Personalized Embedding-based e-Commerce Recommendations at eBay
arxiv(2021)
摘要
Recommender systems are an essential component of e-commerce marketplaces, helping consumers navigate massive amounts of inventory and find what they need or love. In this paper, we present an approach for generating personalized item recommendations in an e-commerce marketplace by learning to embed items and users in the same vector space. In order to alleviate the considerable cold-start problem present in large marketplaces, item and user embeddings are computed using content features and multi-modal onsite user activity respectively. Data ablation is incorporated into the offline model training process to improve the robustness of the production system. In offline evaluation using a dataset collected from eBay traffic, our approach was able to improve the Recall@k metric over the Recently-Viewed-Item (RVI) method. This approach to generating personalized recommendations has been launched to serve production traffic, and the corresponding scalable engineering architecture is also presented. Initial A/B test results show that compared to the current personalized recommendation module in production, the proposed method increases the surface rate by $\sim$6\% to generate recommendations for 90\% of listing page impressions.
更多查看译文
关键词
recommendations,embedding-based,e-commerce
AI 理解论文
溯源树
样例
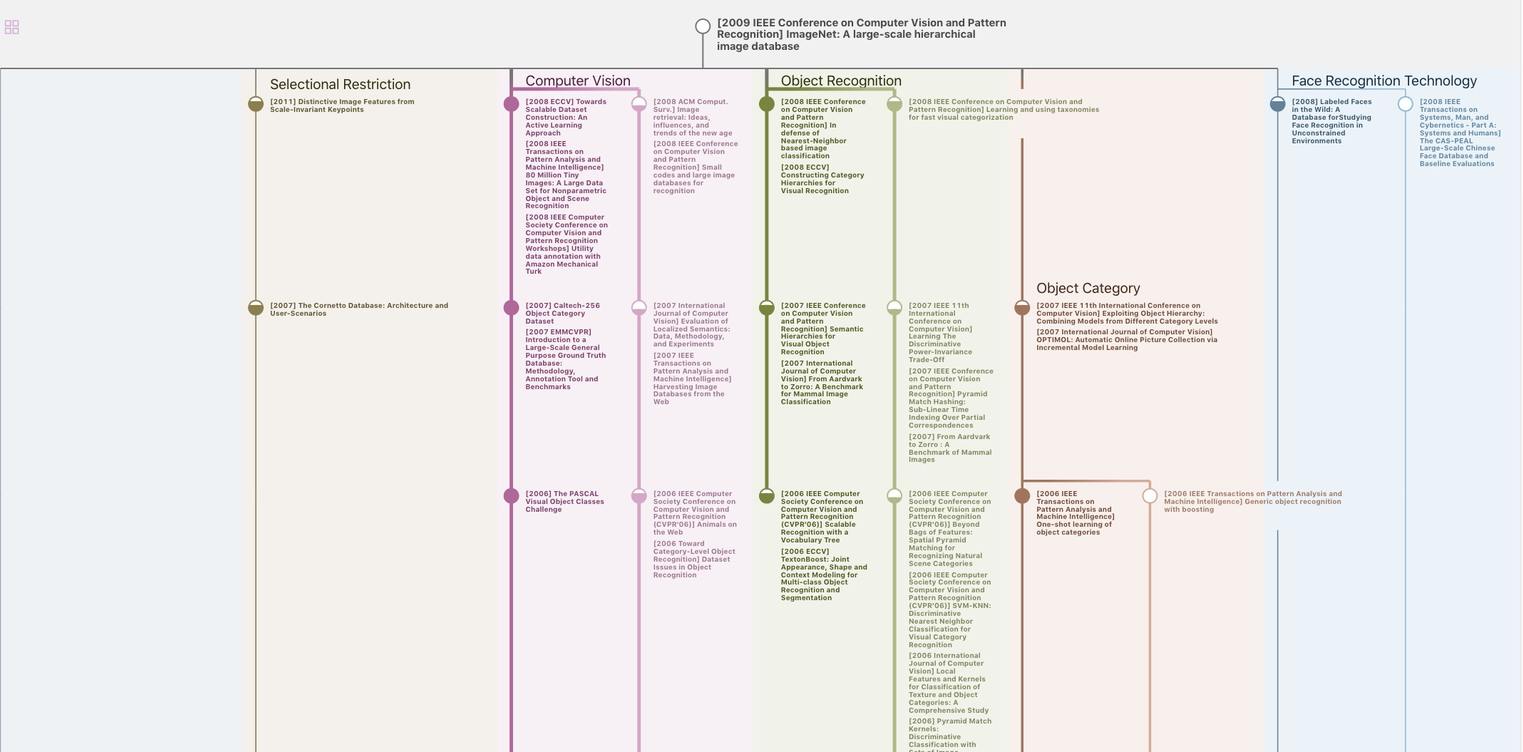
生成溯源树,研究论文发展脉络
Chat Paper
正在生成论文摘要