Kernint: A Kernel Framework For Integrating Supervised And Unsupervised Analyses In Spatio-Temporal Metagenomic Datasets
FRONTIERS IN MICROBIOLOGY(2021)
摘要
The advent of next-generation sequencing technologies allowed relative quantification of microbiome communities and their spatial and temporal variation. In recent years, supervised learning (i.e., prediction of a phenotype of interest) from taxonomic abundances has become increasingly common in the microbiome field. However, a gap exists between supervised and classical unsupervised analyses, based on computing ecological dissimilarities for visualization or clustering. Despite this, both approaches face common challenges, like the compositional nature of next-generation sequencing data or the integration of the spatial and temporal dimensions. Here we propose a kernel framework to place on a common ground the unsupervised and supervised microbiome analyses, including the retrieval of microbial signatures (taxa importances). We define two compositional kernels (Aitchison-RBF and compositional linear) and discuss how to transform non-compositional beta-dissimilarity measures into kernels. Spatial data is integrated with multiple kernel learning, while longitudinal data is evaluated by specific kernels. We illustrate our framework through a single point soil dataset, a human dataset with a spatial component, and a previously unpublished longitudinal dataset concerning pig production. The proposed framework and the case studies are freely available in the kernInt package at https://github.com/elies-ramon/kernInt.
更多查看译文
关键词
microbiome, metagenomics, kernel, supervised, unsupervised, spatio-temporal, SVM, kPCA
AI 理解论文
溯源树
样例
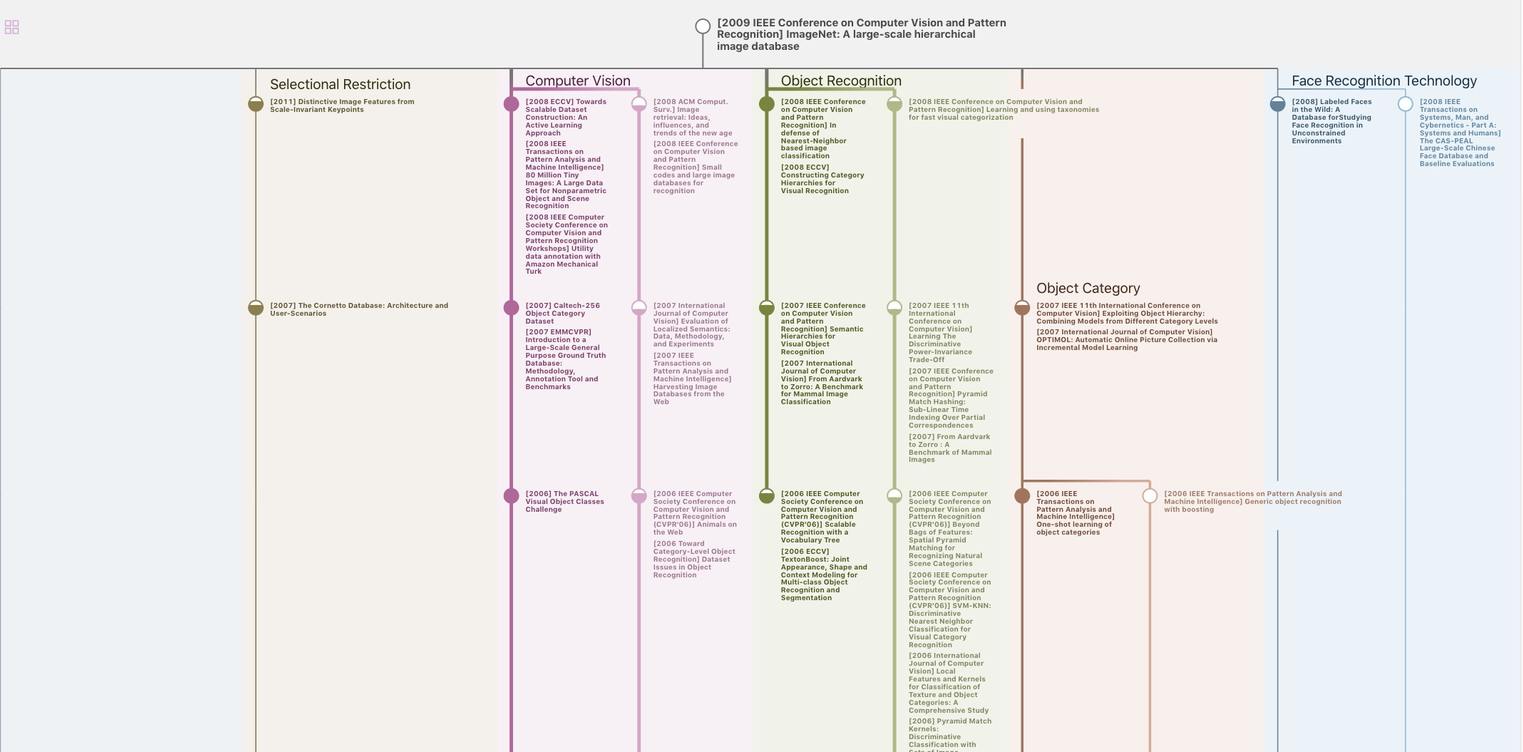
生成溯源树,研究论文发展脉络
Chat Paper
正在生成论文摘要