DO AS I MEAN, NOT AS I SAY: SEQUENCE LOSS TRAINING FOR SPOKEN LANGUAGE UNDERSTANDING
2021 IEEE INTERNATIONAL CONFERENCE ON ACOUSTICS, SPEECH AND SIGNAL PROCESSING (ICASSP 2021)(2021)
摘要
Spoken language understanding (SLU) systems extract transcriptions, as well as semantics of intent or named entities from speech, and are essential components of voice activated systems. SLU models, which either directly extract semantics from audio or are composed of pipelined automatic speech recognition (ASR) and natural language understanding (NLU) models, are typically trained via differentiable cross-entropy losses, even when the relevant performance metrics of interest are word or semantic error rates. In this work, we propose non-differentiable sequence losses based on SLU metrics as a proxy for semantic error and use the REINFORCE trick to train ASR and SLU models with this loss. We show that custom sequence loss training is the state-of-the-art on open SLU datasets and leads to 6% relative improvement in both ASR and NLU performance metrics on large proprietary datasets. We also demonstrate how the semantic sequence loss training paradigm can be used to update ASR and SLU models without transcripts, using semantic feedback alone.
更多查看译文
关键词
speech recognition, spoken language understanding, REINFORCE, multitask training, neural interfaces
AI 理解论文
溯源树
样例
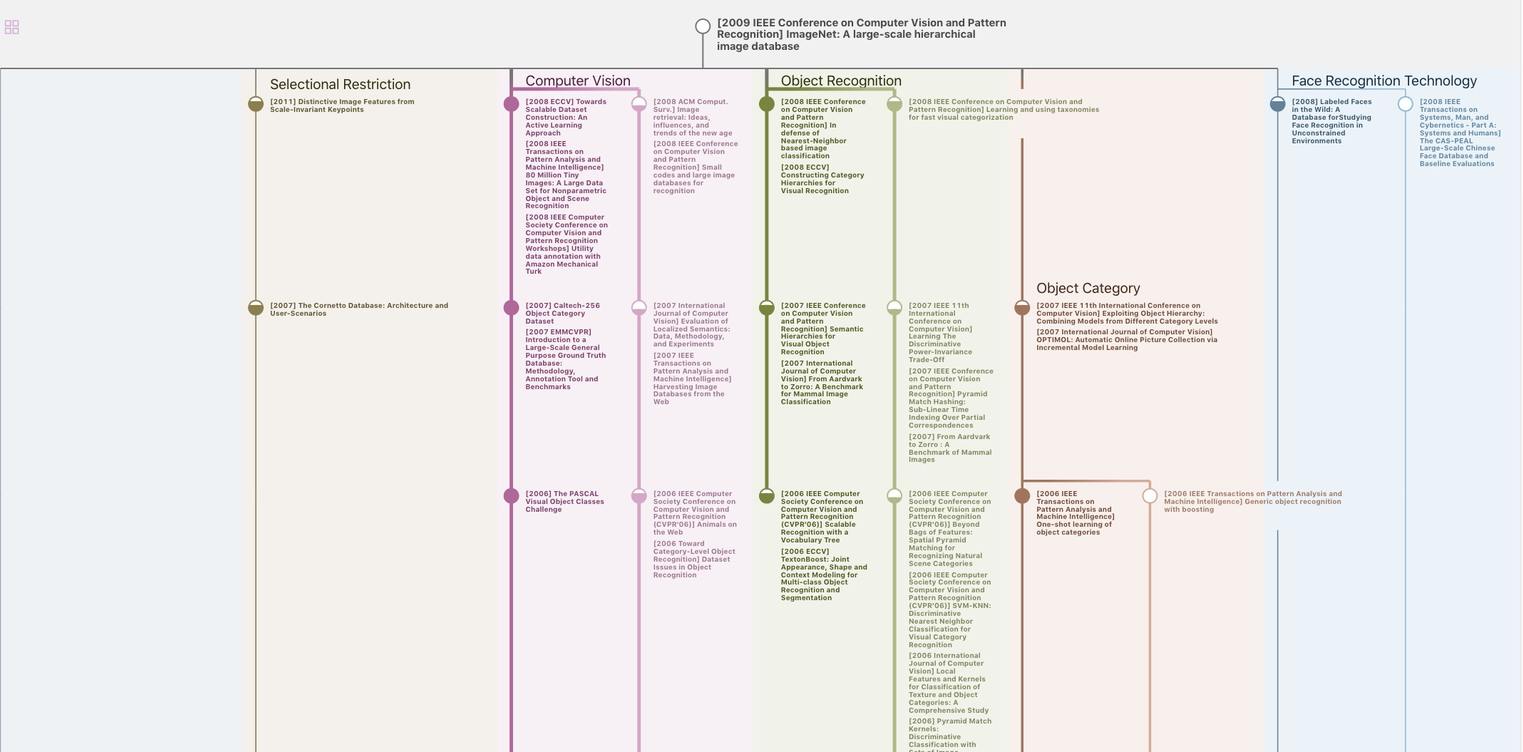
生成溯源树,研究论文发展脉络
Chat Paper
正在生成论文摘要