Generalization Bounds for Noisy Iterative Algorithms Using Properties of Additive Noise Channels
JOURNAL OF MACHINE LEARNING RESEARCH(2023)
摘要
Machine learning models trained by different optimization algorithms under different data distributions can exhibit distinct generalization behaviors. In this paper, we analyze the generalization of models trained by noisy iterative algorithms. We derive distributiondependent generalization bounds by connecting noisy iterative algorithms to additive noise channels found in communication and information theory. Our generalization bounds shed light on several applications, including differentially private stochastic gradient descent (DP-SGD), federated learning, and stochastic gradient Langevin dynamics (SGLD). We demonstrate our bounds through numerical experiments, showing that they can help understand recent empirical observations of the generalization phenomena of neural networks.
更多查看译文
关键词
Information theory,algorithmic generalization bound,differential privacy,stochastic gradient Langevin dynamics,federated learning
AI 理解论文
溯源树
样例
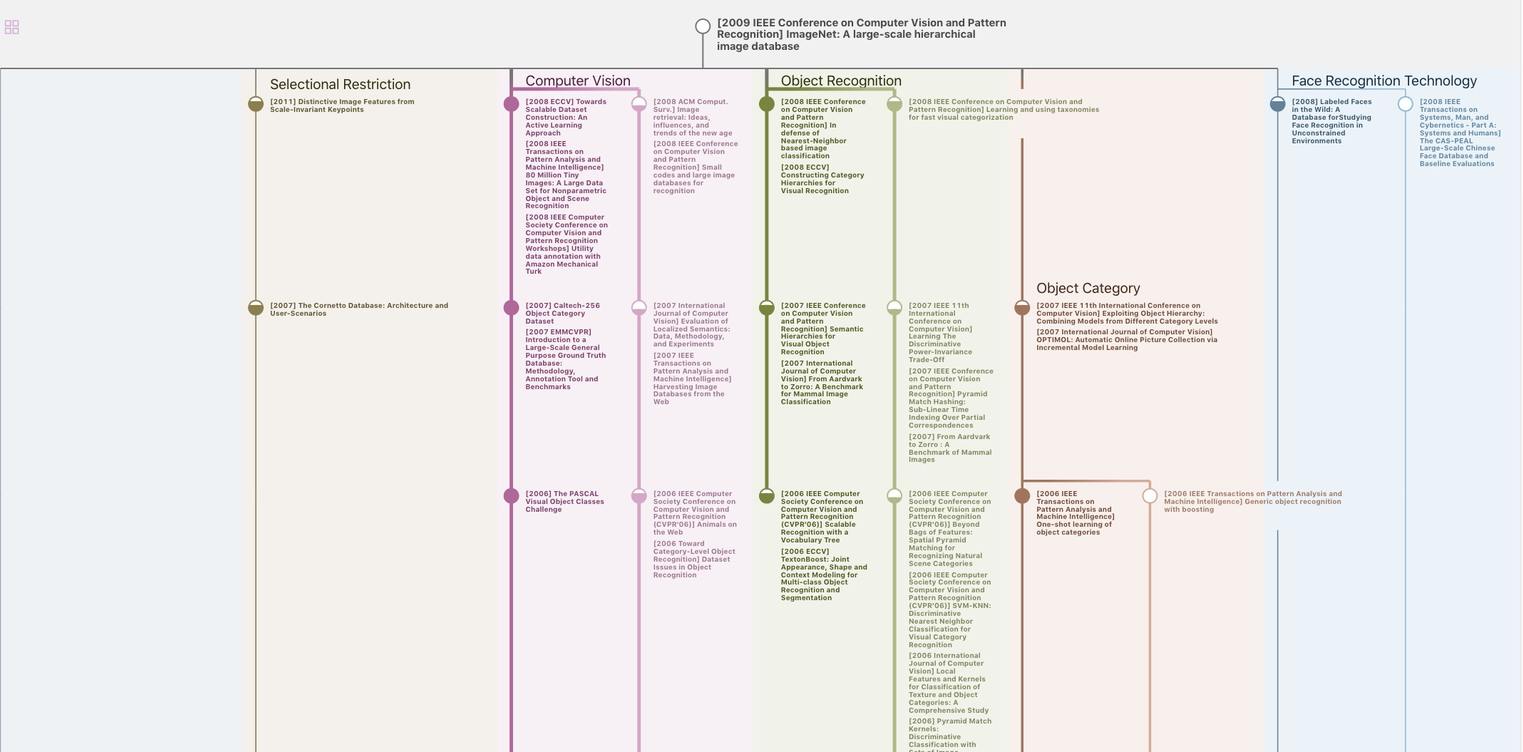
生成溯源树,研究论文发展脉络
Chat Paper
正在生成论文摘要