A Deep Adversarial Model for Suffix and Remaining Time Prediction of Event Sequences.
SDM(2021)
摘要
Event suffix and remaining time prediction are sequence to sequence learning tasks. They have wide applications in different areas such as economics, digital health, business process management and IT infrastructure monitoring. Timestamped event sequences contain ordered events which carry at least two attributes: the event's label and its timestamp. Suffix and remaining time prediction are about obtaining the most likely continuation of event labels and the remaining time until the sequence finishes, respectively. Recent deep learning-based works for such predictions are prone to potentially large prediction errors because of closed-loop training (i.e., the next event is conditioned on the ground truth of previous events) and open-loop inference (i.e., the next event is conditioned on previously predicted events). In this work, we propose an encoder-decoder architecture for open-loop training to advance the suffix and remaining time prediction of event sequences. To capture the joint temporal dynamics of events, we harness the power of adversarial learning techniques to boost prediction performance. We consider four real-life datasets and three baselines in our experiments. The results show improvements up to four times compared to the state of the art in suffix and remaining time prediction of event sequences, specifically in the realm of business process executions. We also show that the obtained improvements of adversarial training are superior compared to standard training under the same experimental setup.
更多查看译文
关键词
deep adversarial model,remaining time prediction,suffix,event
AI 理解论文
溯源树
样例
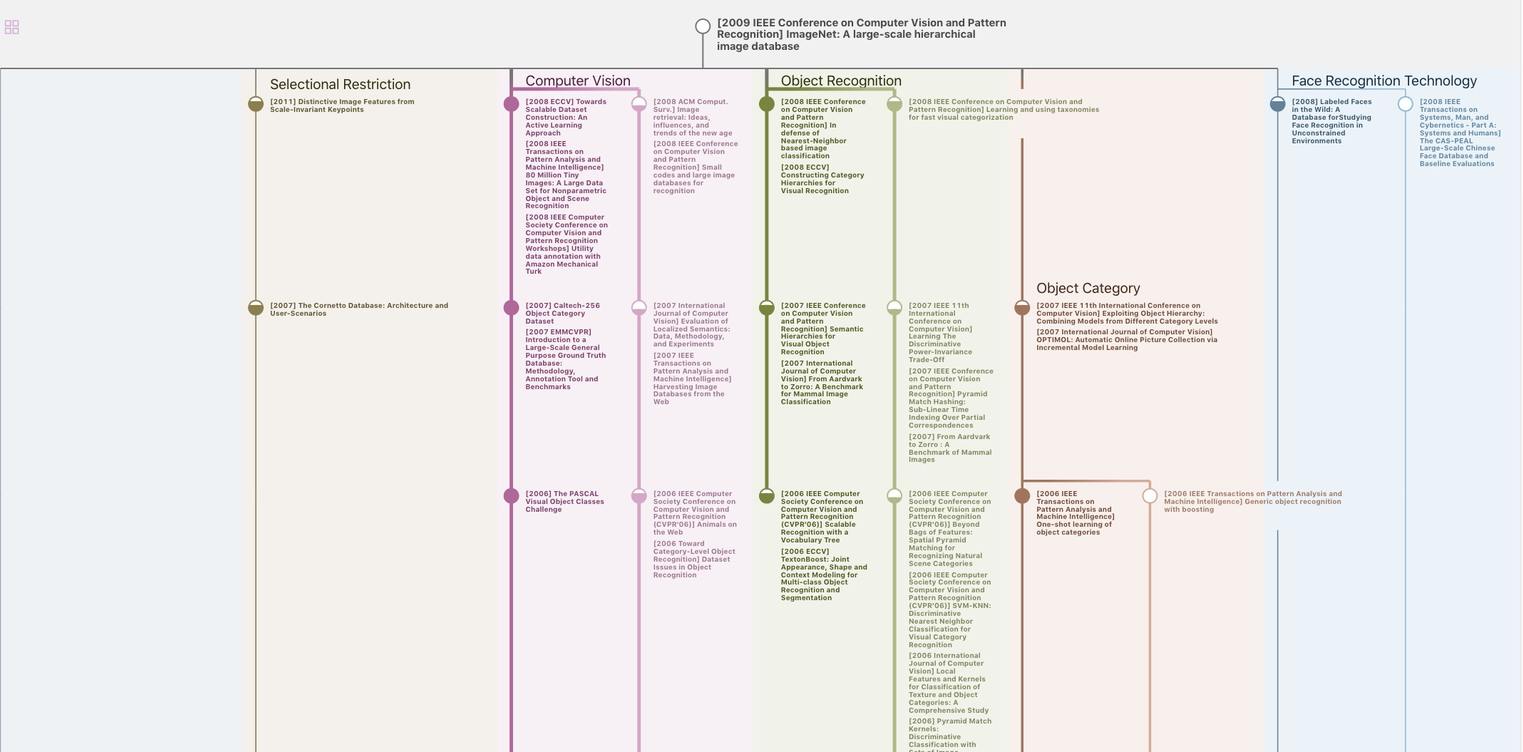
生成溯源树,研究论文发展脉络
Chat Paper
正在生成论文摘要