Superresolving Herschel Imaging: A Proof Of Concept Using Deep Neural Networks
MONTHLY NOTICES OF THE ROYAL ASTRONOMICAL SOCIETY(2021)
摘要
Wide-field submillimetre surveys have driven many major advances in galaxy evolution in the past decade, but without extensive follow-up observations the coarse angular resolution of these surveys limits the science exploitation. This has driven the development of various analytical deconvolution methods. In the last half a decade Generative Adversarial Networks have been used to attempt deconvolutions on optical data. Here, we present an auto-encoder with a novel loss function to overcome this problem in the submillimeter wavelength range. This approach is successfully demonstrated on Herschel SPIRE 500 mu m COSMOS data, with the superresolving target being the JCMT SCUBA-2 450 mu m observations of the same field. We reproduce the JCMT SCUBA-2 images with high fidelity using this auto-encoder. This is quantified through the point source fluxes and positions, the completeness, and the purity.
更多查看译文
关键词
methods: data analysis, submillimetre: galaxies
AI 理解论文
溯源树
样例
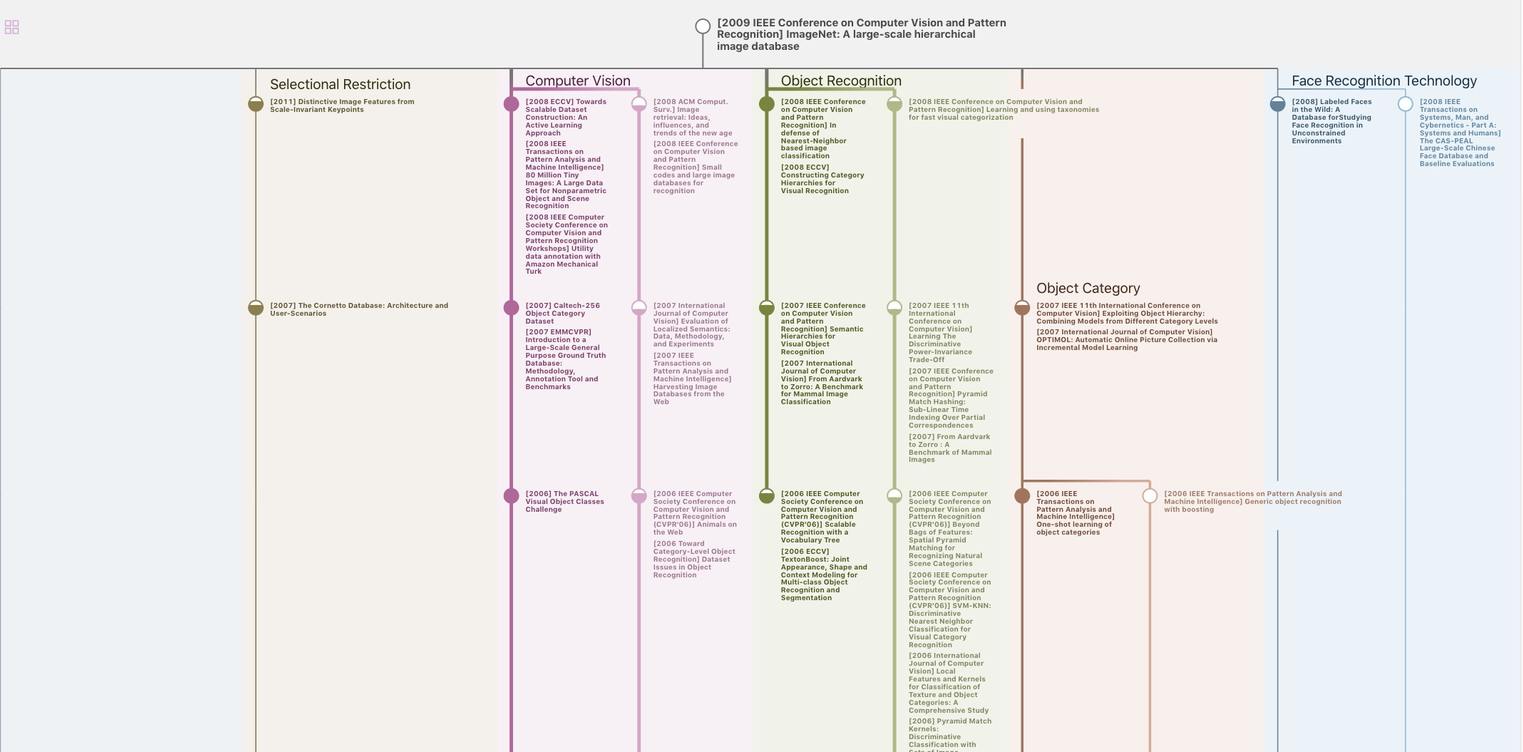
生成溯源树,研究论文发展脉络
Chat Paper
正在生成论文摘要