Statistical Inference for Polyak-Ruppert Averaged Zeroth-order Stochastic Gradient Algorithm
CoRR(2021)
摘要
As machine learning models are deployed in critical applications, it becomes important to not just provide point estimators of the model parameters (or subsequent predictions), but also quantify the uncertainty associated with estimating the model parameters via confidence sets. In the last decade, estimating or training in several machine learning models has become synonymous with running stochastic gradient algorithms. However, computing the stochastic gradients in several settings is highly expensive or even impossible at times. An important question which has thus far not been addressed sufficiently in the statistical machine learning literature is that of equipping zeroth-order stochastic gradient algorithms with practical yet rigorous inferential capabilities. Towards this, in this work, we first establish a central limit theorem for Polyak-Ruppert averaged stochastic gradient algorithm in the zeroth-order setting. We then provide online estimators of the asymptotic covariance matrix appearing in the central limit theorem, thereby providing a practical procedure for constructing asymptotically valid confidence sets (or intervals) for parameter estimation (or prediction) in the zeroth-order setting.
更多查看译文
AI 理解论文
溯源树
样例
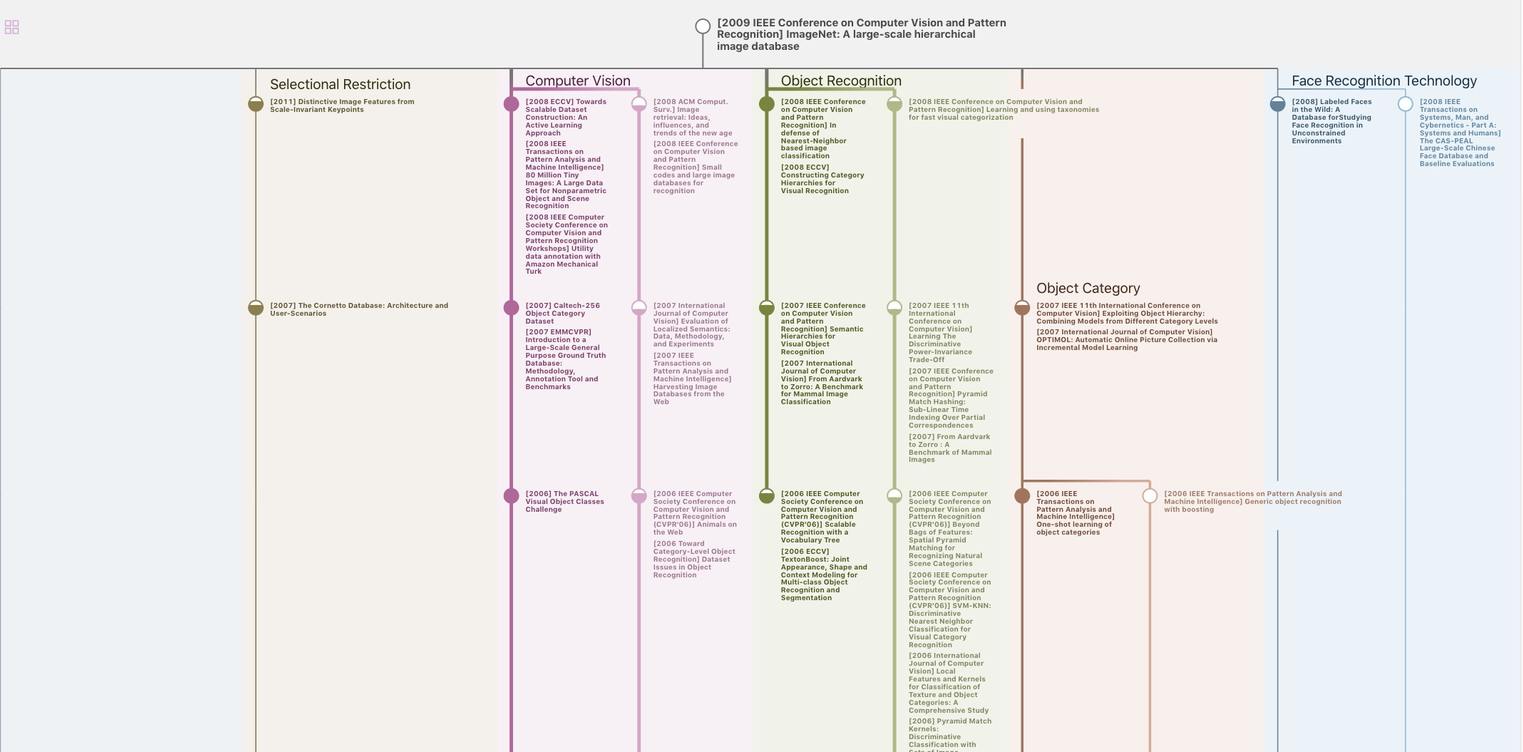
生成溯源树,研究论文发展脉络
Chat Paper
正在生成论文摘要