Sparsification via Compressed Sensing for Automatic Speech Recognition
2021 IEEE INTERNATIONAL CONFERENCE ON ACOUSTICS, SPEECH AND SIGNAL PROCESSING (ICASSP 2021)(2021)
摘要
In order to achieve high accuracy for machine learning (ML) applications, it is essential to employ models with a large number of parameters. Certain applications, such as Automatic Speech Recognition (ASR), however, require real-time interactions with users, hence compelling the model to have as low latency as possible. Deploying large scale ML applications thus necessitates model quantization and compression, especially when running ML models on resource constrained devices. For example, by forcing some of the model weight values into zero, it is possible to apply zero-weight compression, which reduces both the model size and model reading time from the memory. In the literature, such methods are referred to as sparse pruning. The fundamental questions are when and which weights should be forced to zero, i.e. be pruned. In this work, we propose a compressed sensing based pruning (CSP) approach to effectively address those questions. By reformulating sparse pruning as a sparsity inducing and compression-error reduction dual problem, we introduce the classic compressed sensing process into the ML model training process. Using ASR task as an example, we show that CSP consistently outperforms existing approaches in the literature.
更多查看译文
关键词
Model pruning, automatic speech recognition (ASR), sparsity, Recurrent Neural Network Transducer (RNN-T), compressed sensing
AI 理解论文
溯源树
样例
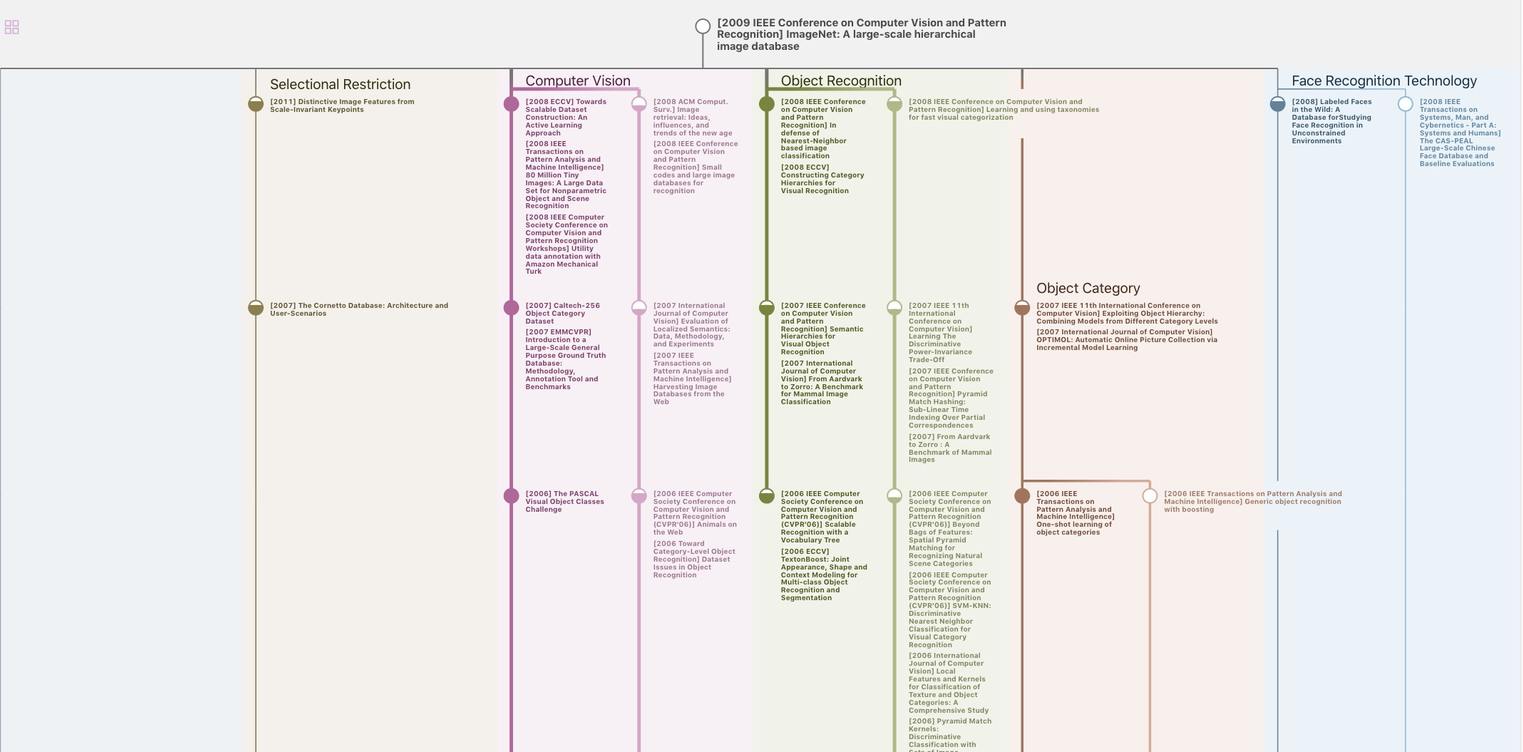
生成溯源树,研究论文发展脉络
Chat Paper
正在生成论文摘要