AN INVESTIGATION OF END-TO-END MODELS FOR ROBUST SPEECH RECOGNITION
2021 IEEE INTERNATIONAL CONFERENCE ON ACOUSTICS, SPEECH AND SIGNAL PROCESSING (ICASSP 2021)(2021)
摘要
End-to-end models for robust automatic speech recognition (ASR) have not been sufficiently well-explored in prior work. With end-to-end models, one could choose to preprocess the input speech using speech enhancement techniques and train the model using enhanced speech. Another alternative is to pass the noisy speech as input and modify the model architecture to adapt to noisy speech. A systematic comparison of these two approaches for end-to-end robust ASR has not been attempted before. We address this gap and present a detailed comparison of speech enhancement-based techniques and three different model-based adaptation techniques covering data augmentation, multi-task learning, and adversarial learning for robust ASR. While adversarial learning is the best-performing technique on certain noise types, it comes at the cost of degrading clean speech WER. On other relatively stationary noise types, a new speech enhancement technique outperformed all the model-based adaptation techniques. This suggests that knowledge of the underlying noise type can meaningfully inform the choice of adaptation technique.
更多查看译文
关键词
Robust ASR, Speech Enhancement, Multi-task and Adversarial Learning, Data Augmentation
AI 理解论文
溯源树
样例
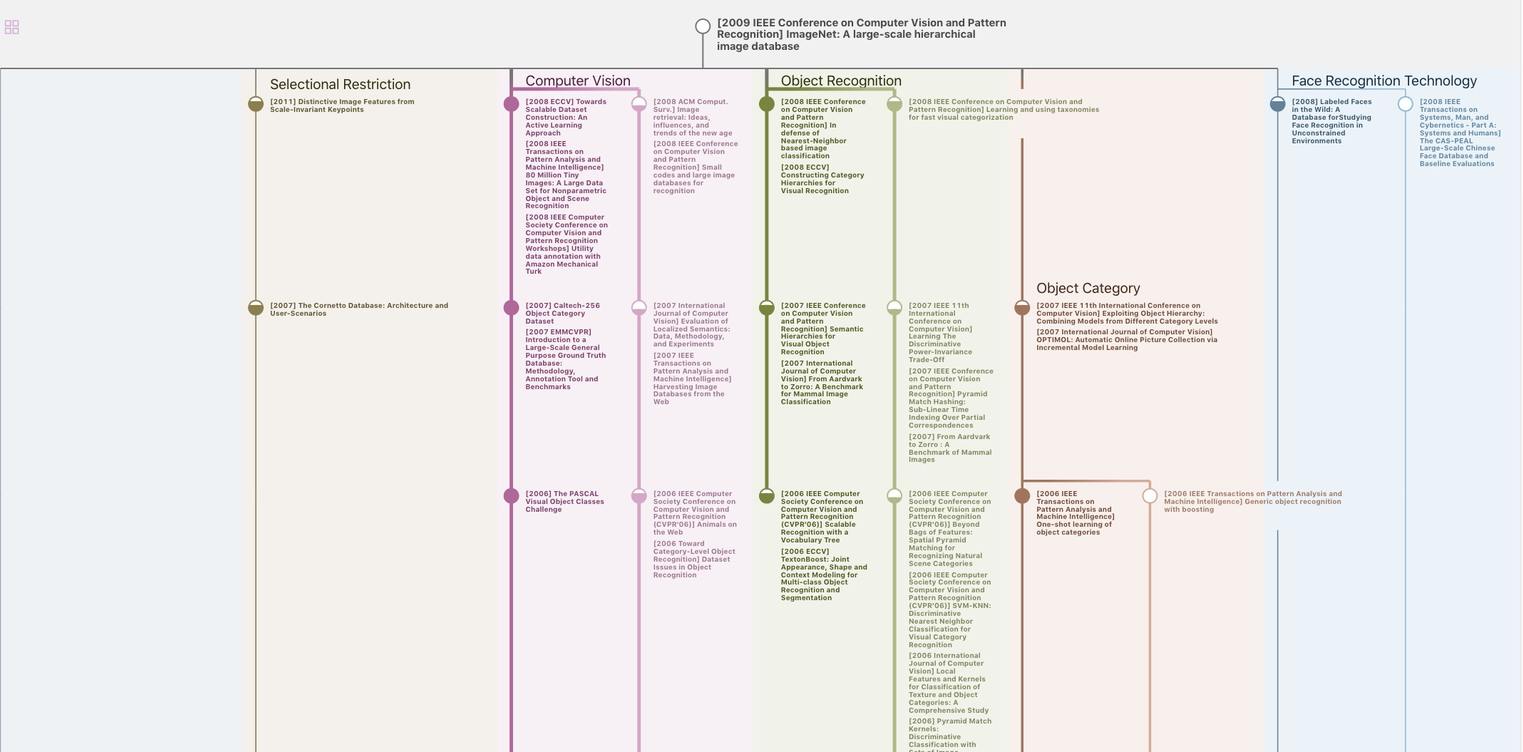
生成溯源树,研究论文发展脉络
Chat Paper
正在生成论文摘要