CONTENT-AWARE SPEAKER EMBEDDINGS FOR SPEAKER DIARISATION
2021 IEEE INTERNATIONAL CONFERENCE ON ACOUSTICS, SPEECH AND SIGNAL PROCESSING (ICASSP 2021)(2021)
Abstract
Recent speaker diarisation systems often convert variable length speech segments into fixed-length vector representations for speaker clustering, which are known as speaker embeddings. In this paper, the content-aware speaker embeddings (CASE) approach is proposed, which extends the input of the speaker classifier to include not only acoustic features but also their corresponding speech content, via phone, character, and word embeddings. Compared to alternative methods that leverage similar information, such as multitask or adversarial training, CASE factorises automatic speech recognition (ASR) from speaker recognition to focus on modelling speaker characteristics and correlations with the corresponding content units to derive more expressive representations. CASE is evaluated for speaker re-clustering with a realistic speaker diarisation setup using the AMI meeting transcription dataset, where the content information is obtained by performing ASR based on an automatic segmentation. Experimental results showed that CASE achieved a 17.8% relative speaker error rate reduction over conventional methods.
MoreTranslated text
Key words
content-aware speaker embedding, diarisation, d-vector, speech recognition, distributed representation
AI Read Science
Must-Reading Tree
Example
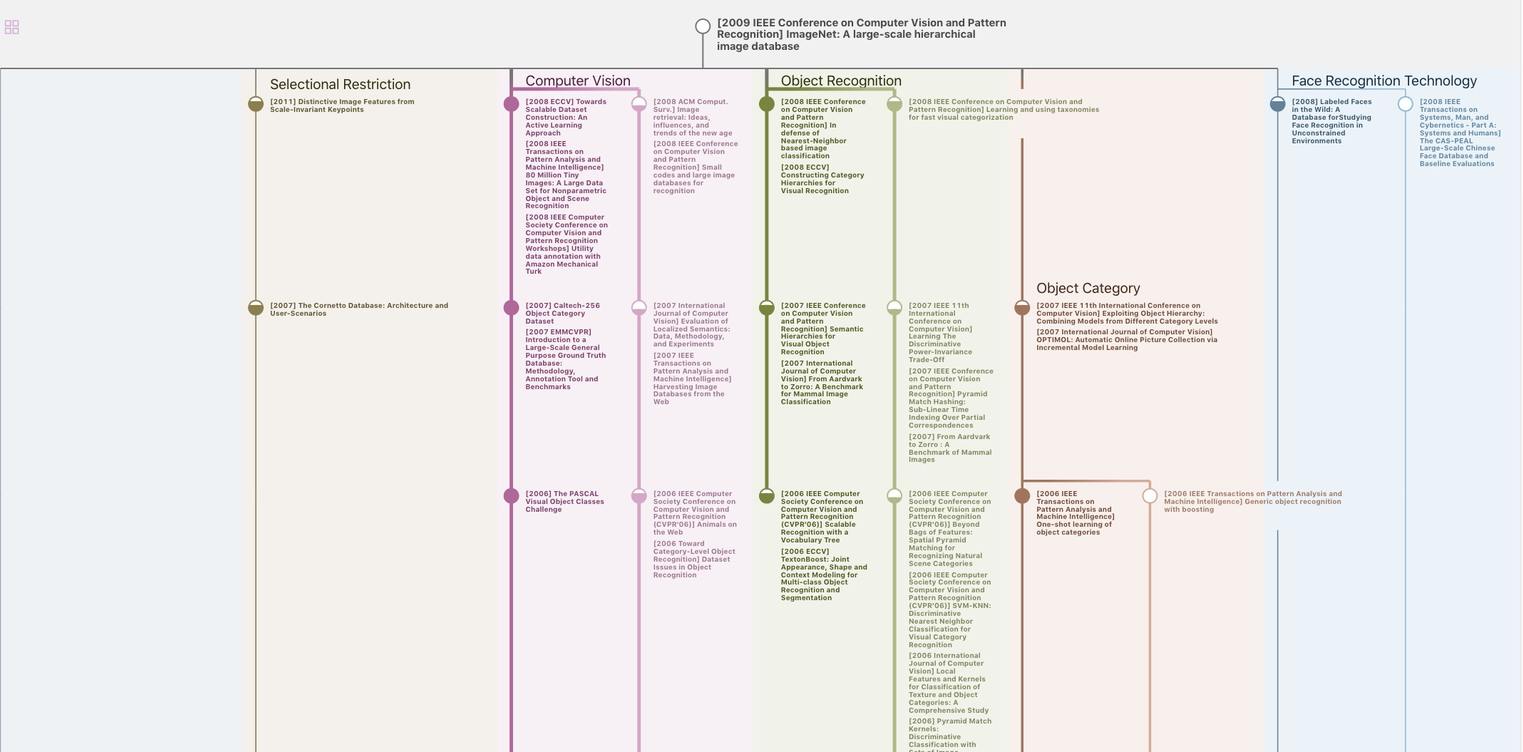
Generate MRT to find the research sequence of this paper
Chat Paper
Summary is being generated by the instructions you defined