Towards Hierarchical Task Decomposition using Deep Reinforcement Learning for Pick and Place Subtasks
2021 20th International Conference on Advanced Robotics (ICAR)(2021)
Abstract
Deep Reinforcement Learning (DRL) is emerging as a promising approach to generate adaptive behaviors for robotic platforms. However, a major drawback of using DRL is the data-hungry training regime that requires millions of trial and error attempts, which is impractical when running experiments on robotic systems. Learning from Demonstrations (LfD) has been introduced to solve this issue by cloning the behavior of expert demonstrations. However, LfD requires a large number of demonstrations that are difficult to be acquired since dedicated complex setups are required. To overcome these limitations, we propose a multi-subtask reinforcement learning methodology where complex pick and place tasks can be decomposed into low-level subtasks. These subtasks are parametrized as expert networks and learned via DRL methods. Trained subtasks are then combined by a high-level choreographer to accomplish the intended pick and place task considering different initial configurations. As a testbed, we use a pick and place robotic simulator to demonstrate our methodology and show that our method outperforms a benchmark methodology based on LfD in terms of sample-efficiency. We transfer the learned policy to the real robotic system and demonstrate robust grasping using various geometric-shaped objects.
MoreTranslated text
Key words
hierarchical task decomposition,place subtasks,deep reinforcement,reinforcement
learning
AI Read Science
Must-Reading Tree
Example
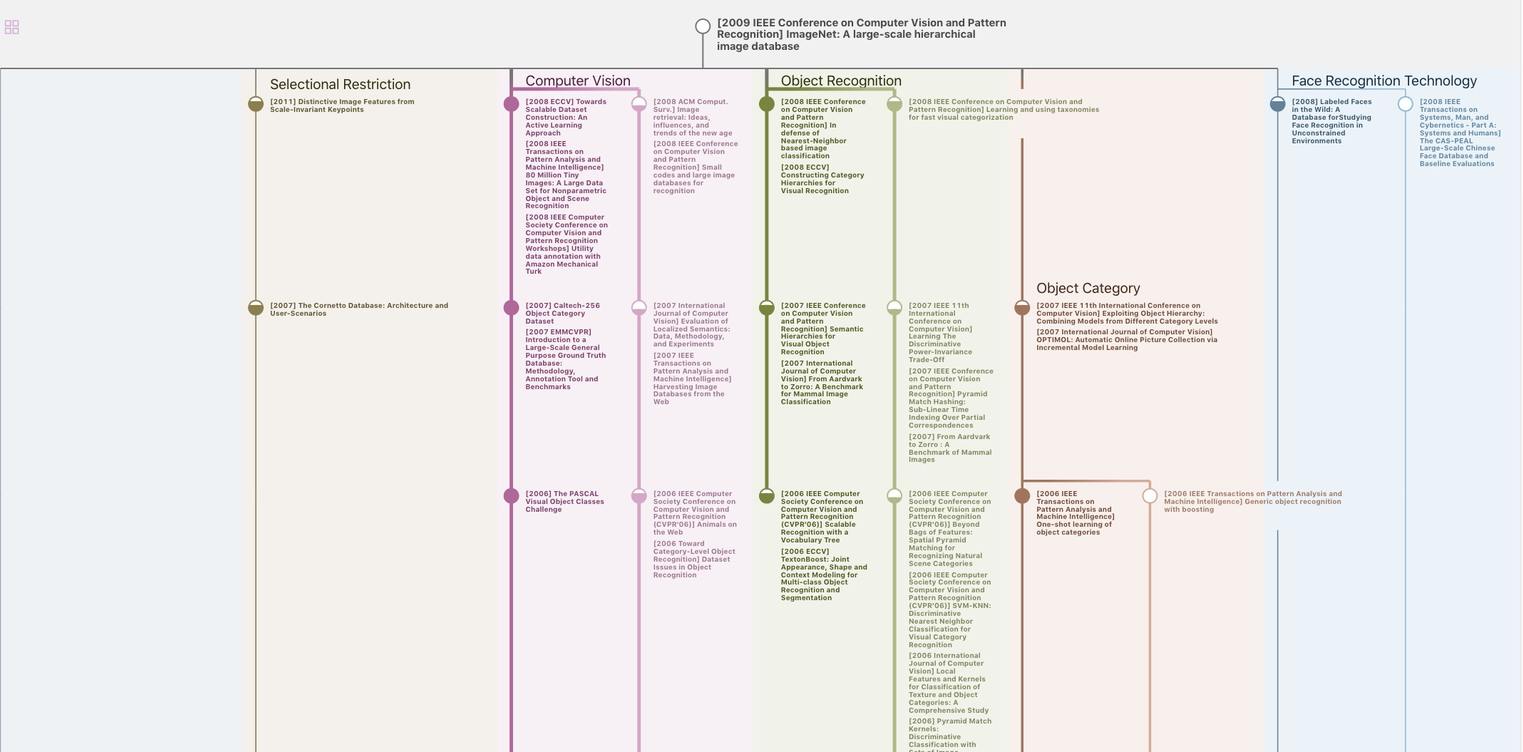
Generate MRT to find the research sequence of this paper
Chat Paper
Summary is being generated by the instructions you defined