Differentiable Particle Filtering Via Entropy-Regularized Optimal Transport
INTERNATIONAL CONFERENCE ON MACHINE LEARNING, VOL 139(2021)
摘要
Particle Filtering (PF) methods are an established class of procedures for performing inference in non-linear state-space models. Resampling is a key ingredient of PF, necessary to obtain low variance likelihood and states estimates. However, traditional resampling methods result in PF-based loss functions being non-differentiable with respect to model and PF parameters. In a variational inference context, resampling also yields high variance gradient estimates of the PF-based evidence lower bound. By leveraging optimal transport ideas, we introduce a principled differentiable particle filter and provide convergence results. We demonstrate this novel method on a variety of applications.
更多查看译文
关键词
differentiable particle filtering,transport,optimal
AI 理解论文
溯源树
样例
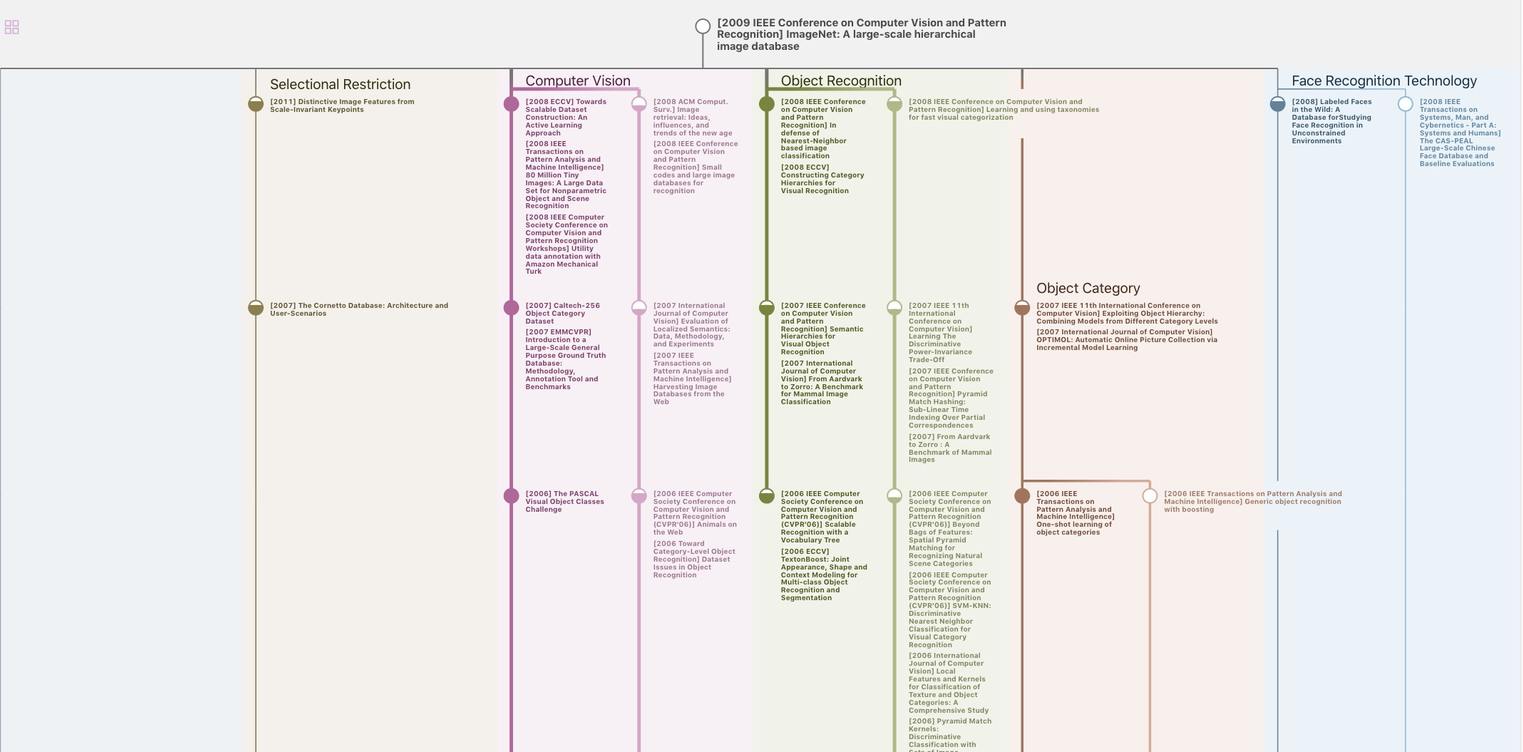
生成溯源树,研究论文发展脉络
Chat Paper
正在生成论文摘要