Empowering Adaptive Early-Exit Inference With Latency Awareness
THIRTY-FIFTH AAAI CONFERENCE ON ARTIFICIAL INTELLIGENCE, THIRTY-THIRD CONFERENCE ON INNOVATIVE APPLICATIONS OF ARTIFICIAL INTELLIGENCE AND THE ELEVENTH SYMPOSIUM ON EDUCATIONAL ADVANCES IN ARTIFICIAL INTELLIGENCE(2021)
摘要
With the capability of trading accuracy for latency on-the-fly, the technique of adaptive early-exit inference has emerged as a promising line of research to accelerate the deep learning inference. However, studies in this line of research commonly use a group of thresholds to control the accuracy-latency trade-off, where a thorough and general methodology on how to determine these thresholds has not been conducted yet, especially with regard to the common requirements of average inference latency. To address this issue and enable latency-aware adaptive early-exit inference, in the present paper, we approximately formulate the threshold determination problem of finding the accuracy-maximum threshold setting that meets a given average latency requirement, and then propose a threshold determination method to tackle our formulated non-convex problem. Theoretically, we prove that, for certain parameter settings, our method finds an approximate stationary point of the formulated problem. Empirically, on top of various models across multiple datasets (CIFAR-10, CIFAR-100, ImageNet and two time-series datasets), we show that our method can well handle the average latency requirements, and consistently finds good threshold settings in negligible time.
更多查看译文
关键词
adaptive,awareness,early-exit
AI 理解论文
溯源树
样例
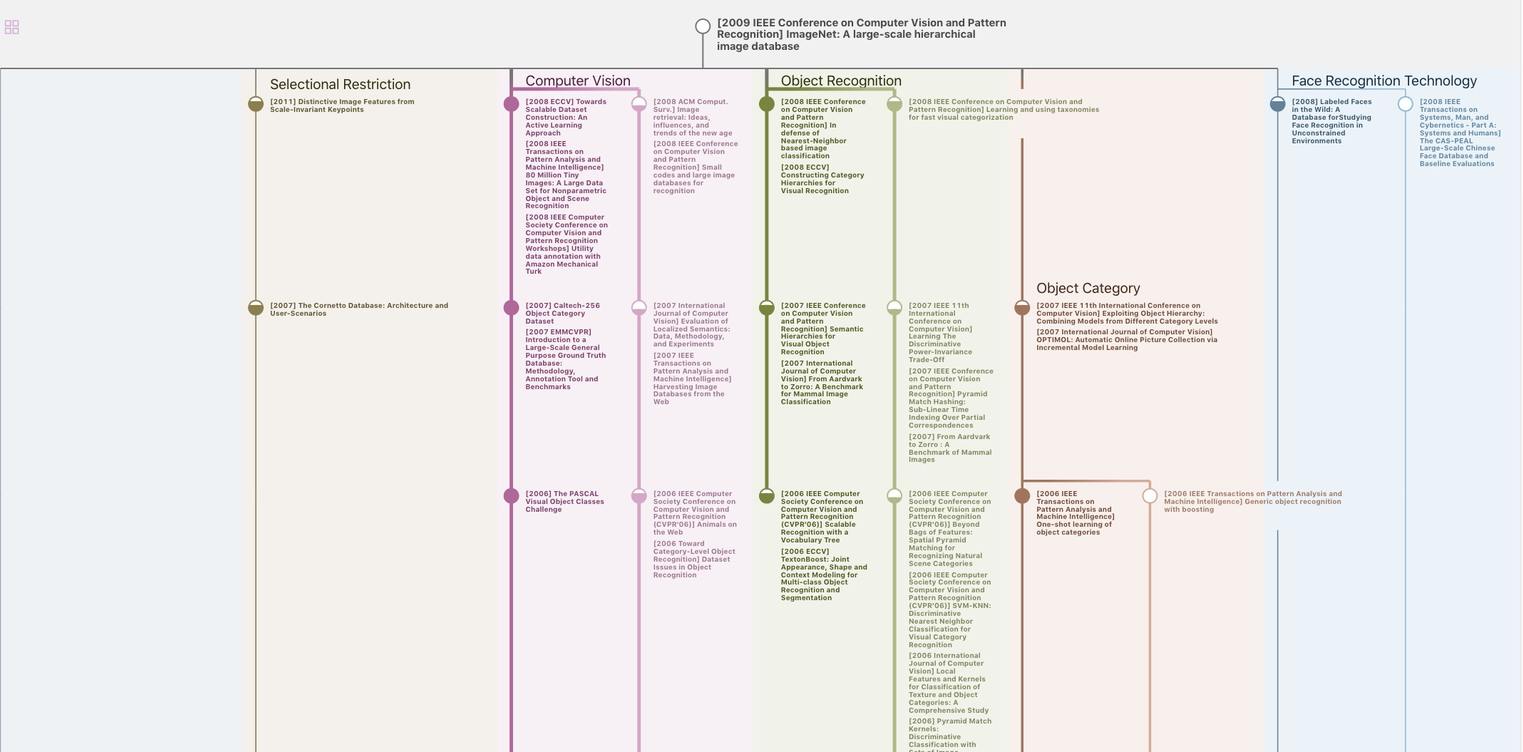
生成溯源树,研究论文发展脉络
Chat Paper
正在生成论文摘要