Efficient Techniques for Training the Memristor-based Spiking Neural Networks Targeting Better Speed, Energy and Lifetime
ASPDAC(2021)
摘要
ABSTRACTSpeed and energy consumption are two important metrics in designing spiking neural networks (SNNs). The inference process of current SNNs is terminated after a preset number of time steps for all images, which leads to a waste of time and spikes. We can terminate the inference process after proper number of time steps for each image. Besides, normalization method also influences the time and spikes of SNNs. In this work, we first use reinforcement learning algorithm to develop an efficient termination strategy which can help find the right number of time steps for each image. Then we propose a model tuning technique for memristor-based crossbar circuit to optimize the weight and bias of a given SNN. Experimental results show that the proposed techniques can reduce about 58.7% crossbar energy consumption and over 62.5% time consumption and double the drift lifetime of memristor-based SNN.
更多查看译文
关键词
Memristor, Spiking neural network, Drift, Reinforcement learning, Model tuning
AI 理解论文
溯源树
样例
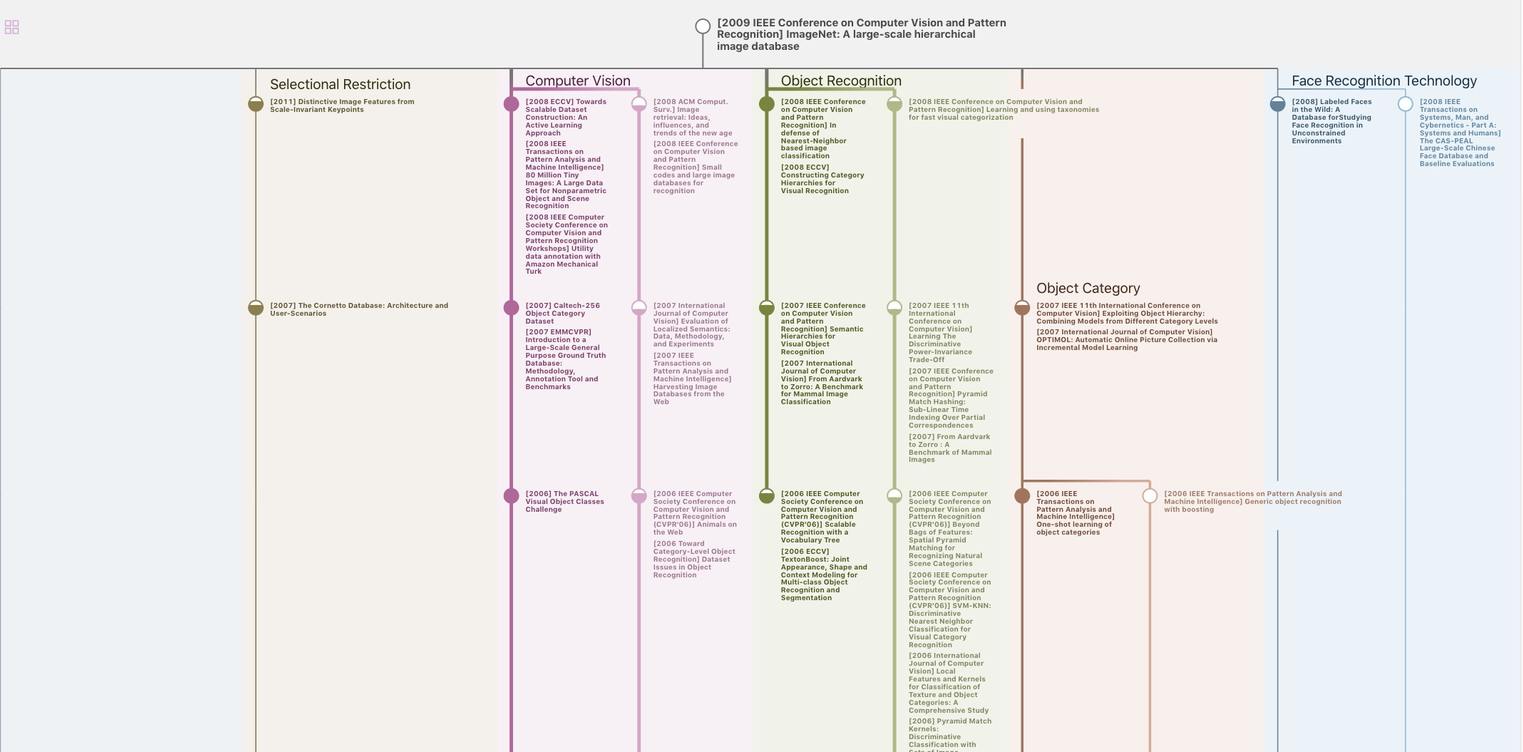
生成溯源树,研究论文发展脉络
Chat Paper
正在生成论文摘要