Context-adaptive Gaussian Attention for Text-independent Speaker Verification.
APSIPA(2020)
摘要
Multi-head attention (MHA) has shown its effectiveness on aggregating frame-level features for speaker verification task. However, MHA weights each frame individually without considering context information which is important for modeling speaker characteristics of the speech. Based on the assumption that the highly relevant context information should follow a temporal Gaussian distribution, we propose a novel variant of multi-head attention, named as context-adaptive Gaussian attention (CGA), which employs a set of Gaussian functions with different parameters to dynamically model the distributions of the weights obtained from each head. Furthermore, a Gaussian Clustering algorithm (GC) is designed to merge the overlapped Gaussian distributions between different heads. In this way, the proposed method can facilitate the model to better capture multi-span context information compared to the traditional multi-head attention. Experiments on Voxceleb1 dataset demonstrate that the proposed CGA outperforms the state-of-the-art pooling approaches.
更多查看译文
关键词
context-adaptive Gaussian attention,text-independent speaker verification,frame-level features,speaker verification task,MHA weights,speaker characteristics,highly relevant context information,temporal Gaussian distribution,Gaussian functions,Gaussian Clustering algorithm,overlapped Gaussian distributions,different heads,capture multispan context information,traditional multihead attention
AI 理解论文
溯源树
样例
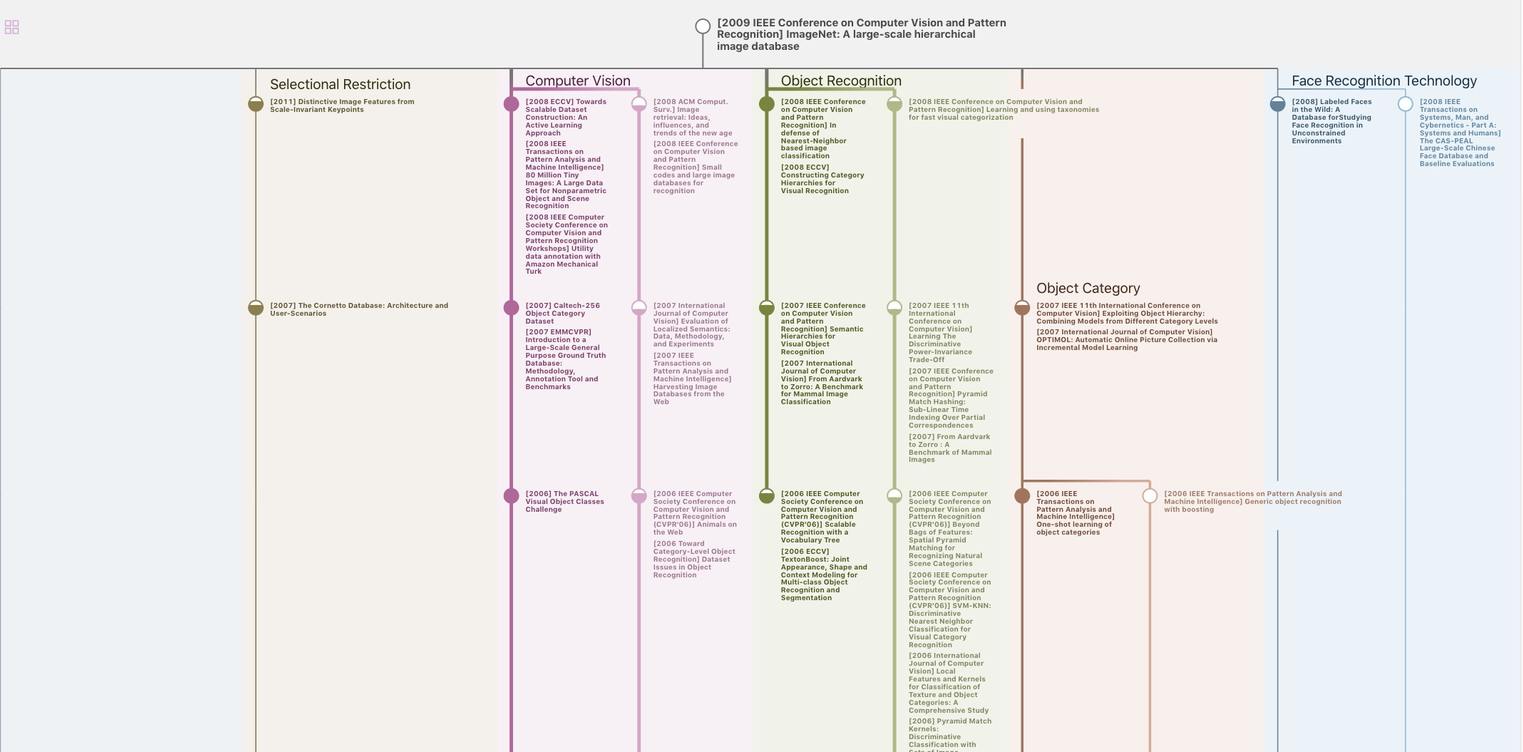
生成溯源树,研究论文发展脉络
Chat Paper
正在生成论文摘要