Accelerated gradient methods with absolute and relative noise in the gradient
arxiv(2023)
摘要
In this paper, we investigate accelerated first-order methods for smooth convex optimization problems under inexact information on the gradient of the objective. The noise in the gradient is considered to be additive with two possibilities: absolute noise bounded by a constant, and relative noise proportional to the norm of the gradient. We investigate the accumulation of the errors in the convex and strongly convex settings with the main difference with most of the previous works being that the feasible set can be unbounded. The key to the latter is to prove a bound on the trajectory of the algorithm. We also give a stopping criterion for the algorithm and consider extensions to the cases of stochastic optimization and composite nonsmooth problems.
更多查看译文
关键词
gradient methods,relative noise
AI 理解论文
溯源树
样例
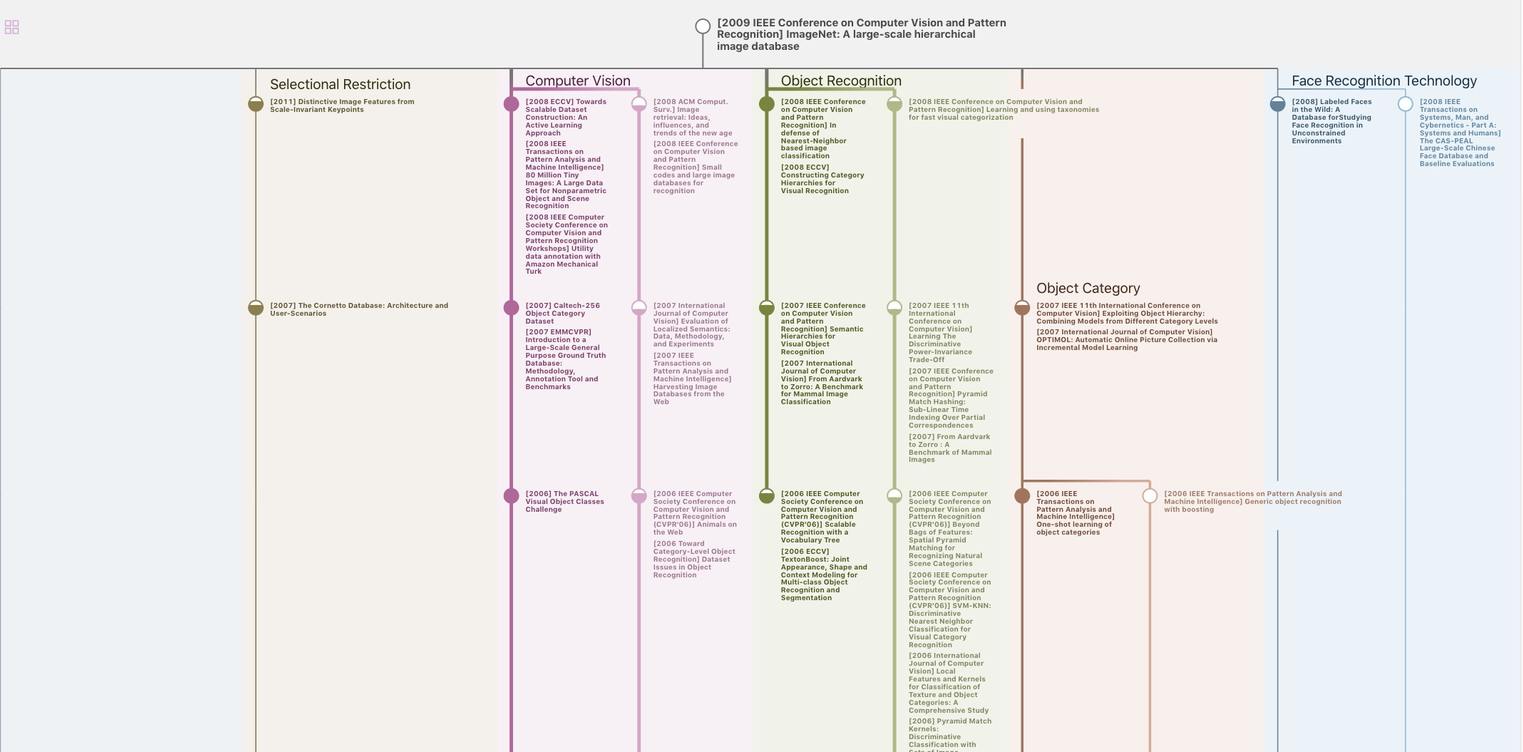
生成溯源树,研究论文发展脉络
Chat Paper
正在生成论文摘要