Single View Point Cloud Generation Via Unified 3d Prototype
THIRTY-FIFTH AAAI CONFERENCE ON ARTIFICIAL INTELLIGENCE, THIRTY-THIRD CONFERENCE ON INNOVATIVE APPLICATIONS OF ARTIFICIAL INTELLIGENCE AND THE ELEVENTH SYMPOSIUM ON EDUCATIONAL ADVANCES IN ARTIFICIAL INTELLIGENCE(2021)
摘要
As 3D point clouds become the representation of choice for multiple vision and graphics applications, such as autonomous driving, robotics, etc., the generation of them by deep neural networks has attracted increasing attention in the research community. Despite the recent success of deep learning models in classification and segmentation, synthe-sizing point clouds remains challenging, especially from a single image. State-of-the-art (SOTA) approaches can generate a point cloud from a hidden vector, however, they treat 2D and 3D features equally and disregard the rich shape information within the 3D data. In this paper, we address this problem by integrating image features with 3D prototype features. Specifically, we propose to learn a set of 3D prototype features from a real point cloud dataset and dynamically adjust them through the training These prototypes are then integrated with incoming image features to guide the point cloud generation process. Experimental results show that our proposed method outperforms SOTA methods on single image based 3D reconstruction tasks.
更多查看译文
AI 理解论文
溯源树
样例
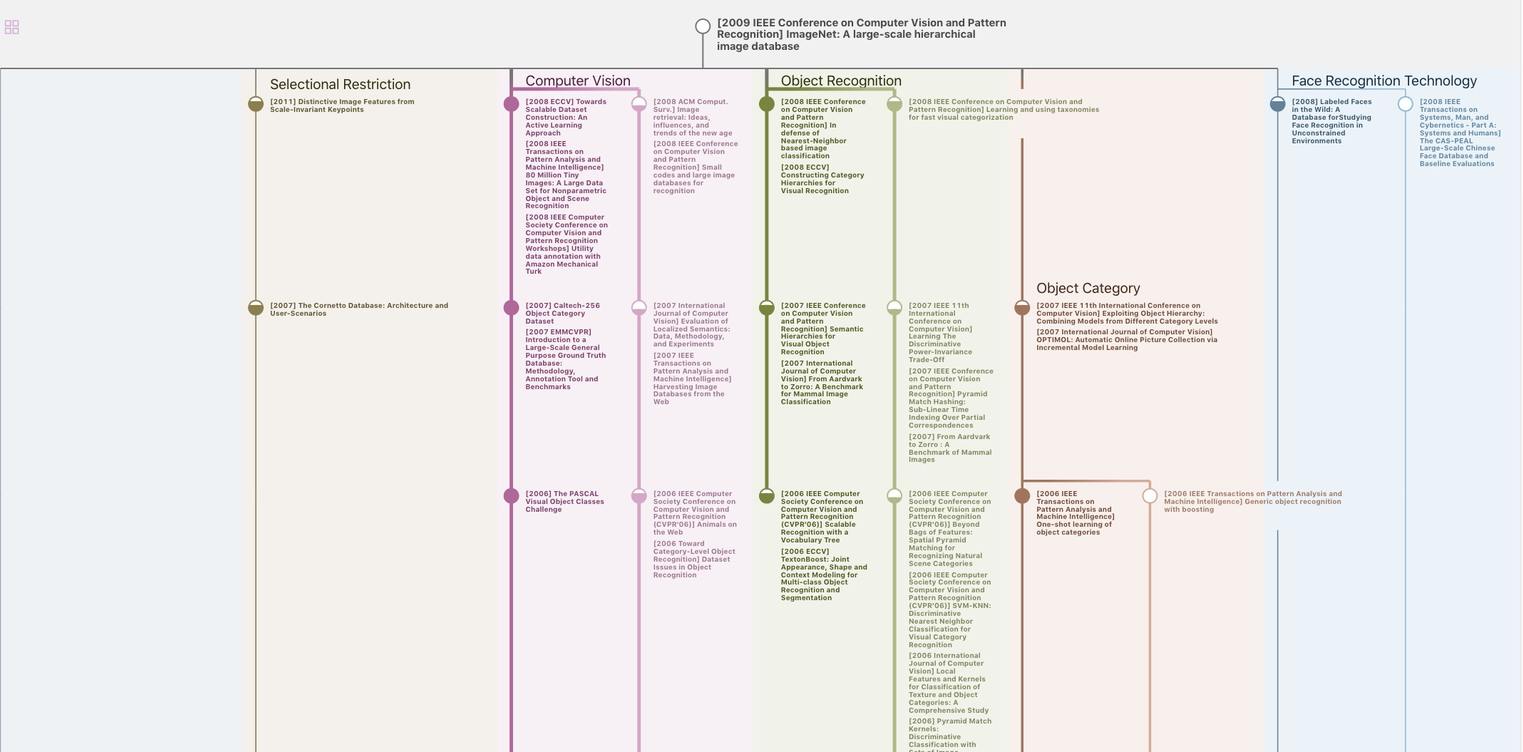
生成溯源树,研究论文发展脉络
Chat Paper
正在生成论文摘要