Knowledge Graph Embedding using Graph Convolutional Networks with Relation-Aware Attention
arxiv(2021)
摘要
Knowledge graph embedding methods learn embeddings of entities and relations in a low dimensional space which can be used for various downstream machine learning tasks such as link prediction and entity matching. Various graph convolutional network methods have been proposed which use different types of information to learn the features of entities and relations. However, these methods assign the same weight (importance) to the neighbors when aggregating the information, ignoring the role of different relations with the neighboring entities. To this end, we propose a relation-aware graph attention model that leverages relation information to compute different weights to the neighboring nodes for learning embeddings of entities and relations. We evaluate our proposed approach on link prediction and entity matching tasks. Our experimental results on link prediction on three datasets (one proprietary and two public) and results on unsupervised entity matching on one proprietary dataset demonstrate the effectiveness of the relation-aware attention.
更多查看译文
关键词
graph convolutional networks,knowledge,attention,relation-aware
AI 理解论文
溯源树
样例
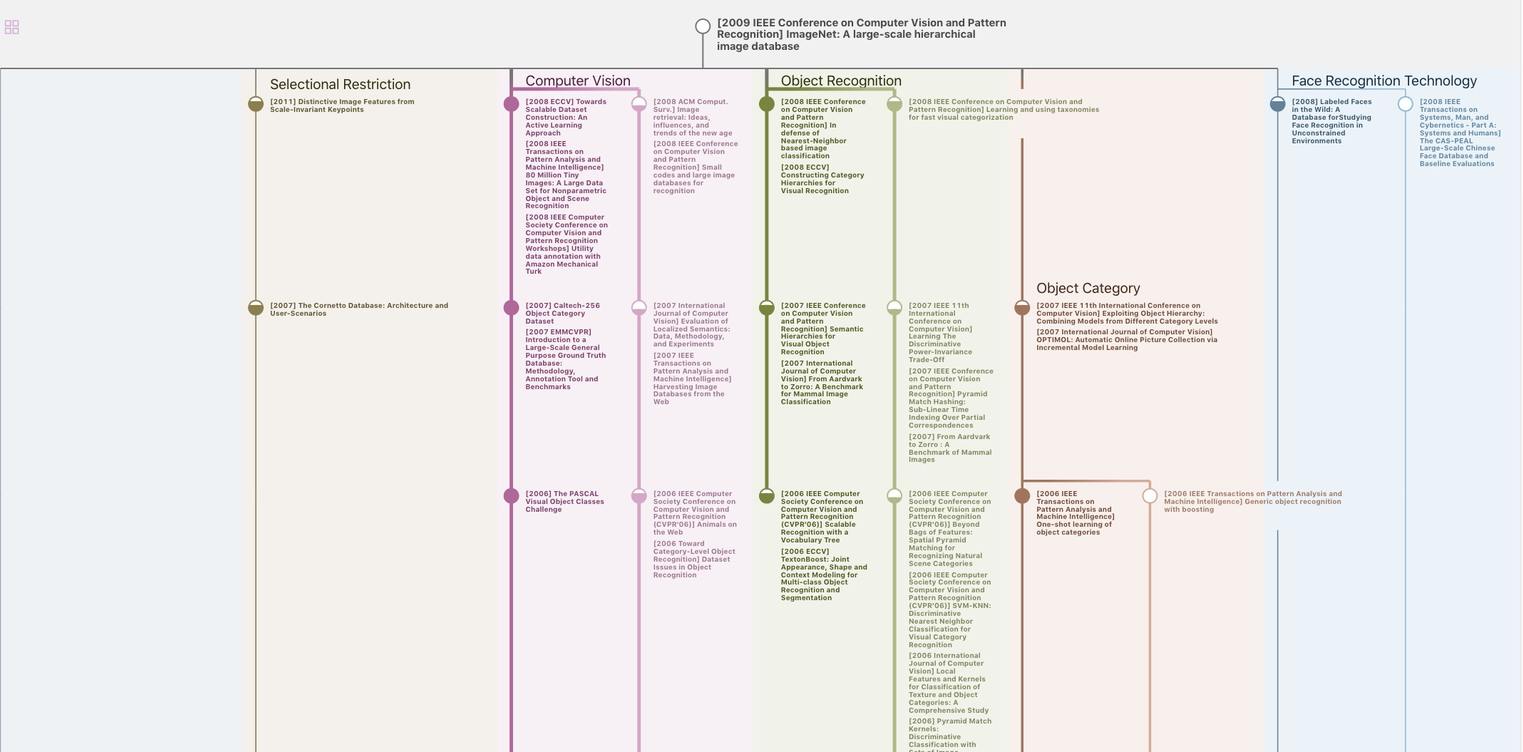
生成溯源树,研究论文发展脉络
Chat Paper
正在生成论文摘要