A Benchmark for the Bayesian Inversion of Coefficients in Partial Differential Equations
SIAM REVIEW(2023)
摘要
Bayesian methods have been widely used in the last two decades to infer statistical properties of spatially variable coefficients in partial differential equations from measurements of the solutions of these equations. Yet, in many cases the number of variables used to parameterize these coefficients is large, and oobtaining meaningful statistics of their probability distributions is difficult using simple sampling methods such as the basic Metropolis-Hastings algorithm-in particular, if the inverse problem is ill-conditioned or ill-posed. As a consequence, many advanced sampling methods have been described in the literature that converge faster than Metropolis-Hastings, for example, by exploiting hierarchies of statistical models or hierarchies of discretizations of the underlying differential equation. At the same time, it remains difficult for the reader of the literature to quantify the advantages of these algorithms because there is no commonly used benchmark. This paper presents a benchmark Bayesian inverse problem-namely, the determination of a spatially variable coefficient, discretized by 64 values, in a Poisson equation, based on point measurements of the solution-that fills the gap between widely used simple test cases (such as superpositions of Gaussians) and real applications that are difficult to replicate for developers of sampling algorithms. We provide a complete description of the test case and provide an open-source implementation that can serve as the basis for further experiments. We have also computed 2 \times 1011 samples, at a cost of some 30 CPU years, of the posterior probability distribution from which we have generated detailed and accurate statistics against which other sampling algorithms can be tested.
更多查看译文
关键词
Bayesian inference,inverse problems,partial differential equations,Markov chain Monte,Carlo,benchmarking
AI 理解论文
溯源树
样例
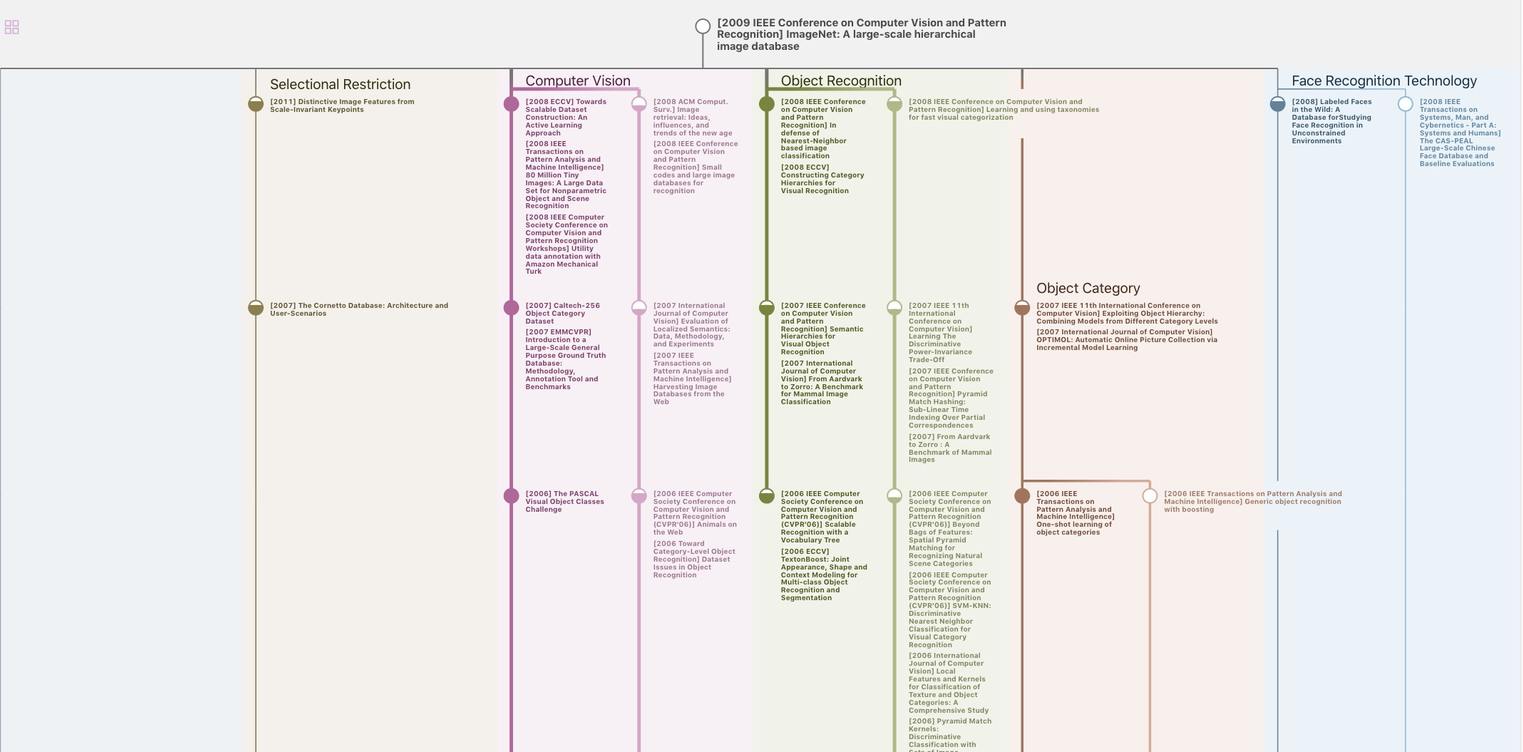
生成溯源树,研究论文发展脉络
Chat Paper
正在生成论文摘要