has some additional properties such as periodicity or smoothness, the rate can actually be faster than in the independent case.
更多查看译文
关键词
Matrix completion, multivariate time series analysis, matrix factorization, high-dimensional time series, concentration inequalities, mixing
AI 理解论文
溯源树
样例
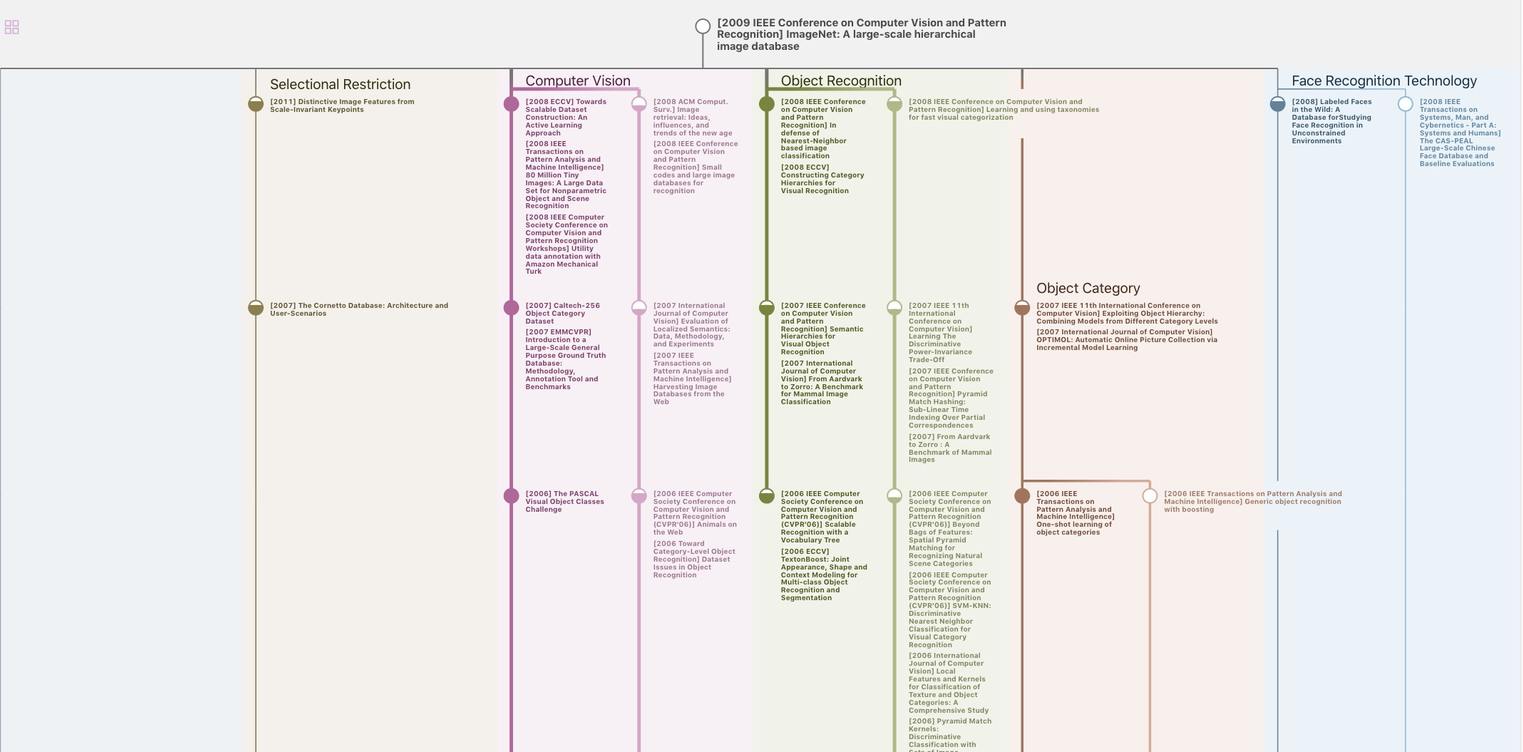
生成溯源树,研究论文发展脉络
Chat Paper
正在生成论文摘要