RL-Scope: Cross-Stack Profiling for Deep Reinforcement Learning Workloads
arxiv(2021)
摘要
RL has made groundbreaking advancements in robotic, datacenter managements and other applications. Unfortunately, system-level bottlenecks in RL workloads are poorly understood; we observe fundamental structural differences in RL workloads that make them inherently less GPU-bound than supervised learning (SL) including gathering training data in simulation, high-level code that frequently transitions to ML backends, and smaller neural networks. To explain where training time is spent in RL workloads, we propose RL-Scope, a cross-stack profiler that scopes low-level CPU/GPU resource usage to high-level algorithmic operations, and provides accurate insights by correcting for profiling overhead. We demonstrate RL-Scope's utility through in-depth case studies. First, we compare RL frameworks to quantify the effects of fundamental design choices behind ML backends. Next, we survey how training bottlenecks change as we consider different simulators and RL algorithms. Finally, we profile a scale-up workload and demonstrate that GPU utilization metrics reported by commonly-used tools dramatically inflate GPU usage, whereas RL-Scope reports true GPU-bound time. RL-Scope is an open-source tool available at https://github.com/UofT-EcoSystem/rlscope .
更多查看译文
关键词
profiling,reinforcement learning,rl-scope,cross-stack
AI 理解论文
溯源树
样例
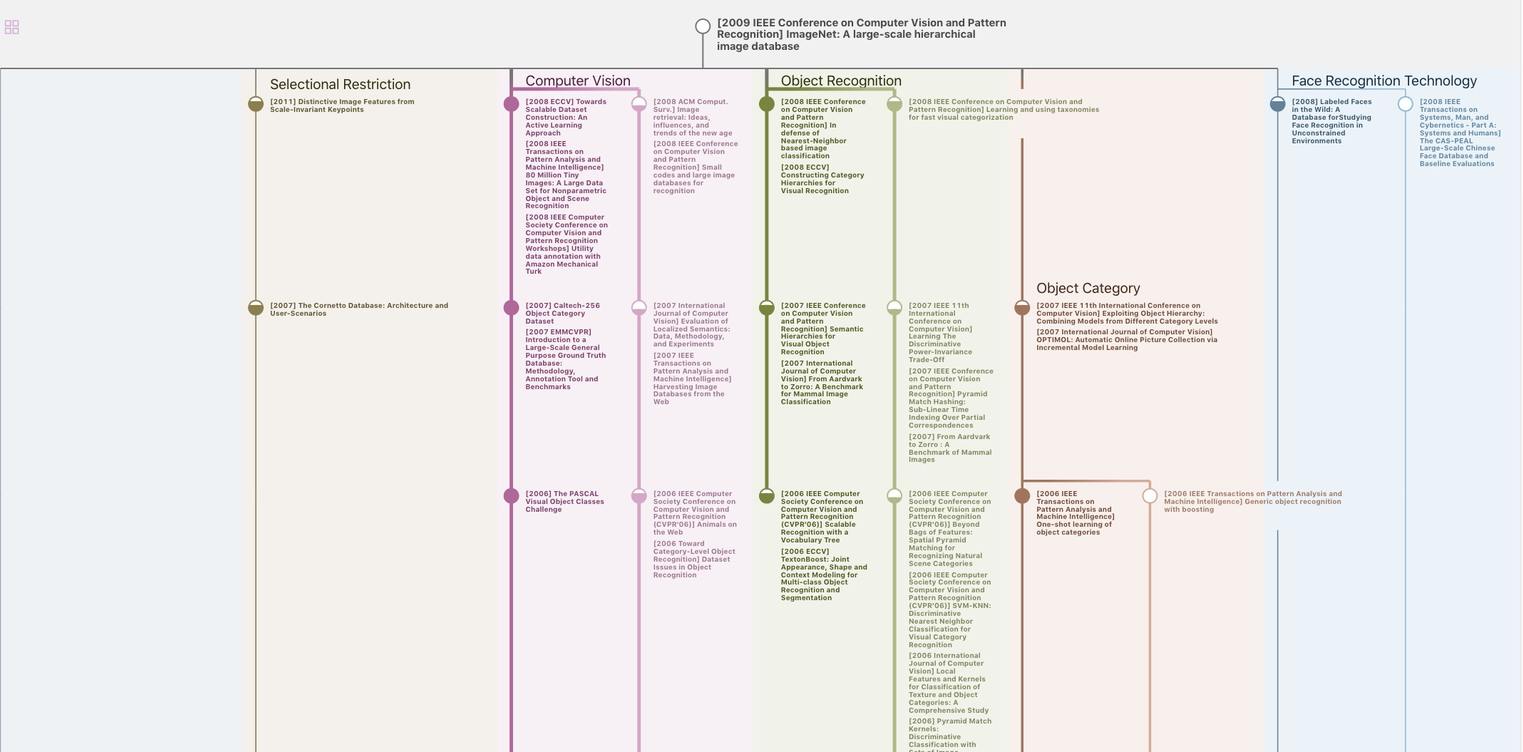
生成溯源树,研究论文发展脉络
Chat Paper
正在生成论文摘要